SSIF: Learning Continuous Image Representation for Spatial-Spectral Super-Resolution
CoRR(2023)
摘要
Existing digital sensors capture images at fixed spatial and spectral resolutions (e.g., RGB, multispectral, and hyperspectral images), and each combination requires bespoke machine learning models. Neural Implicit Functions partially overcome the spatial resolution challenge by representing an image in a resolution-independent way. However, they still operate at fixed, pre-defined spectral resolutions. To address this challenge, we propose Spatial-Spectral Implicit Function (SSIF), a neural implicit model that represents an image as a function of both continuous pixel coordinates in the spatial domain and continuous wavelengths in the spectral domain. We empirically demonstrate the effectiveness of SSIF on two challenging spatio-spectral super-resolution benchmarks. We observe that SSIF consistently outperforms state-of-the-art baselines even when the baselines are allowed to train separate models at each spectral resolution. We show that SSIF generalizes well to both unseen spatial resolutions and spectral resolutions. Moreover, SSIF can generate high-resolution images that improve the performance of downstream tasks (e.g., land use classification) by 1.7%-7%.
更多查看译文
关键词
continuous image representation,spatial-spectral,super-resolution
AI 理解论文
溯源树
样例
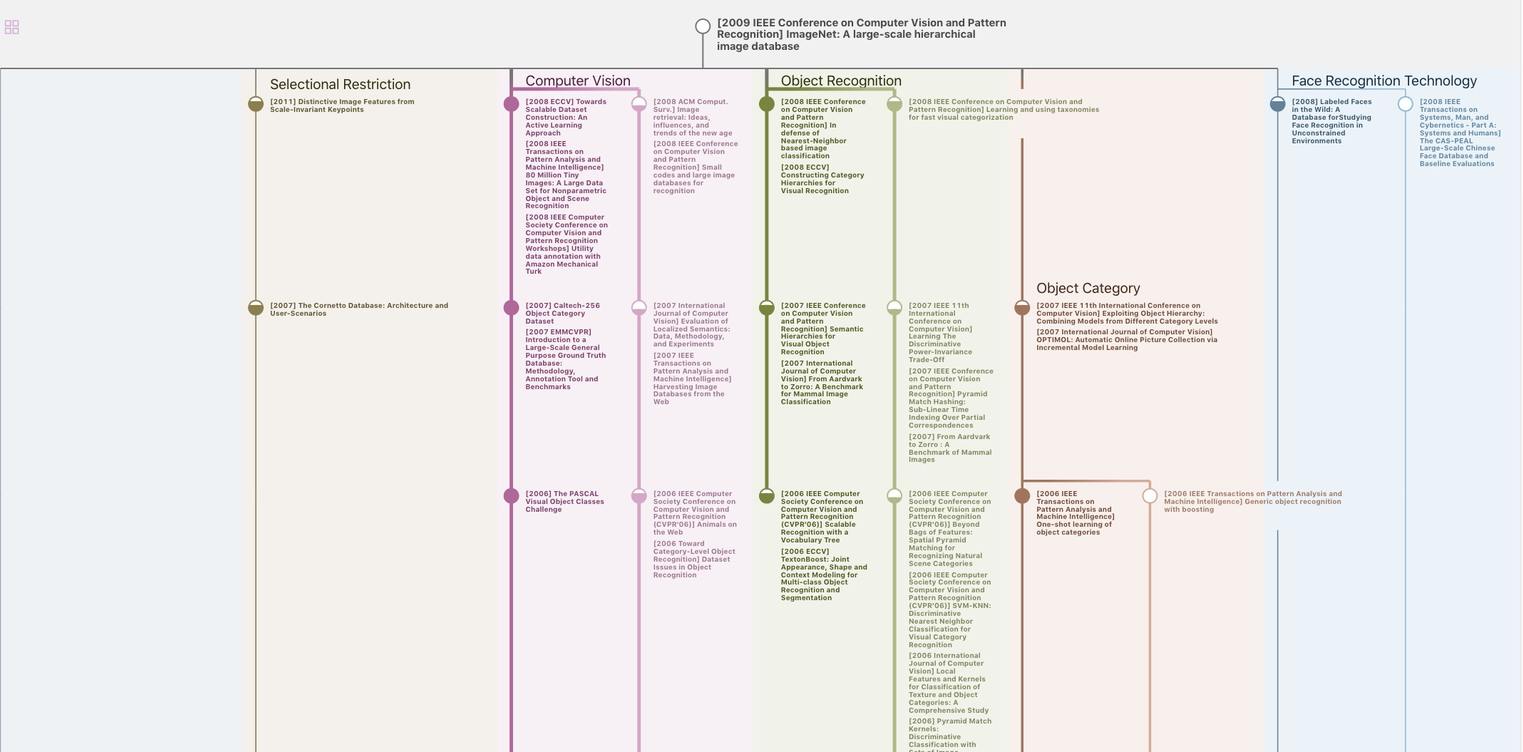
生成溯源树,研究论文发展脉络
Chat Paper
正在生成论文摘要