Prompt-based test-time real image dehazing: a novel pipeline
CoRR(2023)
摘要
Existing methods attempt to improve models' generalization ability on real-world hazy images by exploring well-designed training schemes (e.g., cycleGAN, prior loss). However, most of them need very complicated training procedures to achieve satisfactory results. In this work, we present a totally novel testing pipeline called Prompt-based Test-Time Dehazing (PTTD) to help generate visually pleasing results of real-captured hazy images during the inference phase. We experimentally find that given a dehazing model trained on synthetic data, by fine-tuning the statistics (i.e., mean and standard deviation) of encoding features, PTTD is able to narrow the domain gap, boosting the performance of real image dehazing. Accordingly, we first apply a prompt generation module (PGM) to generate a visual prompt, which is the source of appropriate statistical perturbations for mean and standard deviation. And then, we employ the feature adaptation module (FAM) into the existing dehazing models for adjusting the original statistics with the guidance of the generated prompt. Note that, PTTD is model-agnostic and can be equipped with various state-of-the-art dehazing models trained on synthetic hazy-clean pairs. Extensive experimental results demonstrate that our PTTD is flexible meanwhile achieves superior performance against state-of-the-art dehazing methods in real-world scenarios.
更多查看译文
关键词
real image dehazing,pipeline,prompt-based,test-time
AI 理解论文
溯源树
样例
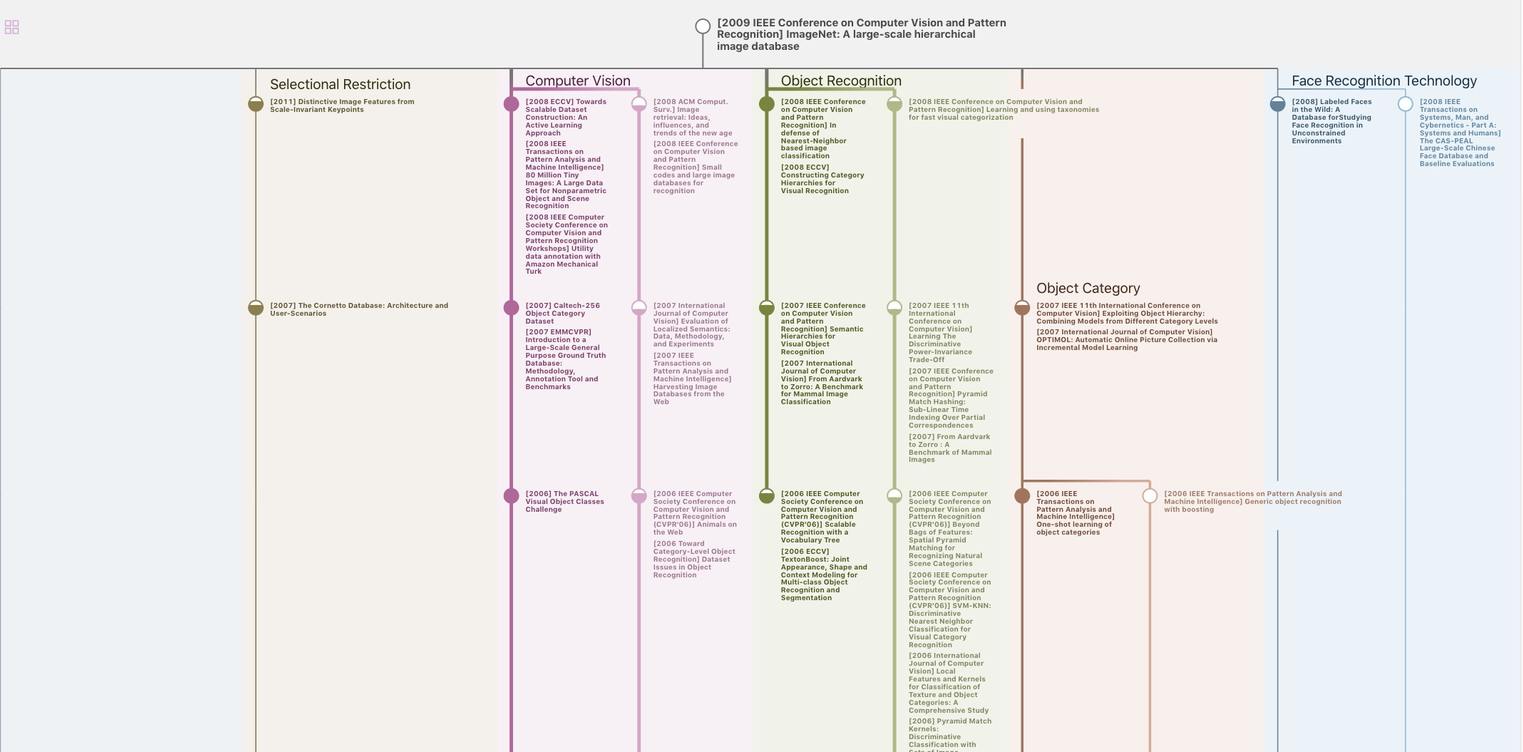
生成溯源树,研究论文发展脉络
Chat Paper
正在生成论文摘要