Impact of geography on the importance of parameters in infectious disease models
CoRR(2023)
摘要
Agent-based models are widely used to predict infectious disease spread. For these predictions, one needs to understand how each input parameter affects the result. Here, some parameters may affect the sensitivities of others, requiring the analysis of higher order coefficients through e.g. Sobol sensitivity analysis. The geographical structures of real-world regions are distinct in that they are difficult to reduce to single parameter values, making a unified sensitivity analysis intractable. Yet analyzing the importance of geographical structure on the sensitivity of other input parameters is important because a strong effect would justify the use of models with real-world geographical representations, as opposed to stylized ones. Here we perform a grouped Sobol's sensitivity analysis on COVID-19 spread simulations across a set of three diverse real-world geographical representations. We study the differences in both results and the sensitivity of non-geographical parameters across these geographies. By comparing Sobol indices of parameters across geographies, we find evidence that infection rate could have more sensitivity in regions where the population is segregated, while parameters like recovery period of mild cases are more sensitive in regions with mixed populations. We also show how geographical structure affects parameter sensitivity changes over time.
更多查看译文
关键词
geography,disease,models
AI 理解论文
溯源树
样例
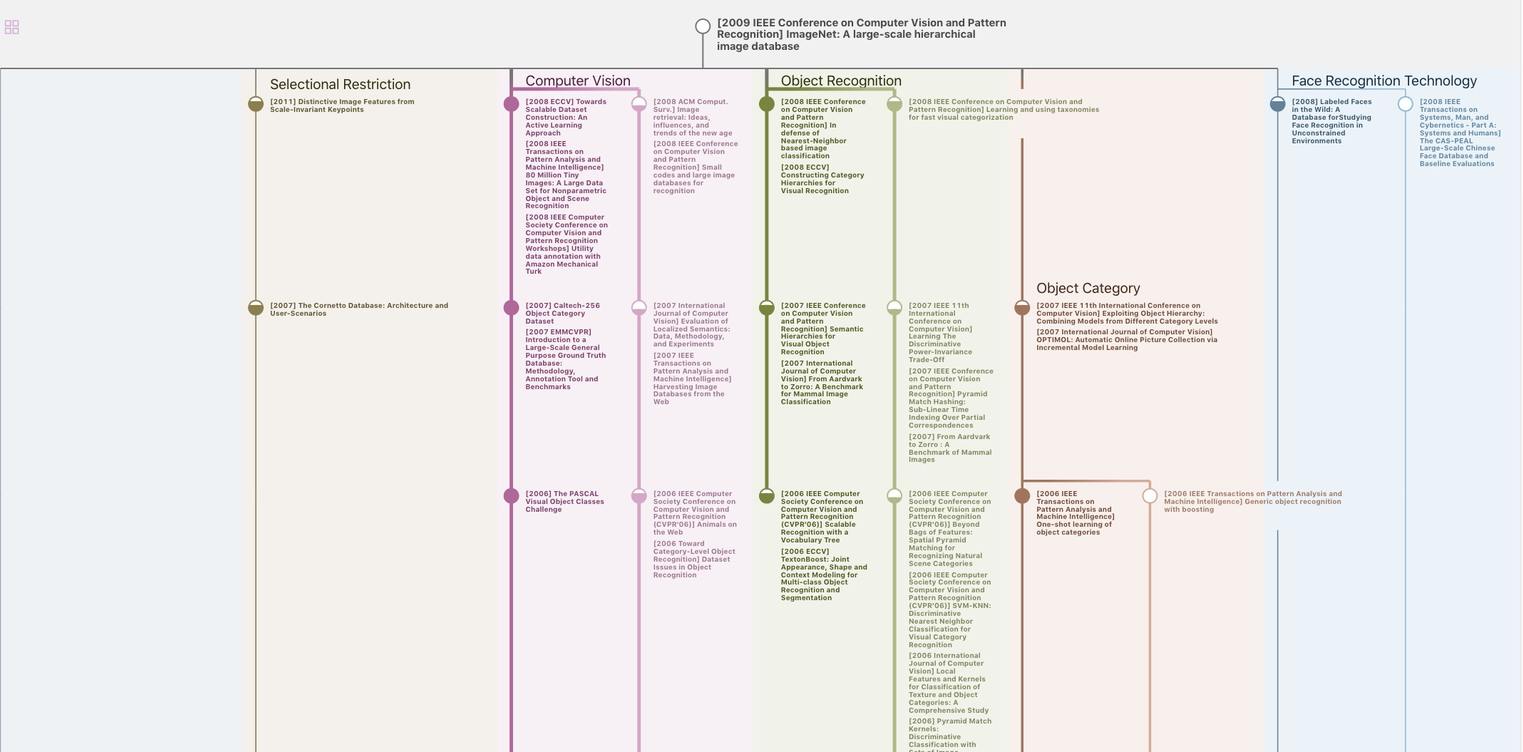
生成溯源树,研究论文发展脉络
Chat Paper
正在生成论文摘要