Improved Variational Bayesian Phylogenetic Inference using Mixtures
CoRR(2023)
摘要
We present VBPI-Mixtures, an algorithm designed to enhance the accuracy of phylogenetic posterior distributions, particularly for tree-topology and branch-length approximations. Despite the Variational Bayesian Phylogenetic Inference (VBPI), a leading-edge black-box variational inference (BBVI) framework, achieving remarkable approximations of these distributions, the multimodality of the tree-topology posterior presents a formidable challenge to sampling-based learning techniques such as BBVI. Advanced deep learning methodologies such as normalizing flows and graph neural networks have been explored to refine the branch-length posterior approximation, yet efforts to ameliorate the posterior approximation over tree topologies have been lacking. Our novel VBPI-Mixtures algorithm bridges this gap by harnessing the latest breakthroughs in mixture learning within the BBVI domain. As a result, VBPI-Mixtures is capable of capturing distributions over tree-topologies that VBPI fails to model. We deliver state-of-the-art performance on difficult density estimation tasks across numerous real phylogenetic datasets.
更多查看译文
关键词
inference
AI 理解论文
溯源树
样例
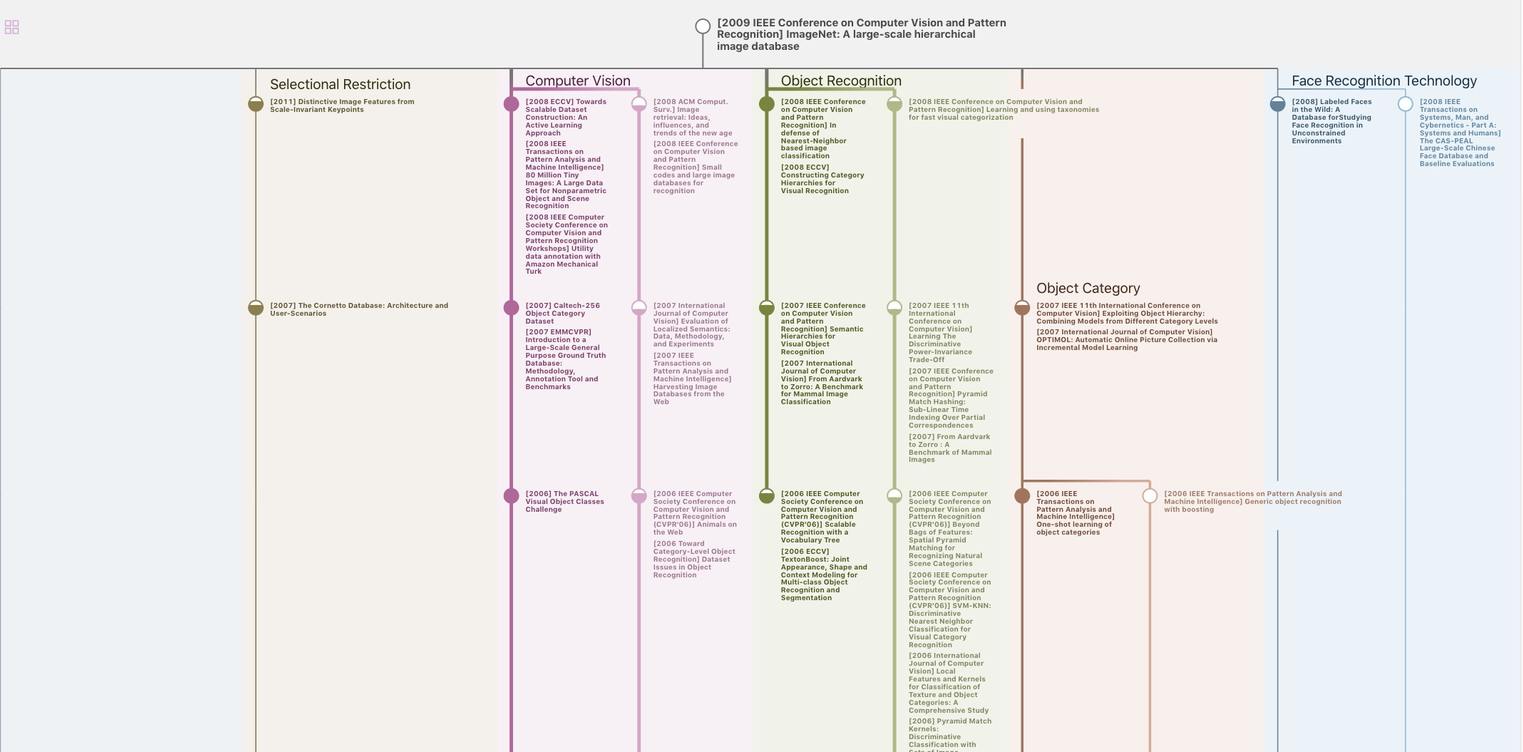
生成溯源树,研究论文发展脉络
Chat Paper
正在生成论文摘要