Sequential Data Generation with Groupwise Diffusion Process
CoRR(2023)
摘要
We present the Groupwise Diffusion Model (GDM), which divides data into multiple groups and diffuses one group at one time interval in the forward diffusion process. GDM generates data sequentially from one group at one time interval, leading to several interesting properties. First, as an extension of diffusion models, GDM generalizes certain forms of autoregressive models and cascaded diffusion models. As a unified framework, GDM allows us to investigate design choices that have been overlooked in previous works, such as data-grouping strategy and order of generation. Furthermore, since one group of the initial noise affects only a certain group of the generated data, latent space now possesses group-wise interpretable meaning. We can further extend GDM to the frequency domain where the forward process sequentially diffuses each group of frequency components. Dividing the frequency bands of the data as groups allows the latent variables to become a hierarchical representation where individual groups encode data at different levels of abstraction. We demonstrate several applications of such representation including disentanglement of semantic attributes, image editing, and generating variations.
更多查看译文
关键词
sequential data,diffusion,process,generation
AI 理解论文
溯源树
样例
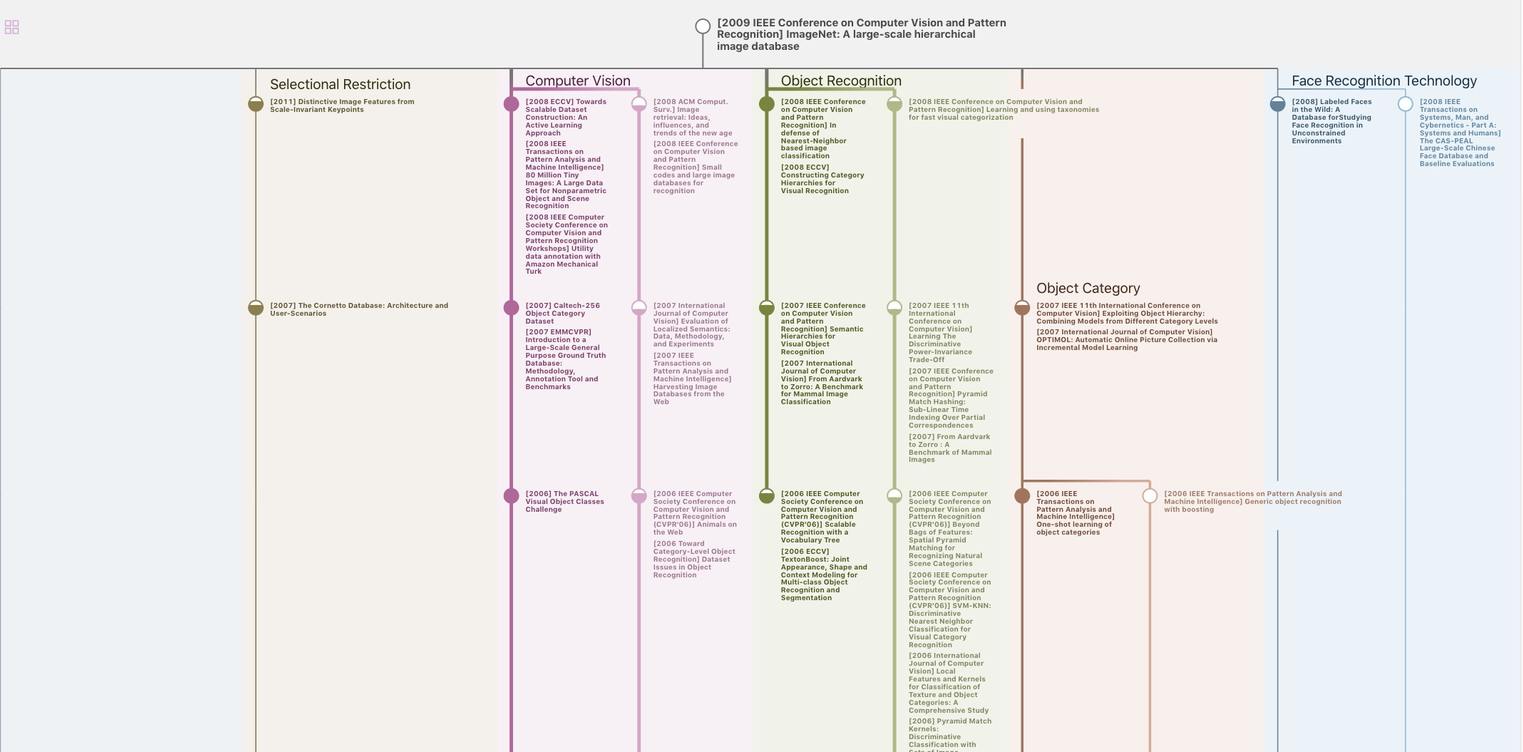
生成溯源树,研究论文发展脉络
Chat Paper
正在生成论文摘要