The Importance of Expert Knowledge for Automatic Modulation Open Set Recognition
IEEE Transactions on Pattern Analysis and Machine Intelligence(2023)
摘要
Automatic modulation classification (AMC) is an important technology for the monitoring, management, and control of communication systems. In recent years, machine learning approaches are becoming popular to improve the effectiveness of AMC for radio signals. However, the automatic modulation open-set recognition (AMOSR) scheme that aims to identify the known modulation types and recognize the unknown modulation signals is not well studied. Therefore, in this paper, we propose a novel multi-modal marginal prototype framework for radio frequency (RF) signals (
MMPRF
) to improve AMOSR performance. First,
MMPRF
addresses the problem of simultaneous recognition of closed and open sets by partitioning the feature space in the way of
one versus other
and marginal restrictions. Second, we exploit the wireless signal domain knowledge to extract a series of signal-related features to enhance the AMOSR capability. In addition, we propose a GAN-based unknown sample generation strategy to allow the model to understand the unknown world. Finally, we conduct extensive experiments on several publicly available radio modulation data, and experimental results show that our proposed
MMPRF
outperforms the state-of-the-art AMOSR methods.
更多查看译文
关键词
Automatic modulation classification,machine learning,marginal prototype,open-set recognition,radio signals
AI 理解论文
溯源树
样例
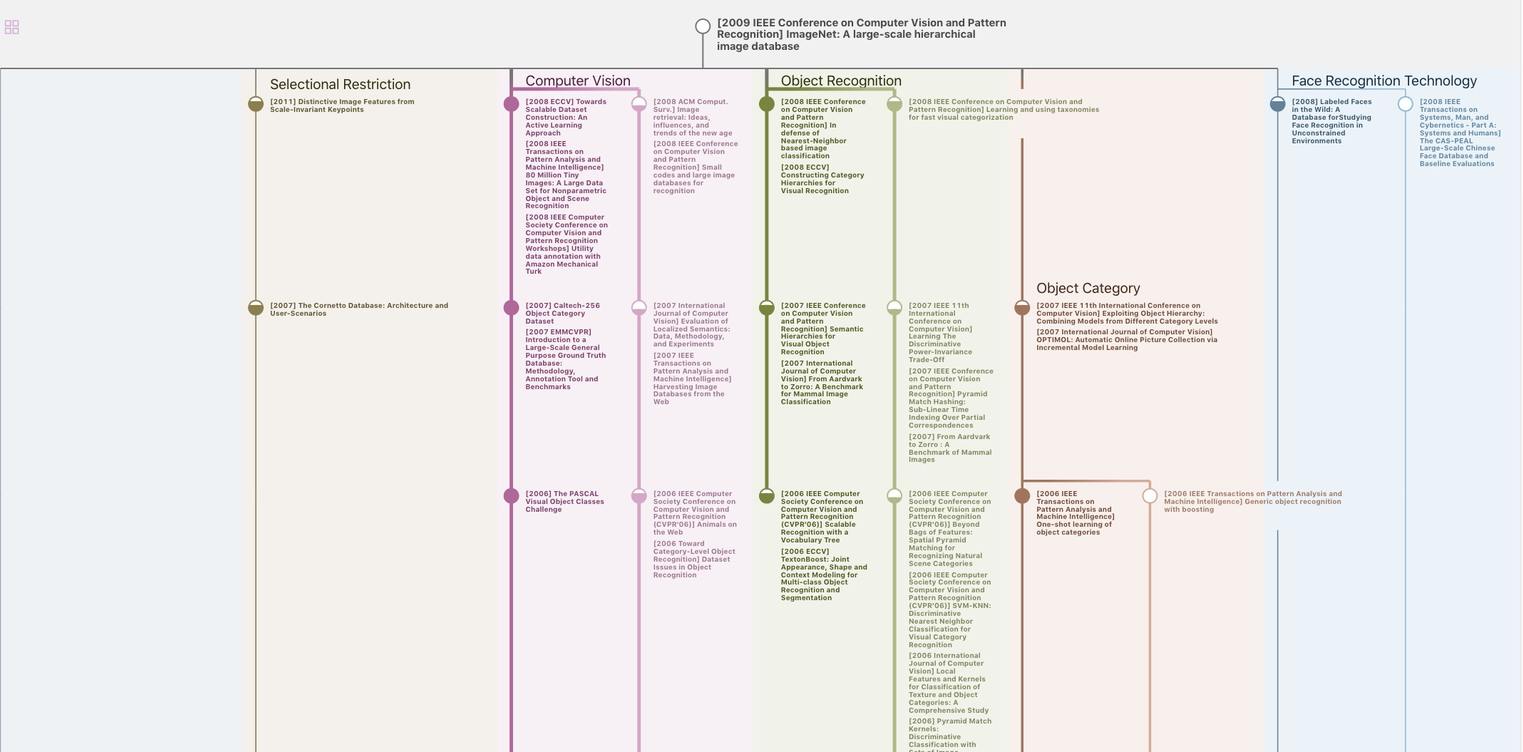
生成溯源树,研究论文发展脉络
Chat Paper
正在生成论文摘要