Scalable Neural Dynamic Equivalence for Power Systems
IEEE Access(2023)
摘要
Traditional grid analytics are model-based, relying strongly on accurate
models of power systems, especially the dynamic models of generators,
controllers, loads and other dynamic components. However, acquiring thorough
power system models can be impractical in real operation due to inaccessible
system parameters and privacy of consumers, which necessitate data-driven
dynamic equivalencing of unknown subsystems. Learning reliable dynamic
equivalent models for the external systems from SCADA and PMU data, however, is
a long-standing intractable problem in power system analysis due to complicated
nonlinearity and unforeseeable dynamic modes of power systems. This paper
advances a practical application of neural dynamic equivalence (NeuDyE) called
Driving Port NeuDyE (DP-NeuDyE), which exploits physics-informed machine
learning and neural-ordinary-differential-equations (ODE-NET) to discover a
dynamic equivalence of external power grids while preserving its dynamic
behaviors after disturbances. The new contributions are threefold: A NeuDyE
formulation to enable a continuous-time, data-driven dynamic equivalence of
power systems, saving the effort and expense of acquiring inaccessible system;
An introduction of a Physics-Informed NeuDyE learning (PI-NeuDyE) to actively
control the closed-loop accuracy of NeuDyE; and A DP-NeuDyE to reduce the
number of inputs required for the training. We conduct extensive case studies
on the NPCC system to validate the generalizability and accuracy of both
PI-NeuDyE and DP-NeuDyE, which span a multitude of scenarios, differing in the
time required for fault clearance, the specific fault locations, and the
limitations of data. Test results have demonstrated the scalability and
practicality of NeuDyE, showing its potential to be used in ISO and utility
control centers for online transient stability analysis and for planning
purposes.
更多查看译文
关键词
Neural dynamic equivalence,ODE-NET,physics-informed machine learning,model order reduction,driving port
AI 理解论文
溯源树
样例
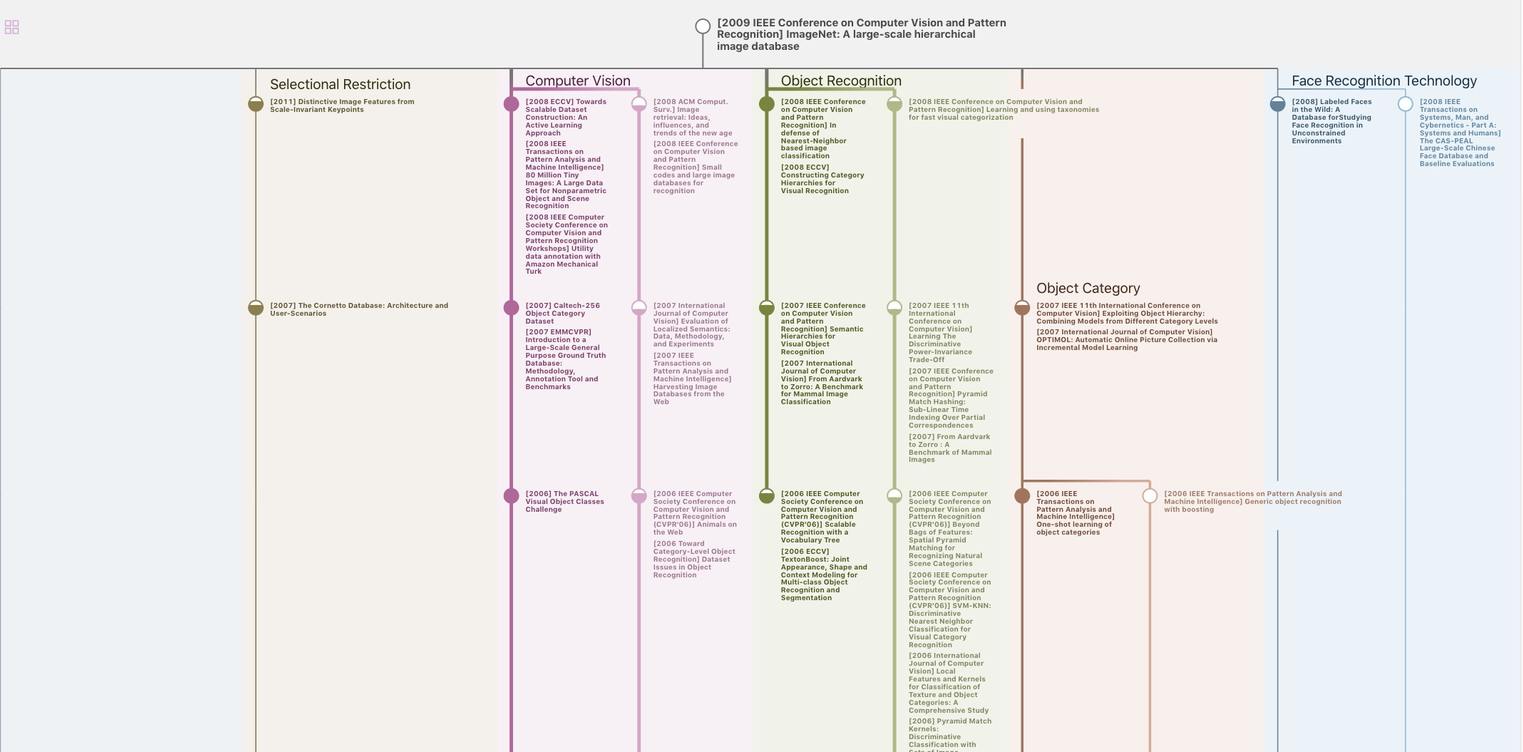
生成溯源树,研究论文发展脉络
Chat Paper
正在生成论文摘要