TACTiS-2: Better, Faster, Simpler Attentional Copulas for Multivariate Time Series
ICLR 2024(2023)
摘要
We introduce a new model for multivariate probabilistic time series prediction, designed to flexibly address a range of tasks including forecasting, interpolation, and their combinations. Building on copula theory, we propose a simplified objective for the recently-introduced transformer-based attentional copulas (TACTiS), wherein the number of distributional parameters now scales linearly with the number of variables instead of factorially. The new objective requires the introduction of a training curriculum, which goes hand-in-hand with necessary changes to the original architecture. We show that the resulting model has significantly better training dynamics and achieves state-of-the-art performance across diverse real-world forecasting tasks, while maintaining the flexibility of prior work, such as seamless handling of unaligned and unevenly-sampled time series.
更多查看译文
关键词
time series,forecasting,probabilistic,multivariate,copula,transformer,density estimation
AI 理解论文
溯源树
样例
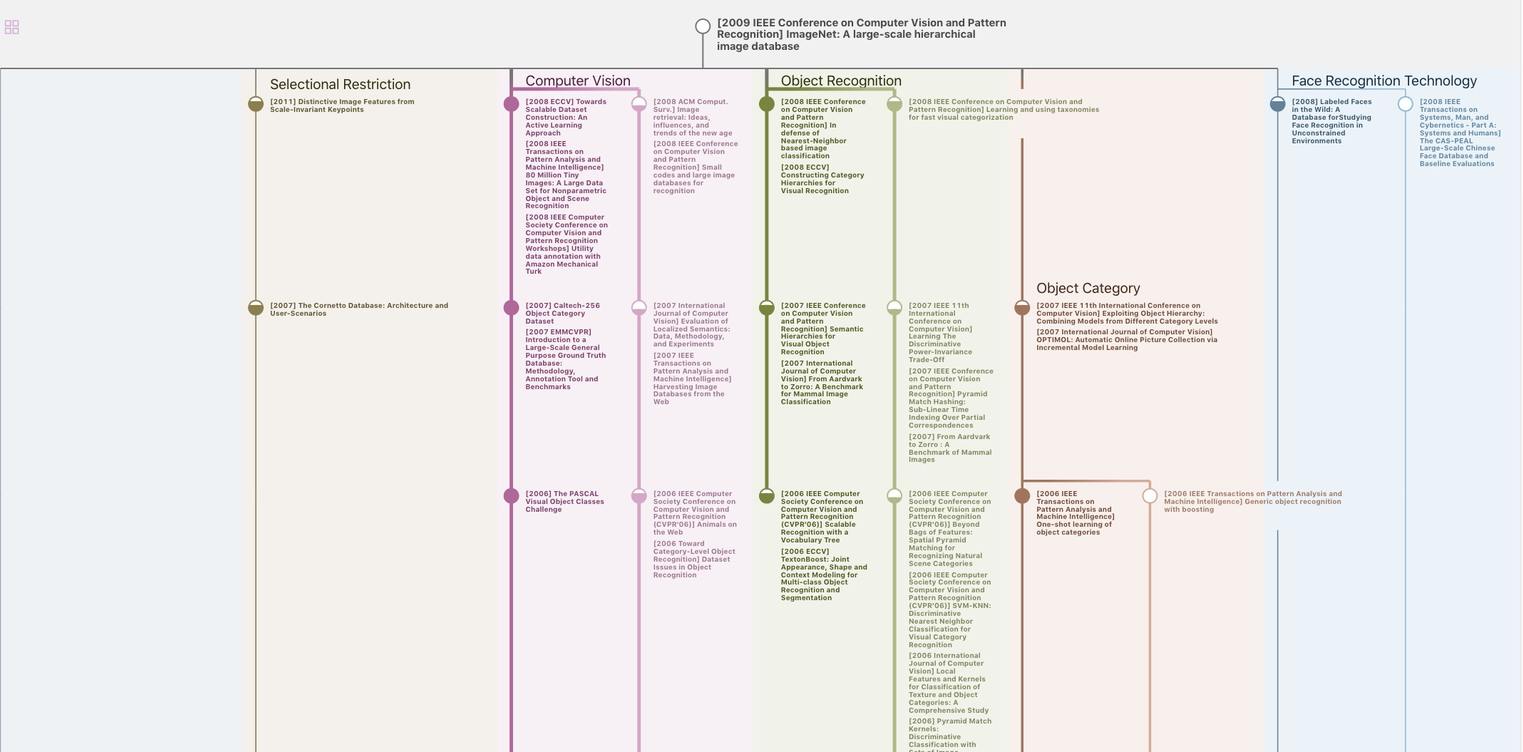
生成溯源树,研究论文发展脉络
Chat Paper
正在生成论文摘要