A simple connection from loss flatness to compressed representations in neural networks
arXiv (Cornell University)(2023)
摘要
The generalization capacity of deep neural networks has been studied in a
variety of ways, including at least two distinct categories of approaches: one
based on the shape of the loss landscape in parameter space, and the other
based on the structure of the representation manifold in feature space (that
is, in the space of unit activities). Although these two approaches are
related, they are rarely studied together explicitly. Here, we present an
analysis that bridges this gap. We show that in the final phase of learning in
deep neural networks, the compression of the manifold of neural representations
correlates with the flatness of the loss around the minima explored by SGD.
This correlation is predicted by a relatively simple mathematical relationship:
a flatter loss corresponds to a lower upper bound on the compression metrics of
neural representations. Our work builds upon the linear stability insight by Ma
and Ying, deriving inequalities between various compression metrics and
quantities involving sharpness. Empirically, our derived inequality predicts a
consistently positive correlation between representation compression and loss
sharpness in multiple experimental settings. Overall, we advance a dual
perspective on generalization in neural networks in both parameter and feature
space.
更多查看译文
关键词
loss flatness,representations,neural networks
AI 理解论文
溯源树
样例
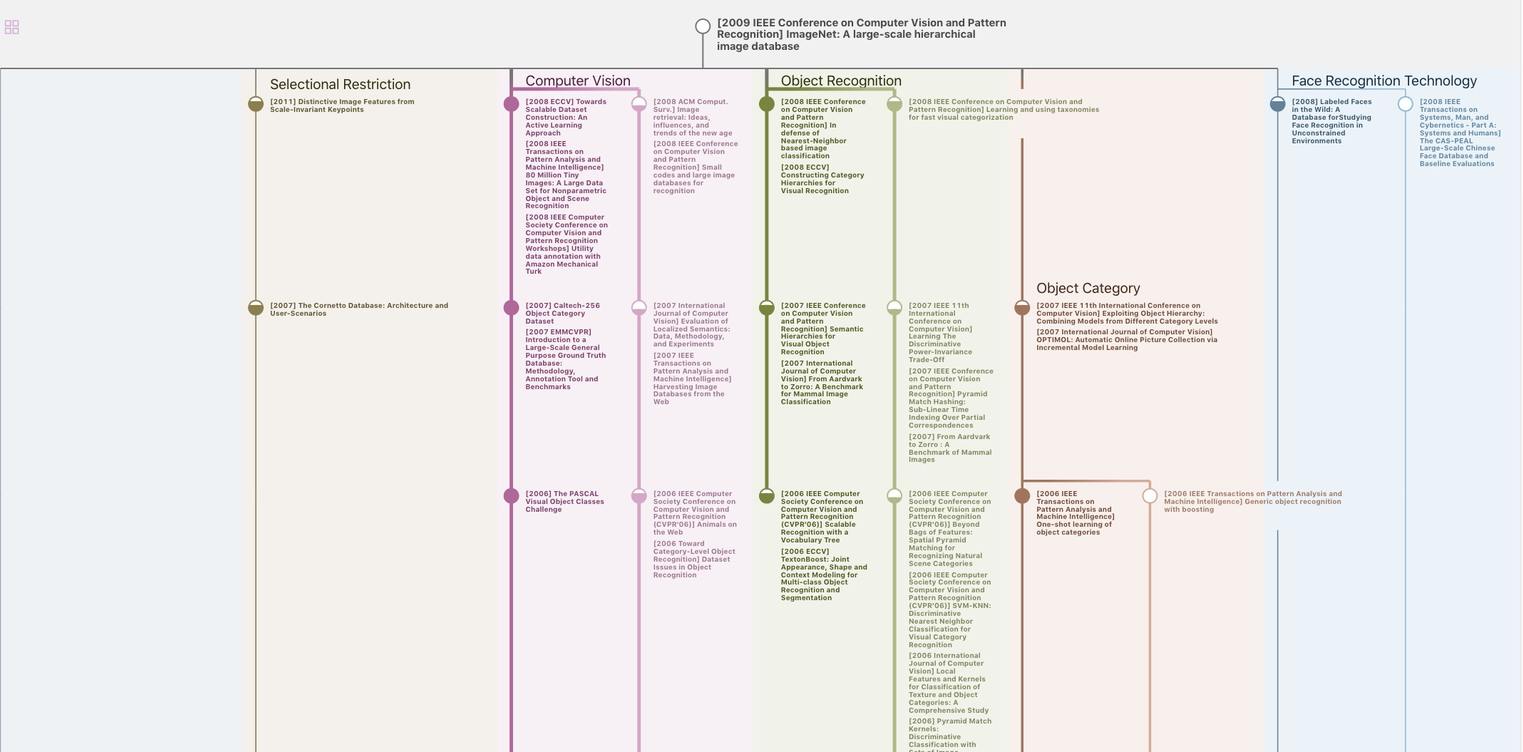
生成溯源树,研究论文发展脉络
Chat Paper
正在生成论文摘要