RT-GAN: Recurrent Temporal GAN for Adding Lightweight Temporal Consistency to Frame-Based Domain Translation Approaches
CoRR(2023)
摘要
While developing new unsupervised domain translation methods for endoscopy videos, it is typical to start with approaches that initially work for individual frames without temporal consistency. Once an individual-frame model has been finalized, additional contiguous frames are added with a modified deep learning architecture to train a new model for temporal consistency. This transition to temporally-consistent deep learning models, however, requires significantly more computational and memory resources for training. In this paper, we present a lightweight solution with a tunable temporal parameter, RT-GAN (Recurrent Temporal GAN), for adding temporal consistency to individual frame-based approaches that reduces training requirements by a factor of 5. We demonstrate the effectiveness of our approach on two challenging use cases in colonoscopy: haustral fold segmentation (indicative of missed surface) and realistic colonoscopy simulator video generation. The datasets, accompanying code, and pretrained models will be made available at \url{https://github.com/nadeemlab/CEP}.
更多查看译文
关键词
recurrent temporal rt-gan,domain translation approaches,lightweight temporal,frame-based
AI 理解论文
溯源树
样例
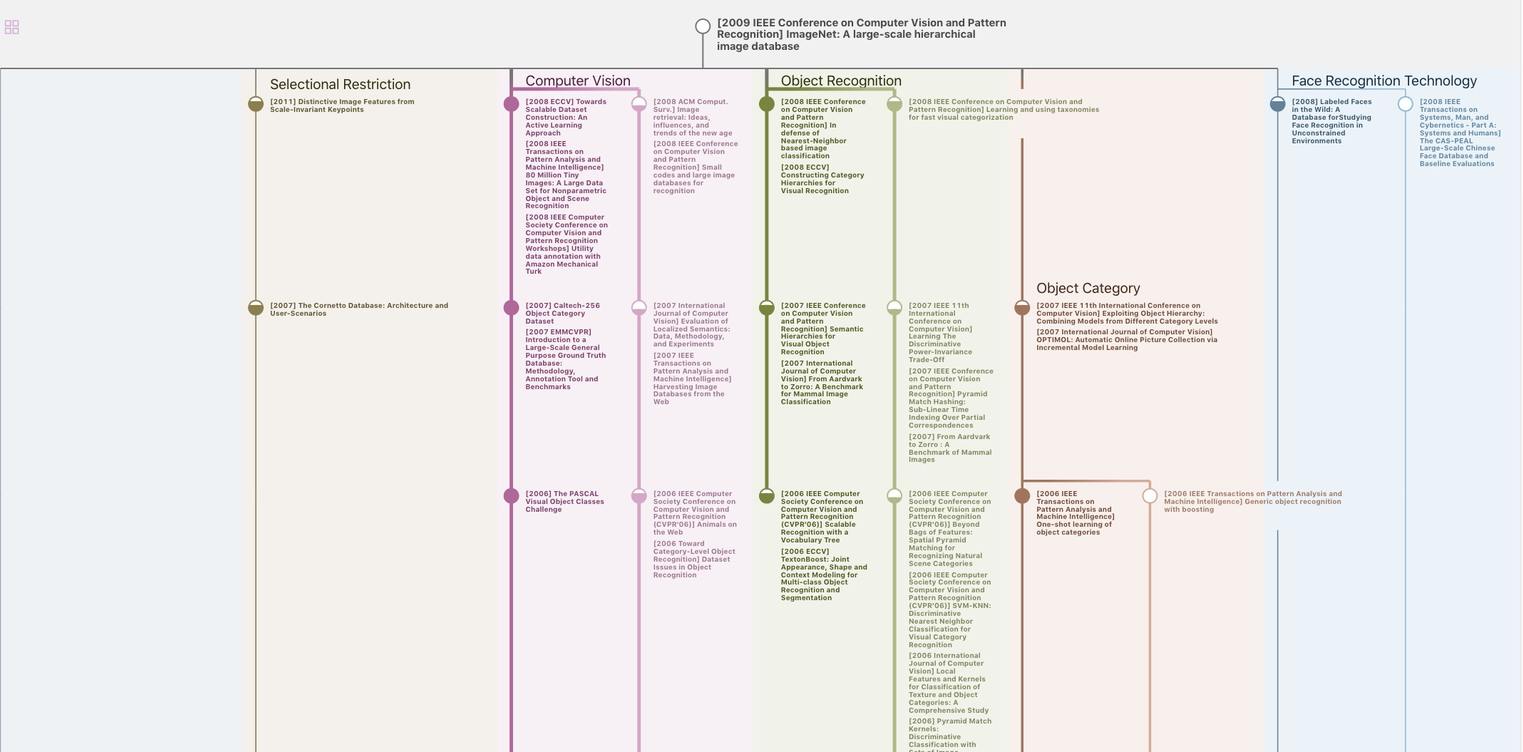
生成溯源树,研究论文发展脉络
Chat Paper
正在生成论文摘要