How Close are Other Computer Vision Tasks to Deepfake Detection?
2023 IEEE International Joint Conference on Biometrics (IJCB)(2023)
摘要
In this paper, we challenge the conventional belief that supervised ImageNet-trained models have strong generalizability and are suitable for use as feature extractors in deepfake detection. We present a new measurement, "model separability," for visually and quantitatively assessing a model's raw capacity to separate data in an unsupervised manner. We also present a systematic benchmark for determining the correlation between deepfake detection and other computer vision tasks using pre-trained models. Our analysis shows that pre-trained face recognition models are more closely related to deepfake detection than other models. Additionally, models trained using self-supervised methods are more effective in separation than those trained using supervised methods. After fine-tuning all models on a small deepfake dataset, we found that self-supervised models deliver the best results, but there is a risk of overfitting. Our results provide valuable insights that should help researchers and practitioners develop more effective deepfake detection models.
更多查看译文
关键词
Deepfake Detection,Face Recognition,Unsupervised Manner,Model Selection,Image Classification,Dataset Size,Transfer Learning,Training Methods,ImageNet,Generative Adversarial Networks,Latent Space,Face Images,Model Discrimination,Unseen Data,Self-supervised Learning,Number Of Annotations,Fine-tuned Model,Dimensionality Reduction Algorithms,Face Recognition Task,Poor Separation,Fake Images,Backbone Architecture,High True Positive Rate,Decision Boundary,Age Estimation,Deep Learning,Largest Dataset,Training Dataset,Training Data
AI 理解论文
溯源树
样例
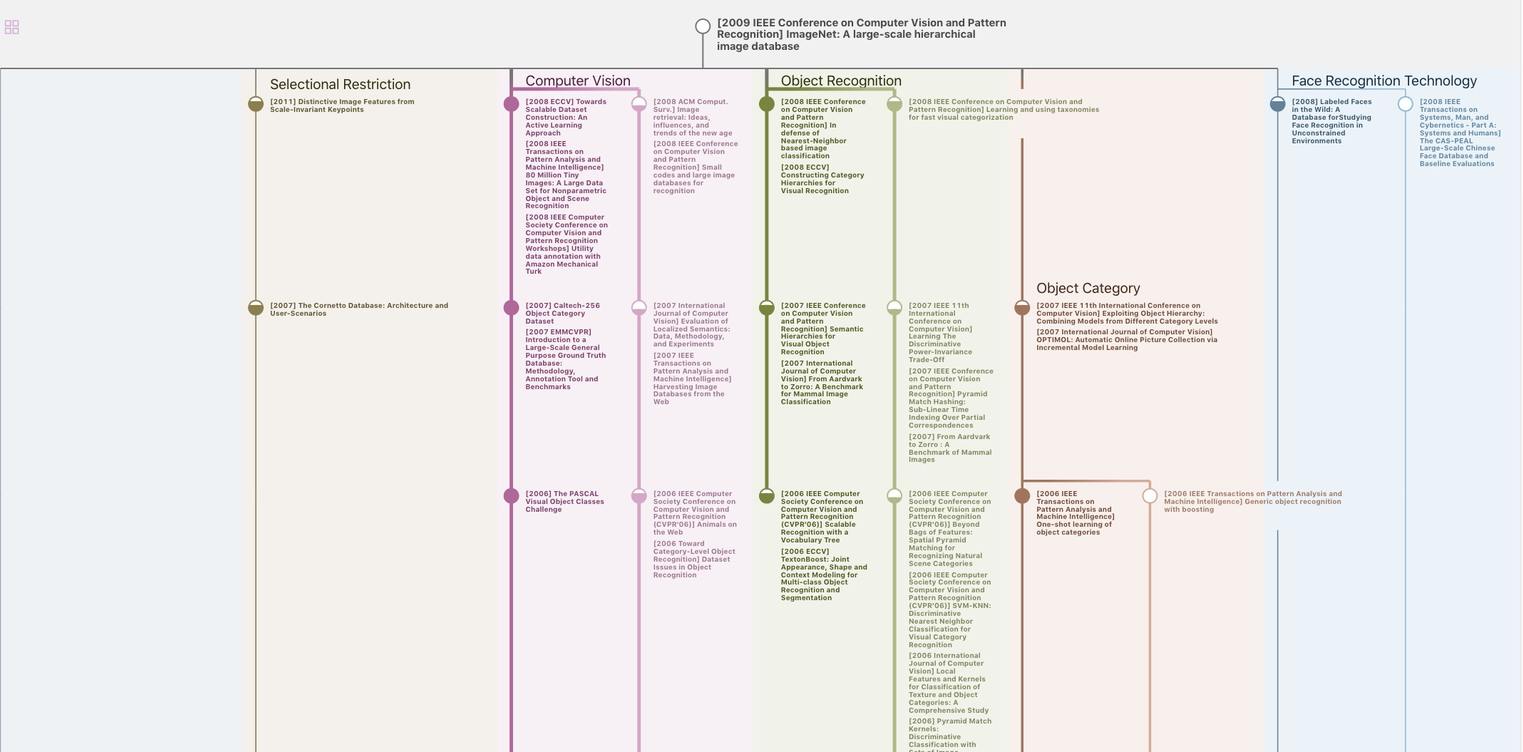
生成溯源树,研究论文发展脉络
Chat Paper
正在生成论文摘要