The Gift of Feedback: Improving ASR Model Quality by Learning from User Corrections through Federated Learning
CoRR(2023)
摘要
Automatic speech recognition (ASR) models are typically trained on large datasets of transcribed speech. As language evolves and new terms come into use, these models can become outdated and stale. In the context of models trained on the server but deployed on edge devices, errors may result from the mismatch between server training data and actual on-device usage. In this work, we seek to continually learn from on-device user corrections through Federated Learning (FL) to address this issue. We explore techniques to target fresh terms that the model has not previously encountered, learn long-tail words, and mitigate catastrophic forgetting. In experimental evaluations, we find that the proposed techniques improve model recognition of fresh terms, while preserving quality on the overall language distribution.
更多查看译文
关键词
speech recognition,federated learning,deep learning,catastrophic forgetting,on-device training
AI 理解论文
溯源树
样例
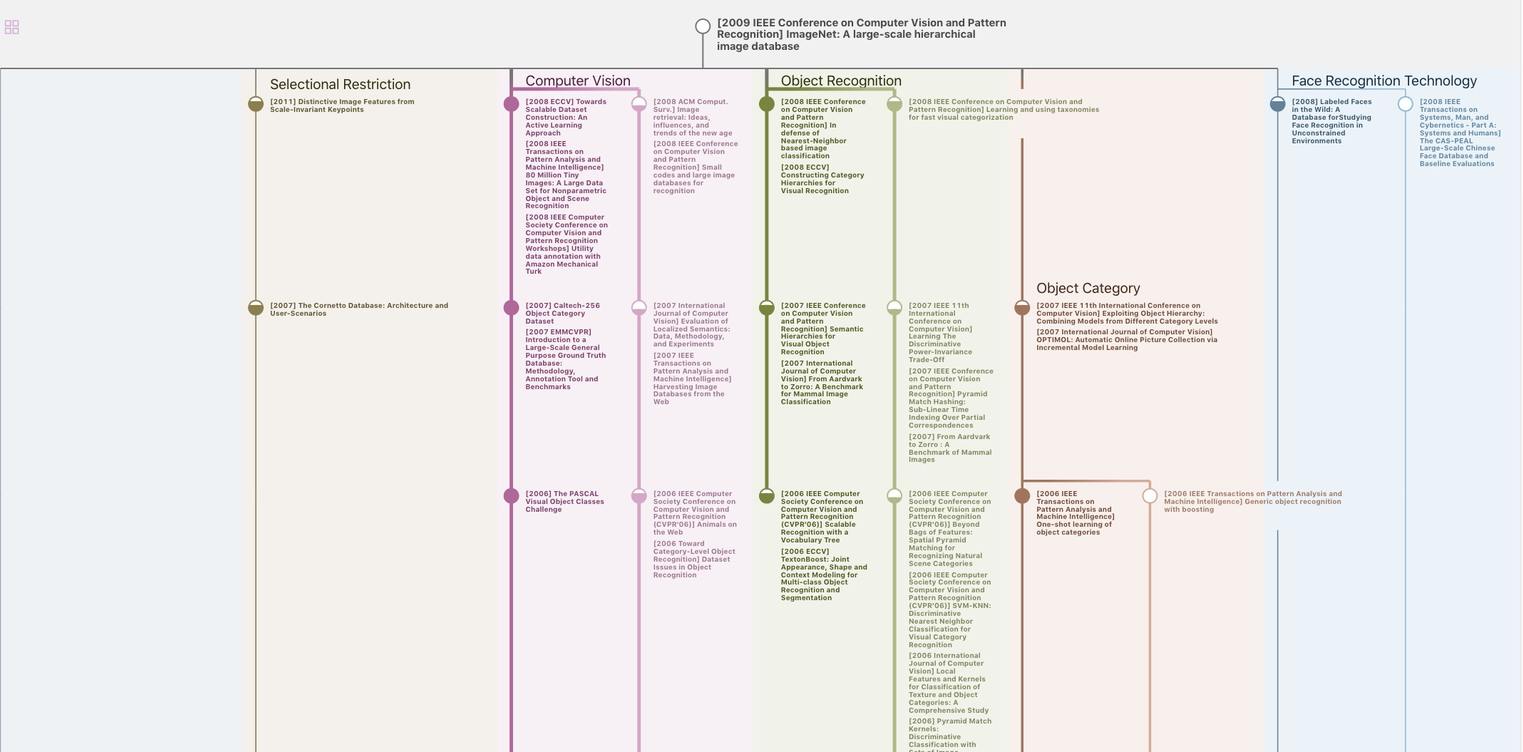
生成溯源树,研究论文发展脉络
Chat Paper
正在生成论文摘要