Data-driven localized waves and parameter discovery in the massive Thirring model via extended physics-informed neural networks with interface zones
Chaos, Solitons & Fractals(2023)
摘要
In this paper, we study data-driven localized wave solutions and parameter discovery in the massive Thirring (MT) model via the deep learning in the framework of physics-informed neural networks (PINNs) algorithm. Abundant data-driven solutions including soliton of bright/dark type, breather and rogue wave are simulated accurately and analyzed contrastively with relative and absolute errors. For higher-order localized wave solutions, we employ the extended PINNs (XPINNs) with domain decomposition to capture the complete pictures of dynamic behaviors such as soliton collisions, breather oscillations and rogue-wave superposition. In particular, we modify the interface line in domain decomposition of XPINNs into a small interface zone and introduce the pseudo initial, residual and gradient conditions as interface conditions linked adjacently with individual neural networks. Then this modified approach is applied successfully to various solutions ranging from bright-bright soliton, dark-dark soliton, dark-antidark soliton, general breather, Kuznetsov-Ma breather and second-order rogue wave. Experimental results show that this improved version of XPINNs reduce the complexity of computation with faster convergence rate and keep the quality of learned solutions with smoother stitching performance as well. For the inverse problems, the unknown coefficient parameters of linear and nonlinear terms in the MT model are identified accurately with and without noise by using the classical PINNs algorithm.
更多查看译文
关键词
thirring model,waves,neural networks,parameter discovery,data-driven,physics-informed
AI 理解论文
溯源树
样例
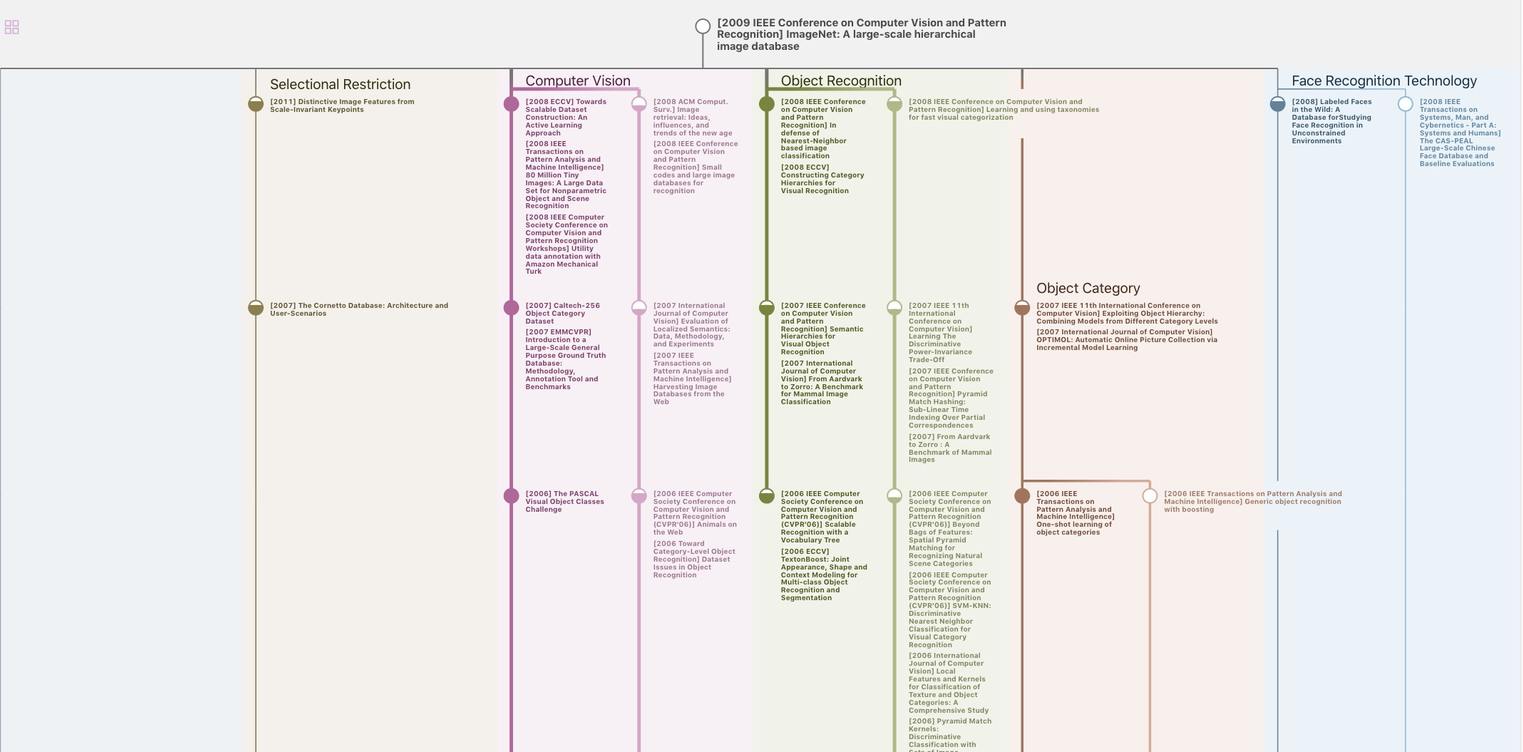
生成溯源树,研究论文发展脉络
Chat Paper
正在生成论文摘要