Quantifying the Plausibility of Context Reliance in Neural Machine Translation
arxiv(2023)
摘要
Establishing whether language models can use contextual information in a
human-plausible way is important to ensure their trustworthiness in real-world
settings. However, the questions of when and which parts of the context affect
model generations are typically tackled separately, with current plausibility
evaluations being practically limited to a handful of artificial benchmarks. To
address this, we introduce Plausibility Evaluation of Context Reliance
(PECoRe), an end-to-end interpretability framework designed to quantify context
usage in language models' generations. Our approach leverages model internals
to (i) contrastively identify context-sensitive target tokens in generated
texts and (ii) link them to contextual cues justifying their prediction. We use
to quantify the plausibility of context-aware machine translation
models, comparing model rationales with human annotations across several
discourse-level phenomena. Finally, we apply our method to unannotated model
translations to identify context-mediated predictions and highlight instances
of (im)plausible context usage throughout generation.
更多查看译文
AI 理解论文
溯源树
样例
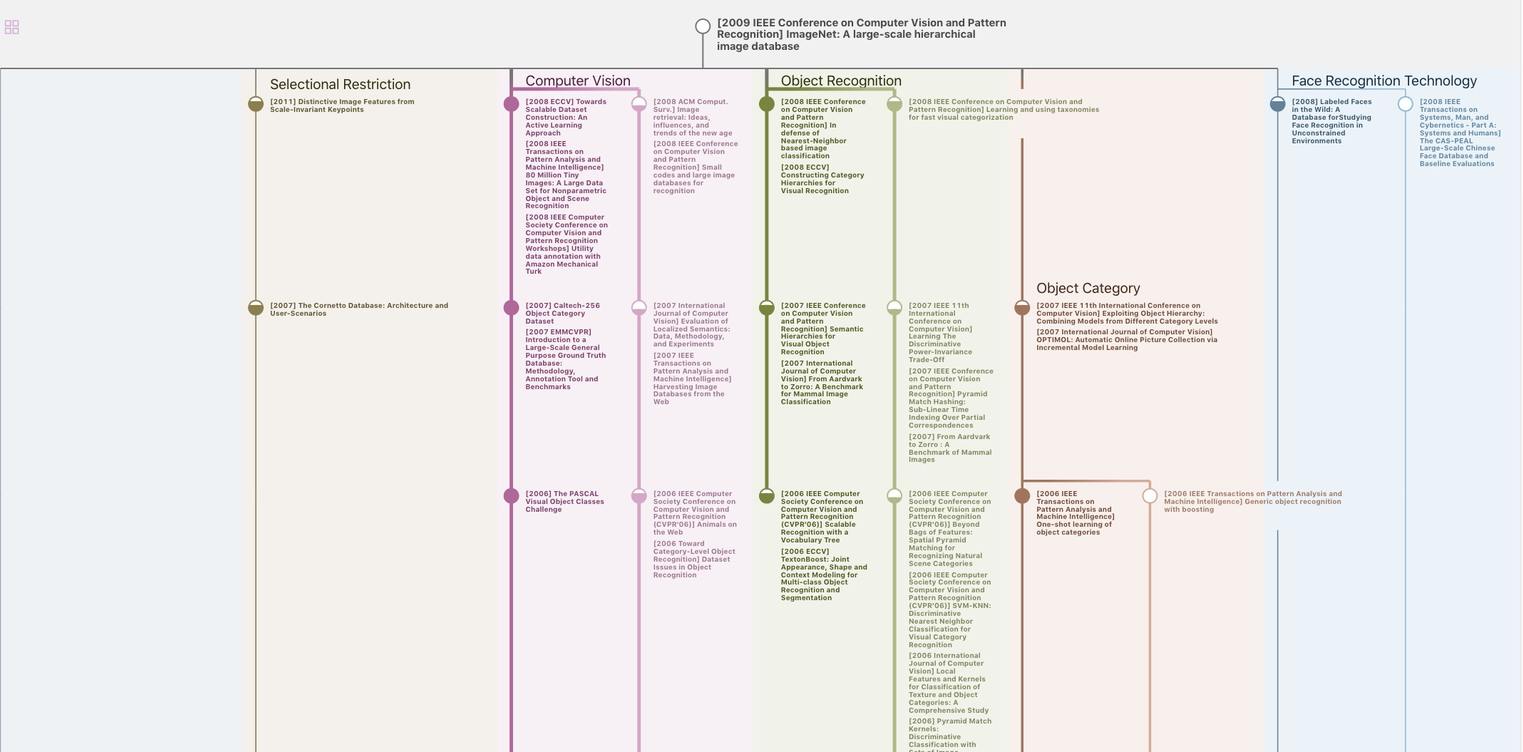
生成溯源树,研究论文发展脉络
Chat Paper
正在生成论文摘要