Incentive-Based Federated Learning for Digital-Twin-Driven Industrial Mobile Crowdsensing
IEEE Internet of Things Journal(2023)
摘要
Mobile crowdsensing has empowered the Industrial Internet of Things (IIoT) in many ways, such as vehicle-aided traffic flow scheduling, drone-aided visual inspections, etc. However, dynamic perception and cooperative decision making among these heterogeneous and resource-constrained mobile clients in IIoT remains a big challenge. In this article, we propose an incentive-based federated learning scheme for digital twin (DT)-driven industrial mobile crowdsensing. Specifically, we first design a DT-driven industrial mobile crowdsensing architecture to achieve dynamic perception of the complex IIoT environment, among heterogeneous and resource-constrained mobile clients. Second, we develop a novel incentive-based federated learning framework incorporated with a contract-based reputation mechanism and a Stackelberg-based interclient incentive mechanism, to optimize the model accuracy. Third, we devise a knowledge distillation algorithm for the federated learning framework, to address the heterogeneity of nonindependent and identically distributed (Non-IID) data. Extensive experiments on both MNIST/FEMNIST and CIFAR10/100 data sets demonstrate the outperformance of our proposed scheme, in terms of model accuracy, incentive fairness, and data compatibility, compared to state-of-the-art studies.
更多查看译文
关键词
Digital twin (DT),federated learning,incentive mechanism,Industrial Internet of Things (IIoT),knowledge distillation,mobile crowdsensing
AI 理解论文
溯源树
样例
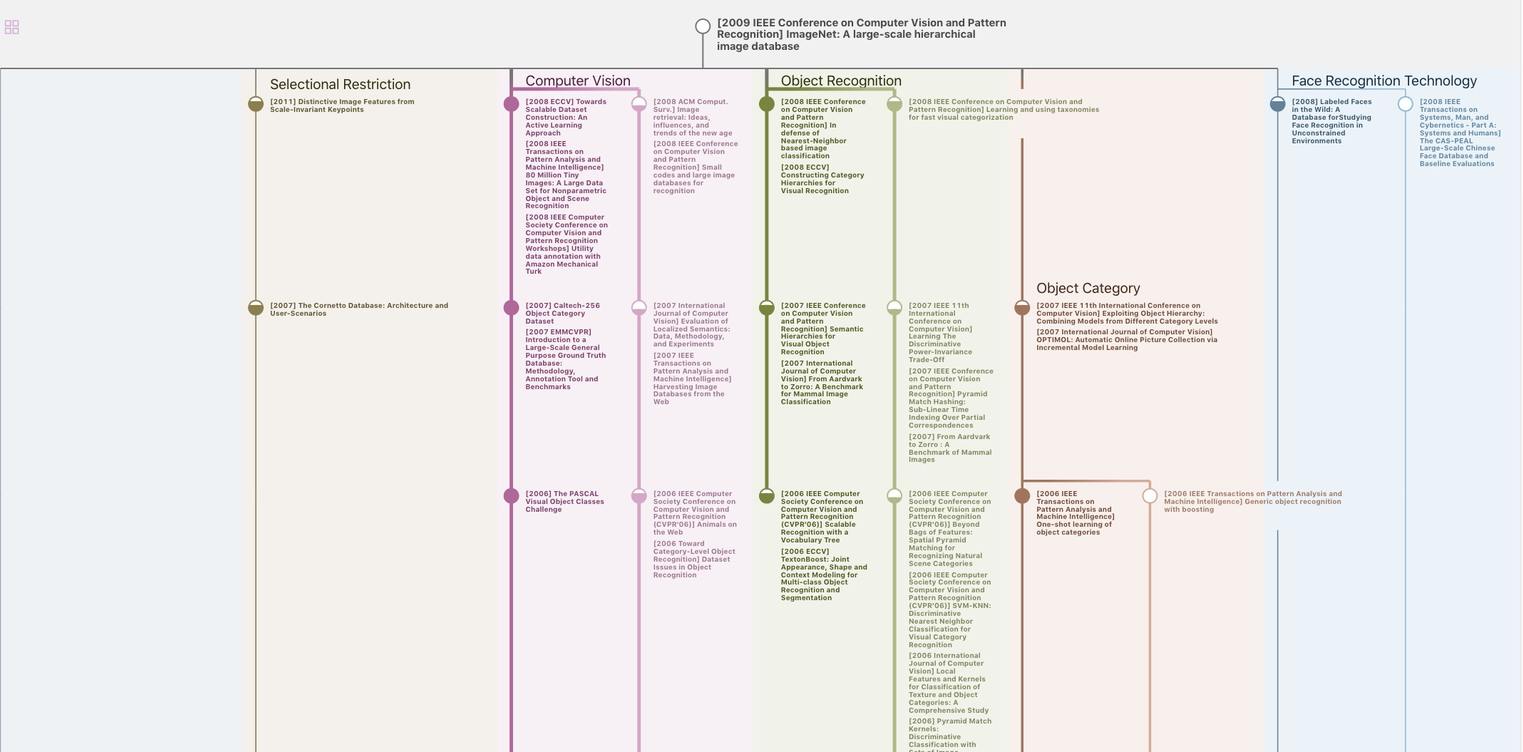
生成溯源树,研究论文发展脉络
Chat Paper
正在生成论文摘要