Deep Learning and Latent Variables in Nonuniform Antenna Array Processing for Direction of Arrival
2023 XXXVth General Assembly and Scientific Symposium of the International Union of Radio Science (URSI GASS)(2023)
AI 理解论文
溯源树
样例
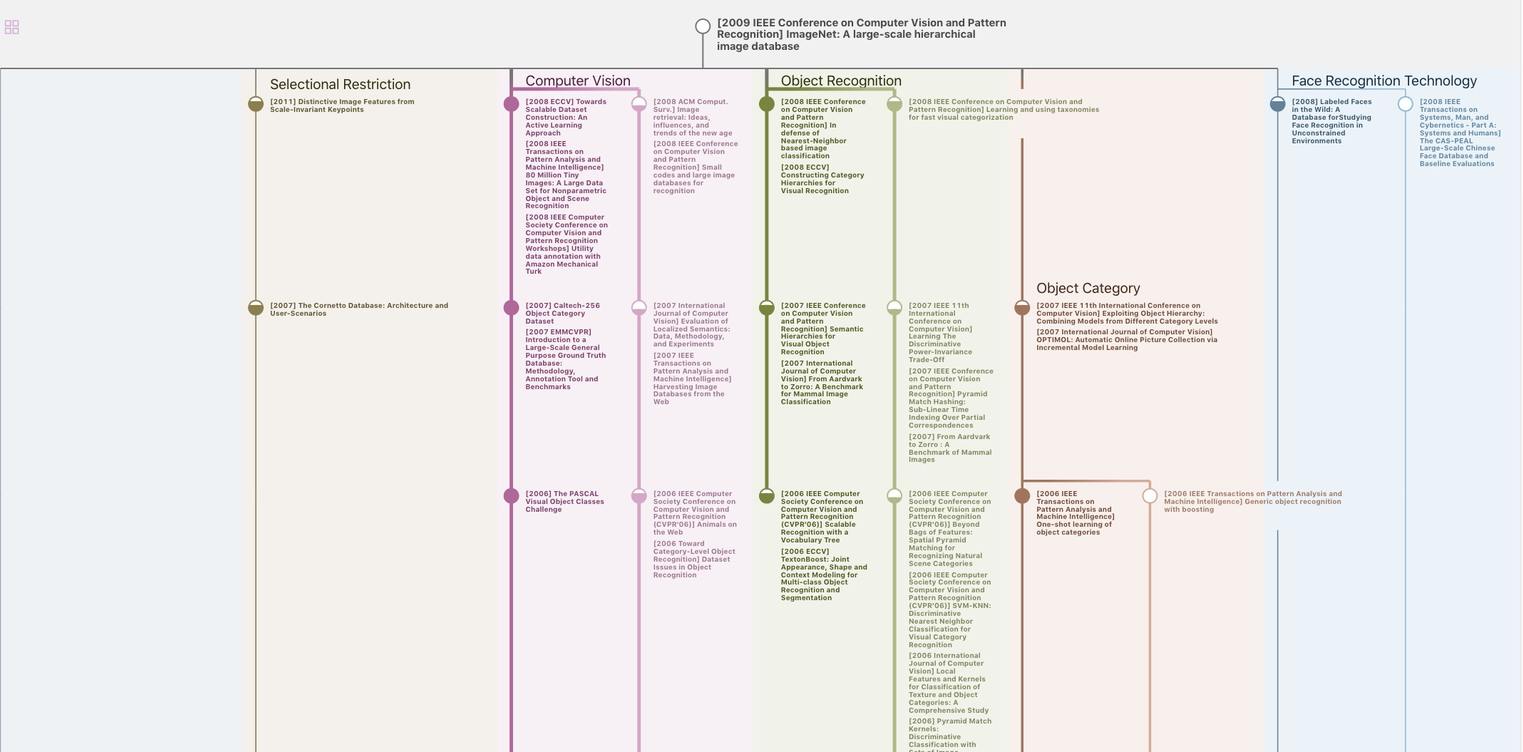
生成溯源树,研究论文发展脉络
Chat Paper
正在生成论文摘要