An Energy-Based Prior for Generative Saliency
IEEE Transactions on Pattern Analysis and Machine Intelligence(2023)
摘要
We propose a novel generative saliency prediction framework that adopts an informative energy-based model as a prior distribution. The energy-based prior model is defined on the latent space of a saliency generator network that generates the saliency map based on a continuous latent variables and an observed image. Both the parameters of saliency generator and the energy-based prior are jointly trained via Markov chain Monte Carlo-based maximum likelihood estimation, in which the sampling from the intractable posterior and prior distributions of the latent variables are performed by Langevin dynamics. With the generative saliency model, we can obtain a pixel-wise uncertainty map from an image, indicating model confidence in the saliency prediction. Different from existing generative models, which define the prior distribution of the latent variables as a simple isotropic Gaussian distribution, our model uses an energy-based informative prior which can be more expressive in capturing the latent space of the data. With the informative energy-based prior, we extend the Gaussian distribution assumption of generative models to achieve a more representative distribution of the latent space, leading to more reliable uncertainty estimation. We apply the proposed frameworks to both RGB and RGB-D salient object detection tasks with both transformer and convolutional neural network backbones. We further propose an adversarial learning algorithm and a variational inference algorithm as alternatives to train the proposed generative framework. Experimental results show that our generative saliency model with an energy-based prior can achieve not only accurate saliency predictions but also reliable uncertainty maps that are consistent with human perception.
更多查看译文
关键词
Adversarial learning,energy-based models,generative models,Langevin dynamics,salient object detection,variational inference,vision transformer
AI 理解论文
溯源树
样例
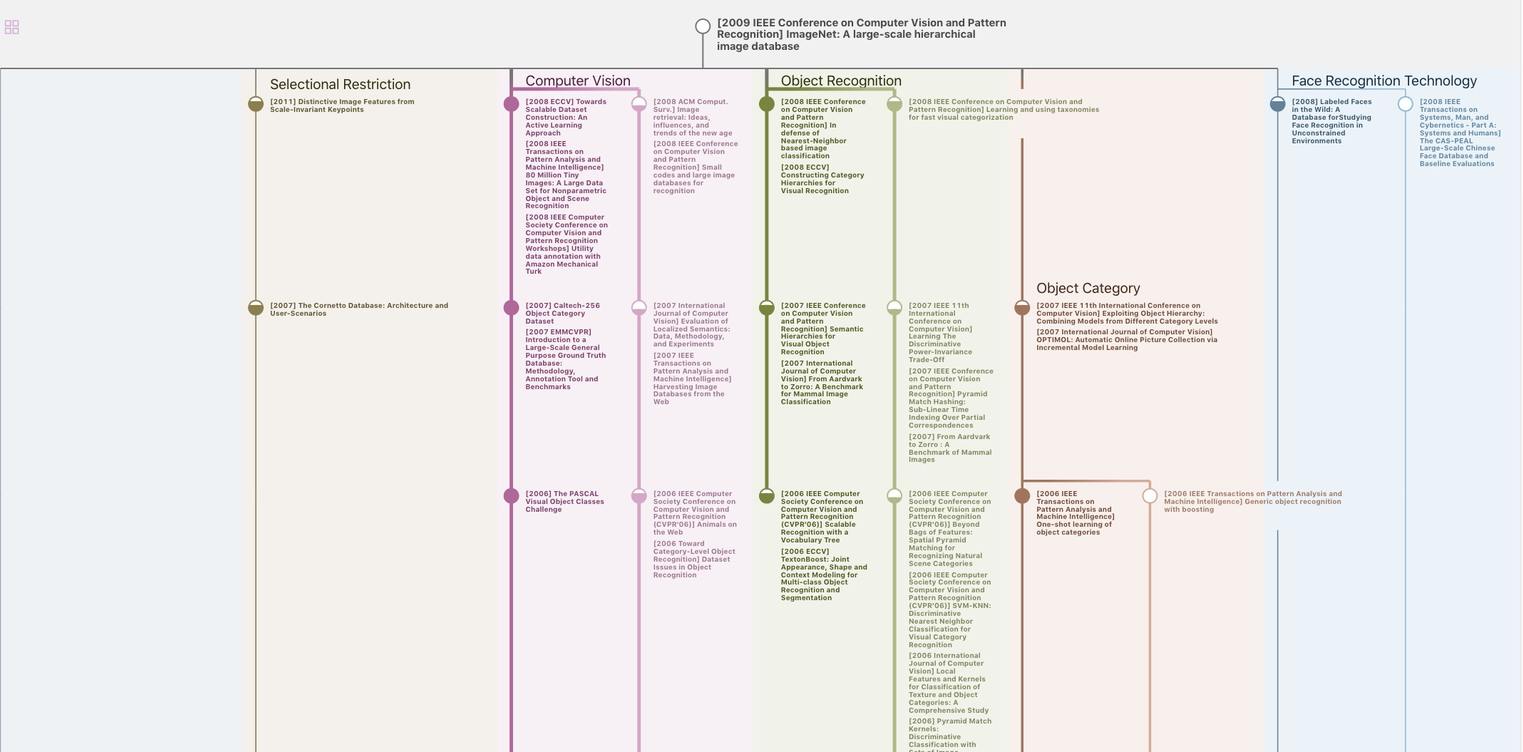
生成溯源树,研究论文发展脉络
Chat Paper
正在生成论文摘要