Anomaly Detection in Power Generation Plants with Generative Adversarial Networks
CoRR(2023)
摘要
Anomaly detection is a critical task that involves the identification of data points that deviate from a predefined pattern, useful for fraud detection and related activities. Various techniques are employed for anomaly detection, but recent research indicates that deep learning methods, with their ability to discern intricate data patterns, are well-suited for this task. This study explores the use of Generative Adversarial Networks (GANs) for anomaly detection in power generation plants. The dataset used in this investigation comprises fuel consumption records obtained from power generation plants operated by a telecommunications company. The data was initially collected in response to observed irregularities in the fuel consumption patterns of the generating sets situated at the company's base stations. The dataset was divided into anomalous and normal data points based on specific variables, with 64.88% classified as normal and 35.12% as anomalous. An analysis of feature importance, employing the random forest classifier, revealed that Running Time Per Day exhibited the highest relative importance. A GANs model was trained and fine-tuned both with and without data augmentation, with the goal of increasing the dataset size to enhance performance. The generator model consisted of five dense layers using the tanh activation function, while the discriminator comprised six dense layers, each integrated with a dropout layer to prevent overfitting. Following data augmentation, the model achieved an accuracy rate of 98.99%, compared to 66.45% before augmentation. This demonstrates that the model nearly perfectly classified data points into normal and anomalous categories, with the augmented data significantly enhancing the GANs' performance in anomaly detection. Consequently, this study recommends the use of GANs, particularly when using large datasets, for effective anomaly detection.
更多查看译文
关键词
Generative adversarial networks,anomaly detection,random forest,power generation plants,telecommunication
AI 理解论文
溯源树
样例
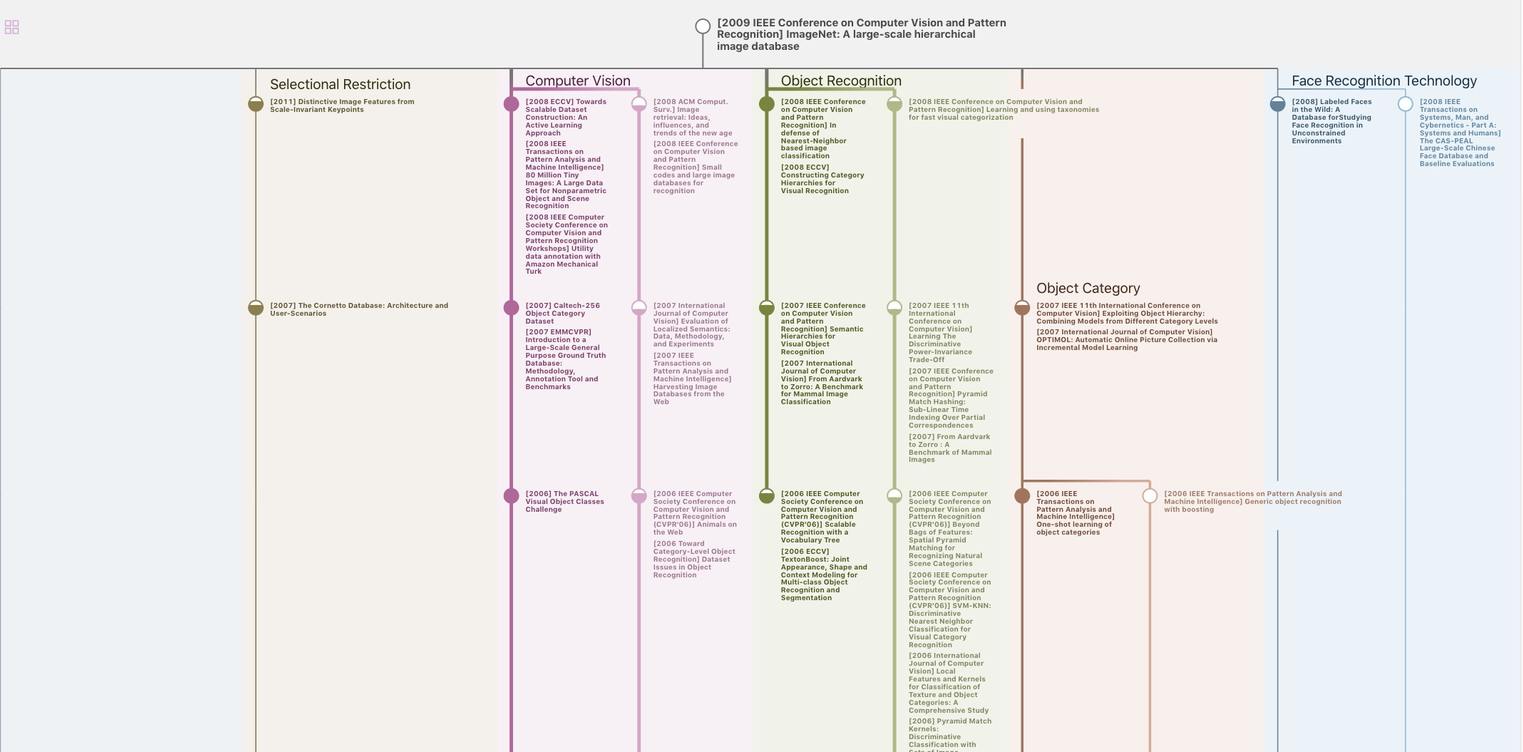
生成溯源树,研究论文发展脉络
Chat Paper
正在生成论文摘要