Wine feature importance and quality prediction: A comparative study of machine learning algorithms with unbalanced data
Lecture Notes of the Institute for Computer Sciences, Social Informatics and Telecommunications Engineering Safe, Secure, Ethical, Responsible Technologies and Emerging Applications(2023)
摘要
Classifying wine as "good" is a challenging task due to the absence of a clear criterion. Nevertheless, an accurate prediction of wine quality can be valuable in the certification phase. Previously, wine quality was evaluated solely by human experts, but with the advent of machine learning this evaluation process can now be automated, thereby reducing the time and effort required from experts. The feature selection process can be utilized to examine the impact of analytical tests on wine quality. If it is established that specific input variables have a significant effect on predicting wine quality, this information can be employed to enhance the production process. We studied the feature importance, which allowed us to explore various factors that affect the quality of the wine. The feature importance analysis suggests that alcohol significantly impacts wine quality. Furthermore, several machine learning models are compared, including Random Forest (RF), Support Vector Machine (SVM), Gradient Boosting (GB), K-Nearest Neighbors (KNN), and Decision Tree (DT). The analysis revealed that SVM excelled above all other models with a 96\% accuracy rate.
更多查看译文
AI 理解论文
溯源树
样例
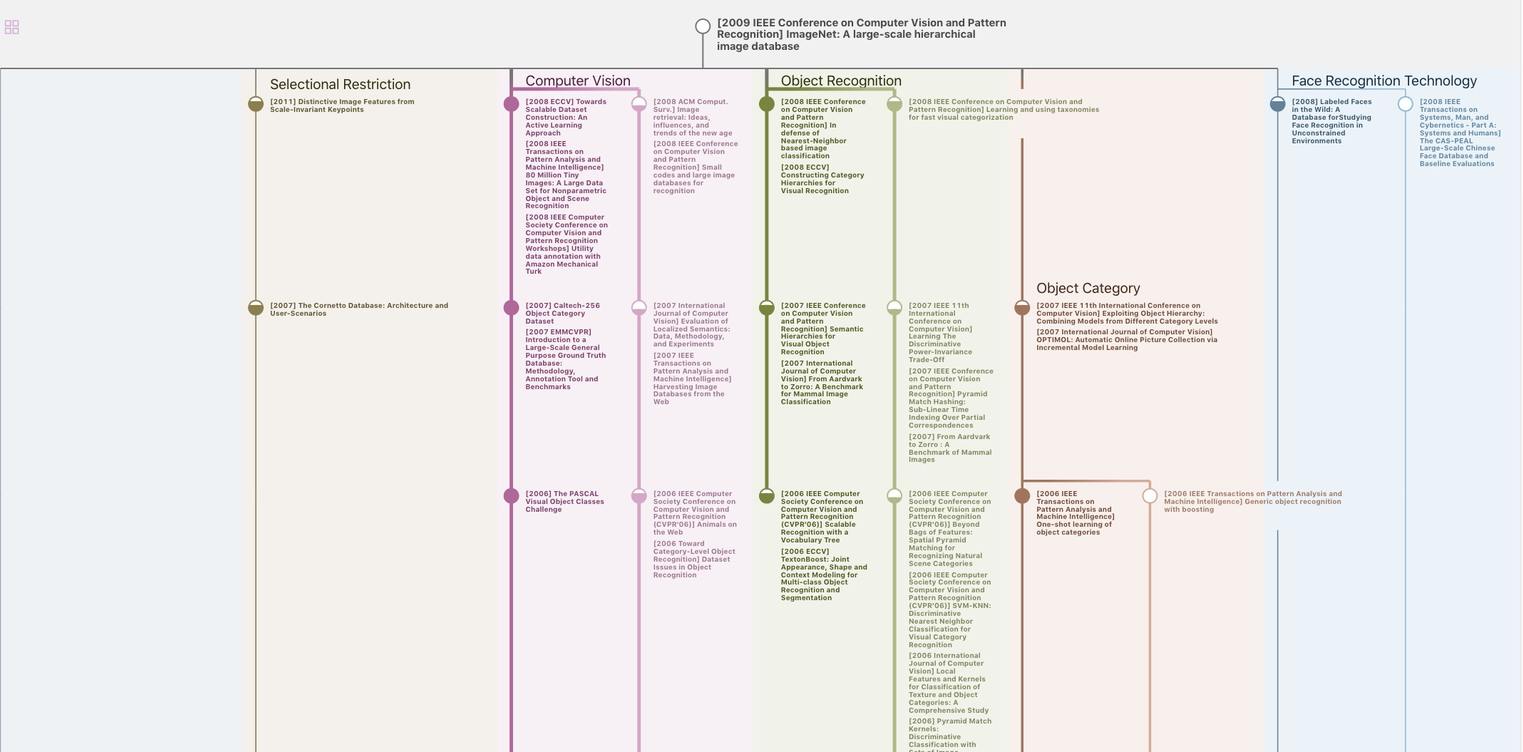
生成溯源树,研究论文发展脉络
Chat Paper
正在生成论文摘要