Adversarial Explainability: Utilizing Explainable Machine Learning in Bypassing IoT Botnet Detection Systems
CoRR(2023)
摘要
Botnet detection based on machine learning have witnessed significant leaps in recent years, with the availability of large and reliable datasets that are extracted from real-life scenarios. Consequently, adversarial attacks on machine learning-based cybersecurity systems are posing a significant threat to the practicality of these solutions. In this paper, we introduce a novel attack that utilizes machine learning model's explainability in evading detection by botnet detection systems. The proposed attack utilizes information obtained from model's explainability to build adversarial samples that can evade detection in a blackbox setting. The proposed attack was tested on a trained IoT botnet detection systems and was capable of bypassing the botnet detection with 0% detection by altering one feature only to generate the adversarial samples.
更多查看译文
关键词
adversarial explainability,explainable machine learning,machine learning,detection
AI 理解论文
溯源树
样例
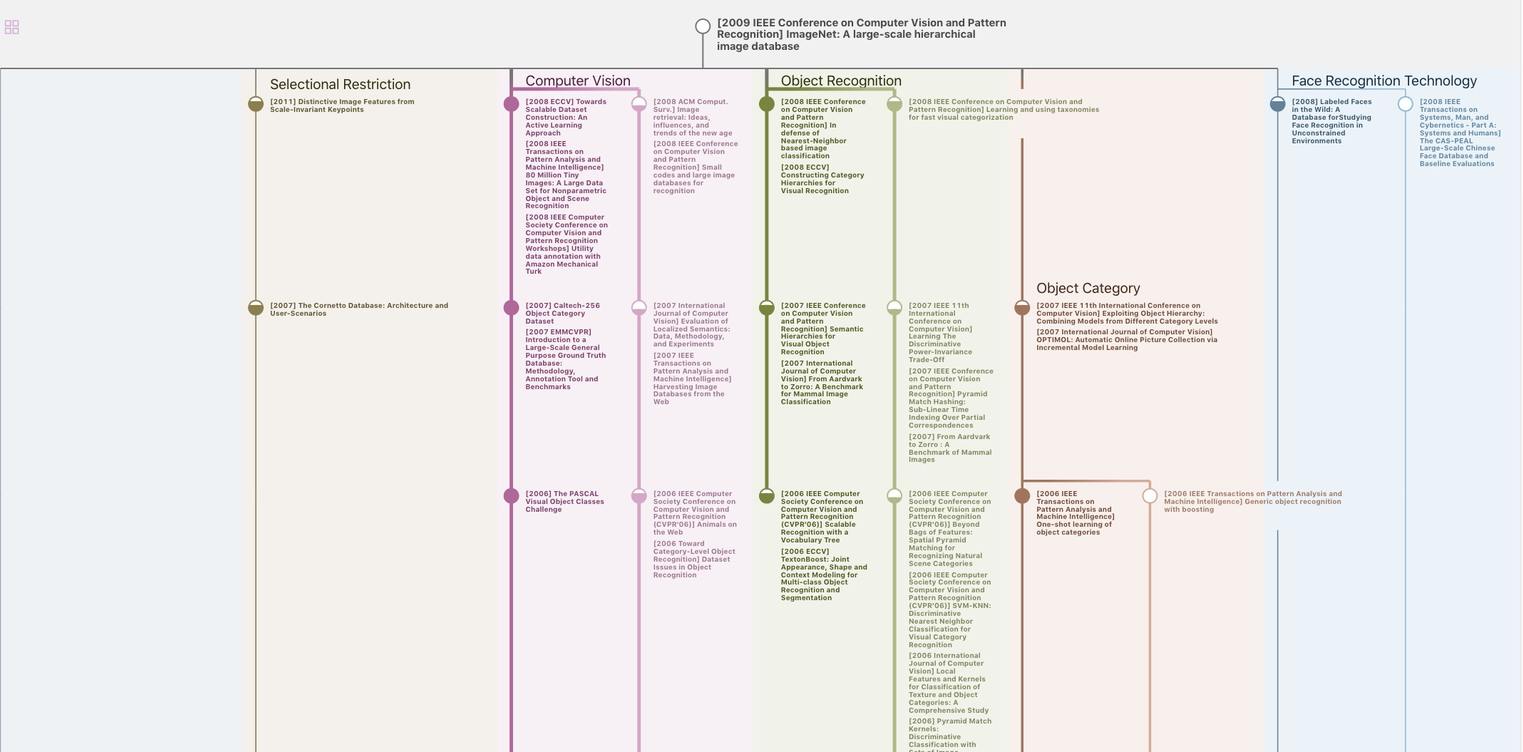
生成溯源树,研究论文发展脉络
Chat Paper
正在生成论文摘要