Generalized Convergence Analysis of Tsetlin Machines: A Probabilistic Approach to Concept Learning
CoRR(2023)
摘要
Tsetlin Machines (TMs) have garnered increasing interest for their ability to learn concepts via propositional formulas and their proven efficiency across various application domains. Despite this, the convergence proof for the TMs, particularly for the AND operator (\emph{conjunction} of literals), in the generalized case (inputs greater than two bits) remains an open problem. This paper aims to fill this gap by presenting a comprehensive convergence analysis of Tsetlin automaton-based Machine Learning algorithms. We introduce a novel framework, referred to as Probabilistic Concept Learning (PCL), which simplifies the TM structure while incorporating dedicated feedback mechanisms and dedicated inclusion/exclusion probabilities for literals. Given $n$ features, PCL aims to learn a set of conjunction clauses $C_i$ each associated with a distinct inclusion probability $p_i$. Most importantly, we establish a theoretical proof confirming that, for any clause $C_k$, PCL converges to a conjunction of literals when $0.5更多
查看译文
关键词
tsetlin machines,generalized convergence analysis,learning,concept
AI 理解论文
溯源树
样例
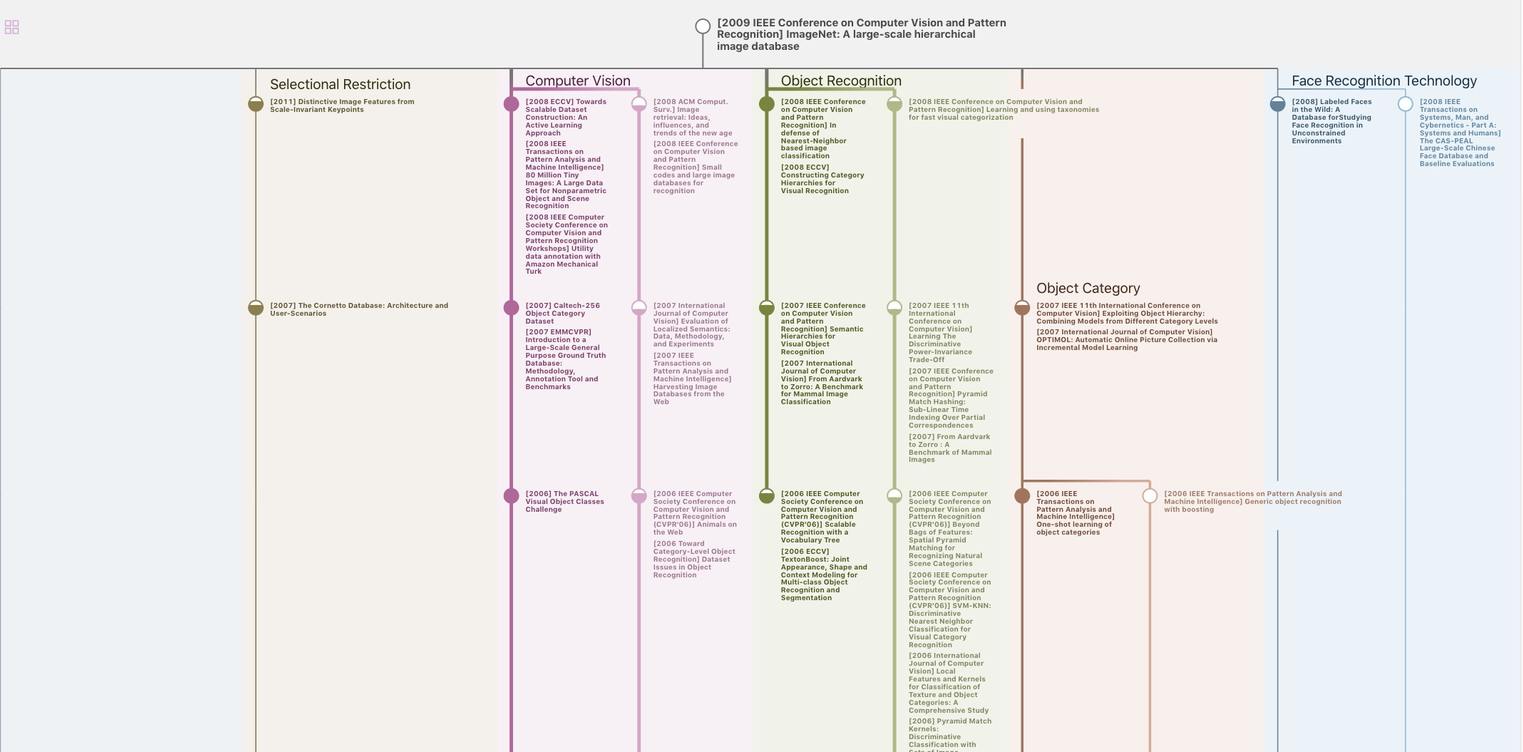
生成溯源树,研究论文发展脉络
Chat Paper
正在生成论文摘要