Attention Sorting Combats Recency Bias In Long Context Language Models
CoRR(2023)
摘要
Current language models often fail to incorporate long contexts efficiently during generation. We show that a major contributor to this issue are attention priors that are likely learned during pre-training: relevant information located earlier in context is attended to less on average. Yet even when models fail to use the information from a relevant document in their response, they still pay preferential attention to that document compared to an irrelevant document at the same position. We leverage this fact to introduce ``attention sorting'': perform one step of decoding, sort documents by the attention they receive (highest attention going last), repeat the process, generate the answer with the newly sorted context. We find that attention sorting improves performance of long context models. Our findings highlight some challenges in using off-the-shelf language models for retrieval augmented generation.
更多查看译文
关键词
language,context
AI 理解论文
溯源树
样例
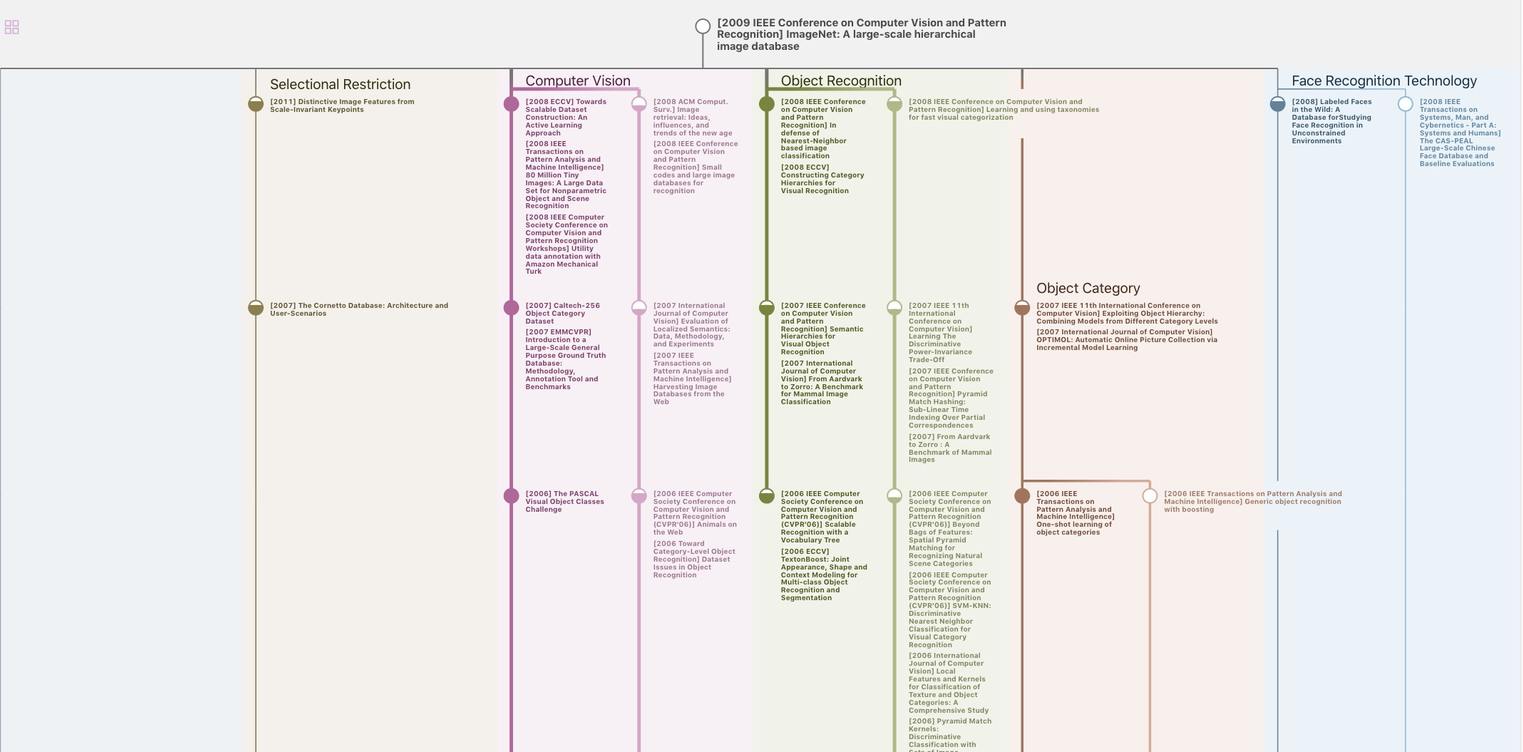
生成溯源树,研究论文发展脉络
Chat Paper
正在生成论文摘要