Architecture Optimization of a CNN Media Noise Estimator for TDMR
IEEE Transactions on Magnetics(2023)
Key words
TDMR,media noise estimation,convolutional neural network (CNN)
AI Read Science
Must-Reading Tree
Example
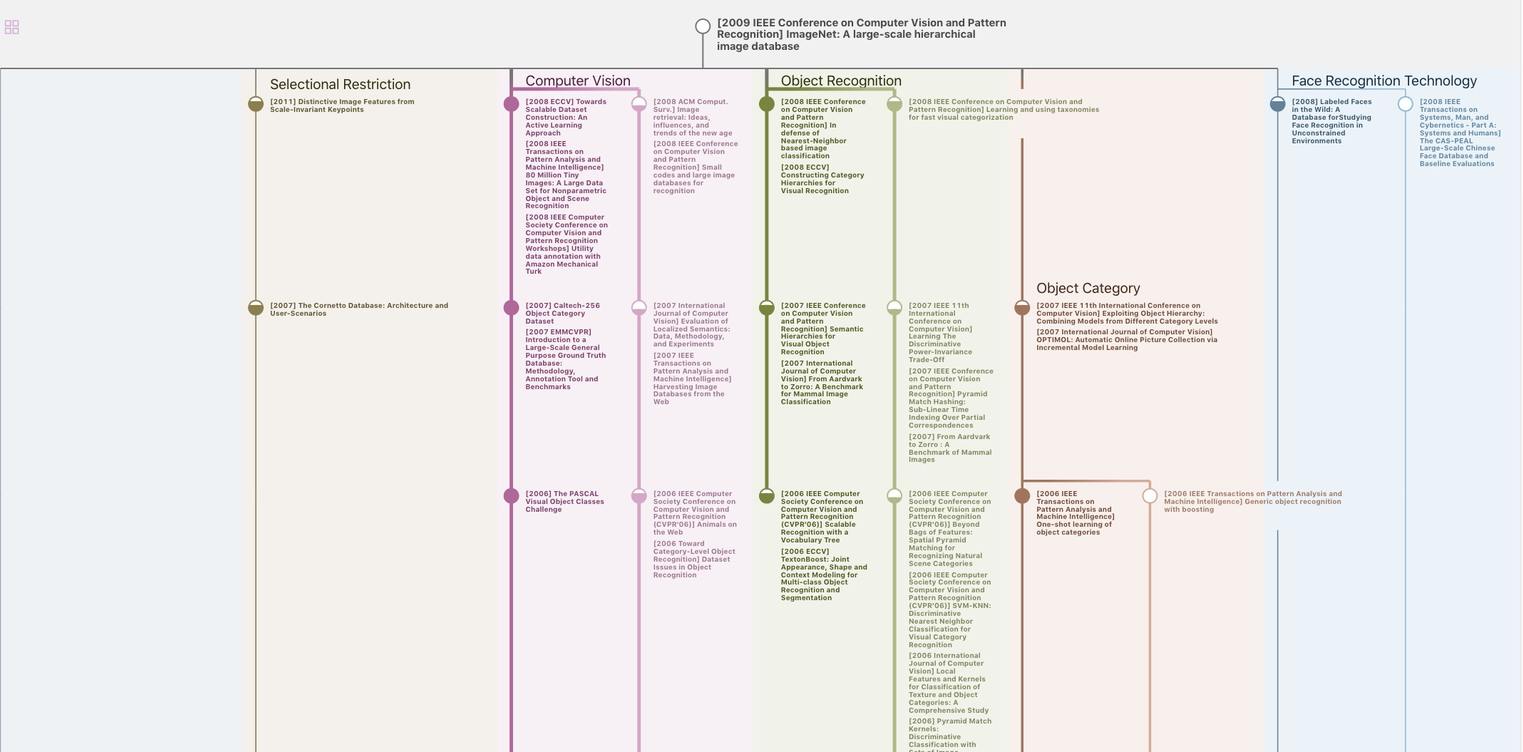
Generate MRT to find the research sequence of this paper
Chat Paper
Summary is being generated by the instructions you defined