A Comparative Study of Machine Learning Algorithms and SIFT for Identifying the Quality of Human Induced Pluripotent Stem Cell Colony
2023 IEEE Conference on Computational Intelligence in Bioinformatics and Computational Biology (CIBCB)(2023)
Abstract
Personalized medicine took giant steps further when the human induced pluripotent stem cell (hiPSC) technology was introduced. hiPSC technology enables reprogramming human somatic cells back to stem cells and differentiate them into any cell type wanted, and use them, for instance, in treatments and pharmacological research. Growing and differentiating hiPSCs is a sensitive process that requires constant monitoring since during the growth cycle unwanted changes in hiPSC colonies may occur which prevents their further use. To automate the monitoring and the quality control of hiPSC colonies, we need computational tools. In this paper, we examine the quality identification of hiPSC colony images by comparing a collection of machine learning methods and applying Scaled Invariant Feature Transformation (SIFT) algorithm in classification. Our dataset consists of altogether 229 hiPSC colony images from good/semigood/bad classes and having three quality classes separates our study from the other researches in this field. We obtained 73.4% accuracy using K-Nearest Neighbor (KNN) classifier which outperforms the results from our earlier researches with above 10%. The results show that the quality control of hiPSC colonies can be performed with good accuracy and with methods that are transparent and suitable in medical domain.
MoreTranslated text
Key words
Machine learning, SIFT, Human induced pluripotent stem cell, Personalized medicine
AI Read Science
Must-Reading Tree
Example
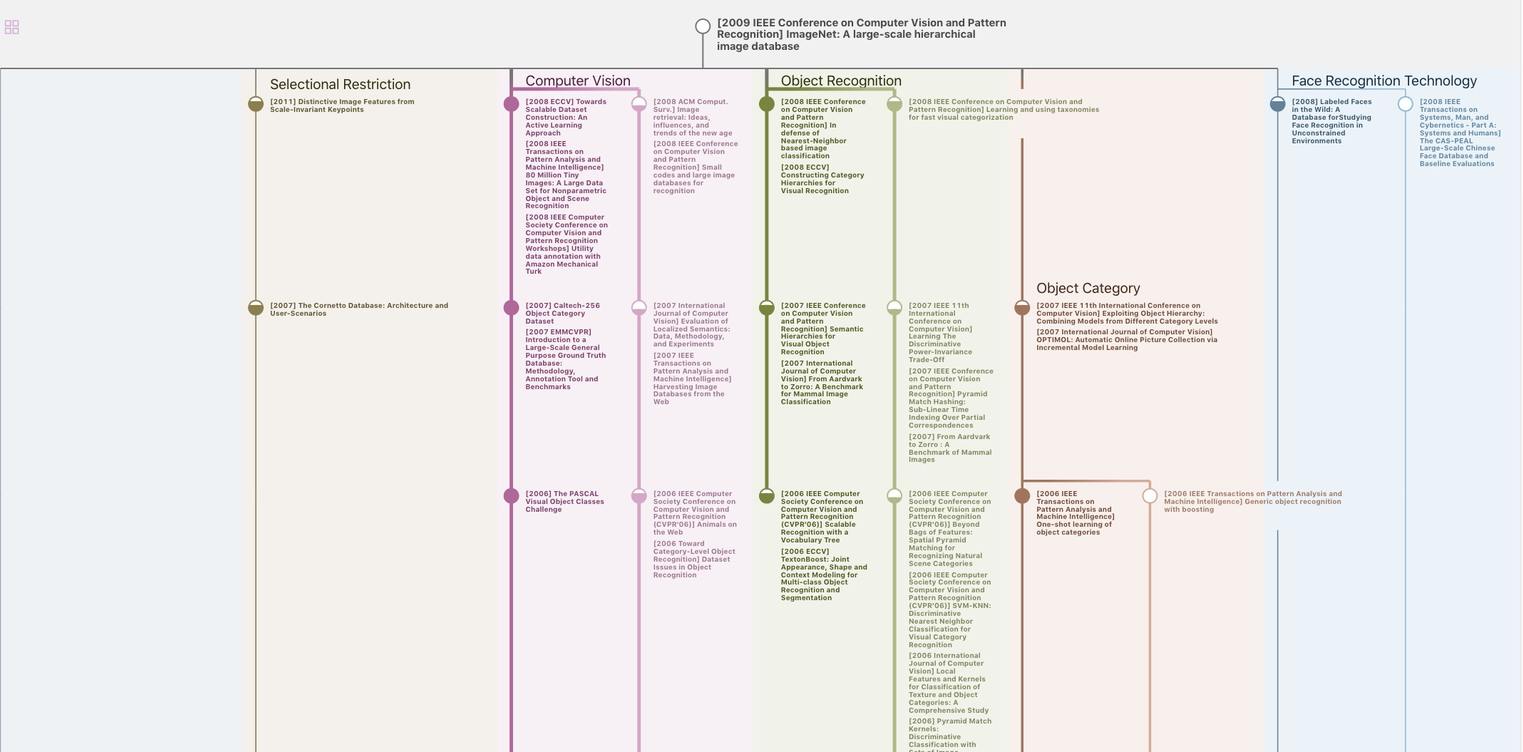
Generate MRT to find the research sequence of this paper
Chat Paper
Summary is being generated by the instructions you defined