Multi-depth branch network for efficient image super-resolution
Image and Vision Computing(2024)
摘要
A longstanding challenge in Super-Resolution (SR) is how to efficiently enhance high-frequency details in Low-Resolution (LR) images while maintaining semantic coherence. This is particularly crucial in practical applications where SR models are often deployed on low-power devices. To address this issue, we propose an innovative asymmetric SR architecture featuring Multi-Depth Branch Module (MDBM). These MDBMs contain branches of different depths, designed to capture high- and low-frequency information simultaneously and efficiently. The hierarchical structure of MDBM allows the deeper branch to gradually accumulate fine-grained local details under the contextual guidance of the shallower branch. We visualize this process using feature maps, and further demonstrate the rationality and effectiveness of this design using proposed novel Fourier spectral analysis methods. Moreover, our model exhibits more significant spectral differentiation between branches than existing branch networks. This suggests that MDBM reduces feature redundancy and offers a more effective method for integrating high- and low-frequency information. Extensive qualitative and quantitative evaluations on various datasets show that our model can generate structurally consistent and visually realistic HR images. It achieves state-of-the-art (SOTA) results at a very fast inference speed. Our code is available at https://github.com/thy960112/MDBN.
更多查看译文
关键词
network,multi-depth,super-resolution
AI 理解论文
溯源树
样例
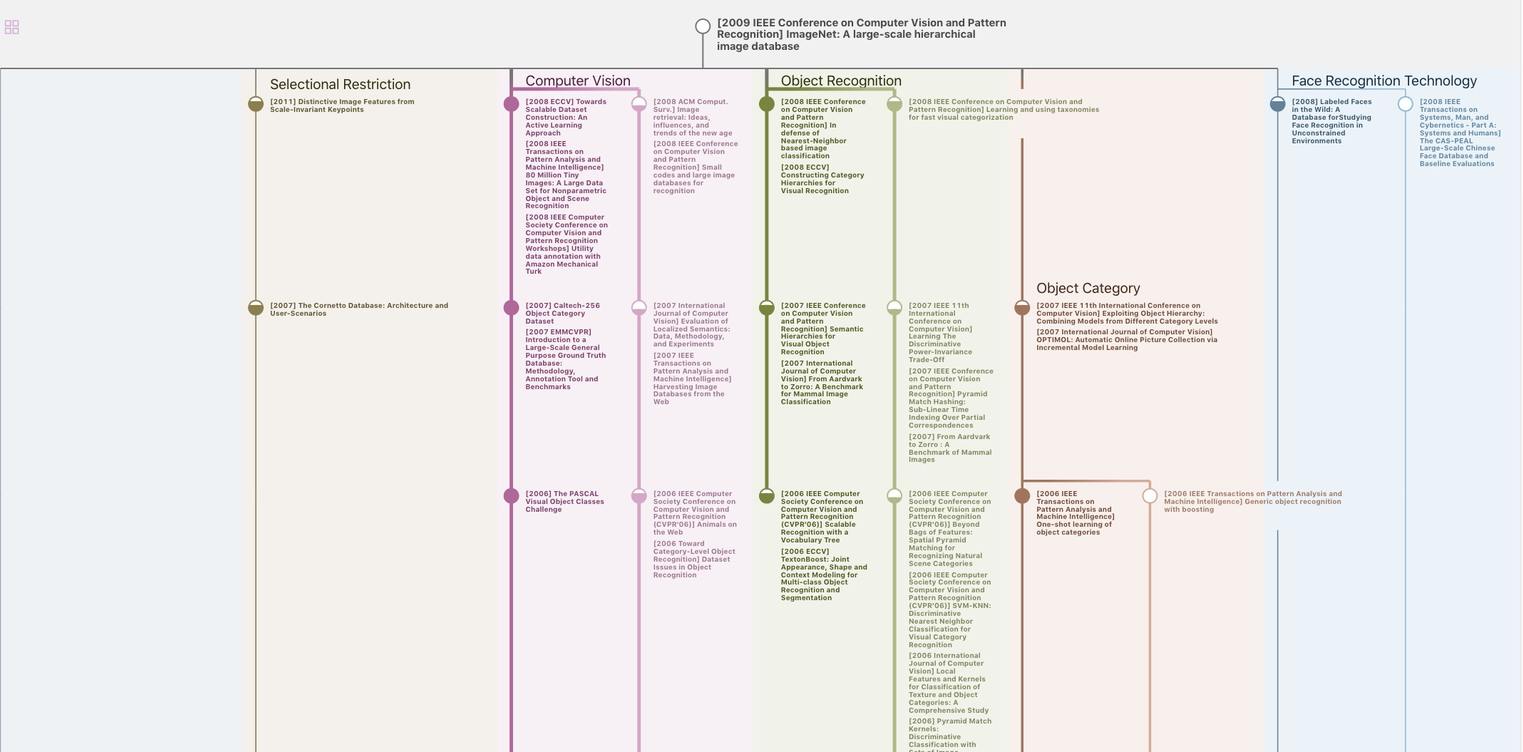
生成溯源树,研究论文发展脉络
Chat Paper
正在生成论文摘要