Diff-DOPE: Differentiable Deep Object Pose Estimation
CoRR(2023)
摘要
We introduce Diff-DOPE, a 6-DoF pose refiner that takes as input an image, a 3D textured model of an object, and an initial pose of the object. The method uses differentiable rendering to update the object pose to minimize the visual error between the image and the projection of the model. We show that this simple, yet effective, idea is able to achieve state-of-the-art results on pose estimation datasets. Our approach is a departure from recent methods in which the pose refiner is a deep neural network trained on a large synthetic dataset to map inputs to refinement steps. Rather, our use of differentiable rendering allows us to avoid training altogether. Our approach performs multiple gradient descent optimizations in parallel with different random learning rates to avoid local minima from symmetric objects, similar appearances, or wrong step size. Various modalities can be used, e.g., RGB, depth, intensity edges, and object segmentation masks. We present experiments examining the effect of various choices, showing that the best results are found when the RGB image is accompanied by an object mask and depth image to guide the optimization process.
更多查看译文
关键词
object,diff-dope
AI 理解论文
溯源树
样例
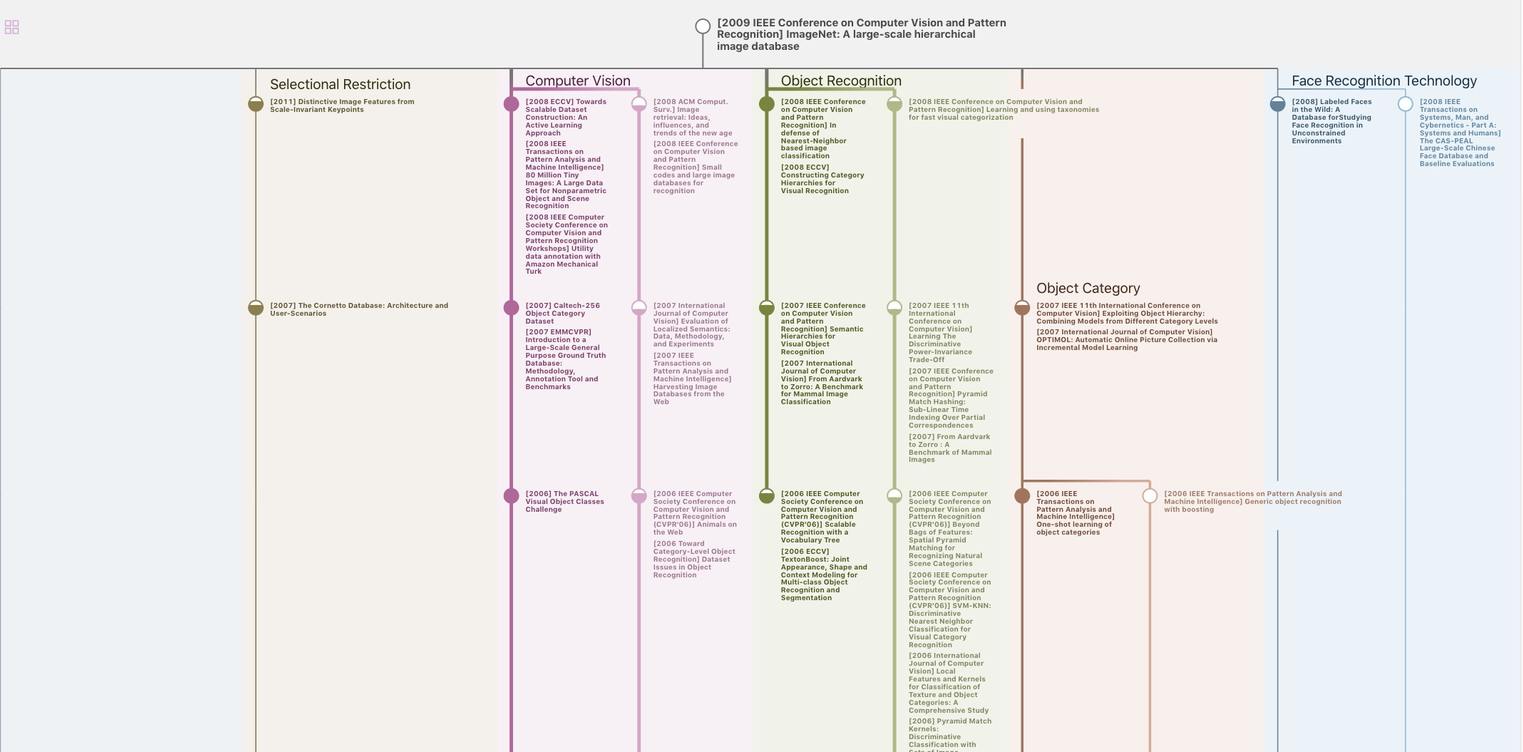
生成溯源树,研究论文发展脉络
Chat Paper
正在生成论文摘要