Multi-label residual weighted learning for individualized combination treatment rule
ELECTRONIC JOURNAL OF STATISTICS(2024)
摘要
Individualized treatment rules (ITRs) have been widely applied in many fields such as precision medicine and personalized marketing. Beyond the extensive studies on ITR for binary or multiple treatments, there is considerable interest in applying combination treatments. This paper introduces a novel ITR estimation method for combination treatments incorporating interaction effects among treatments. Specifically, we propose the generalized psi-loss as a non -convex surrogate in the residual weighted learning framework, offering desirable statistical and computational properties. Statistically, the minimizer of the proposed surrogate loss is Fisherconsistent with the optimal decision rules, incorporating interaction effects at any intensity level - a significant improvement over existing methods. Computationally, the proposed method applies the difference -of -convex algorithm for efficient computation. Through simulation studies and realworld data applications, we demonstrate the superior performance of the proposed method in recommending combination treatments.
更多查看译文
关键词
Combination therapy,decision making,difference of convex,Fisher consistency,precision medicine
AI 理解论文
溯源树
样例
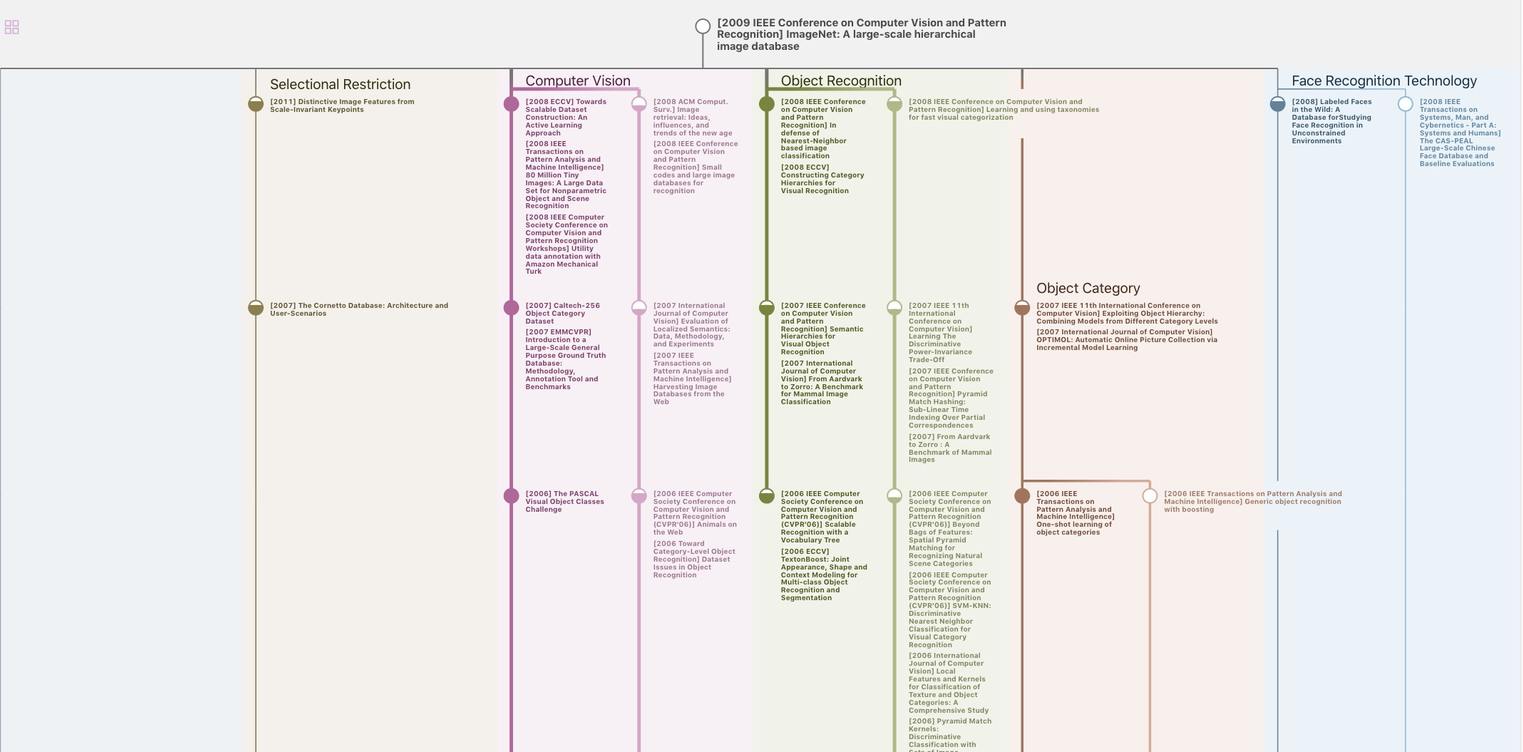
生成溯源树,研究论文发展脉络
Chat Paper
正在生成论文摘要