Physics-Aware Neural Dynamic Equivalence of Power Systems
IEEE TRANSACTIONS ON POWER SYSTEMS(2024)
Abstract
This letter devises Neural Dynamic Equivalence (NeuDyE), which explores physics-aware machine learning and neural-ordinary-differential-equations (ODE-Net) to discover a dynamic equivalence of external power grids while preserving its dynamic behaviors after disturbances. The contributions are threefold: 1) an ODE-Net-enabled NeuDyE formulation to enable a continuous-time, data-driven dynamic equivalence of power systems; 2) a physics-informed NeuDyE learning method (PI-NeuDyE) to actively control the closed-loop accuracy of NeuDyE without an additional verification module; 3) a physics-guided NeuDyE (PG-NeuDyE) to enhance the method's applicability even in the absence of analytical physics models. Extensive case studies in the NPCC system validate the efficacy of NeuDyE, and, in particular, its capability under various contingencies.
MoreTranslated text
Key words
Dynamic equivalence,ODE-Net,physics-informed machine learning,model order reduction
AI Read Science
Must-Reading Tree
Example
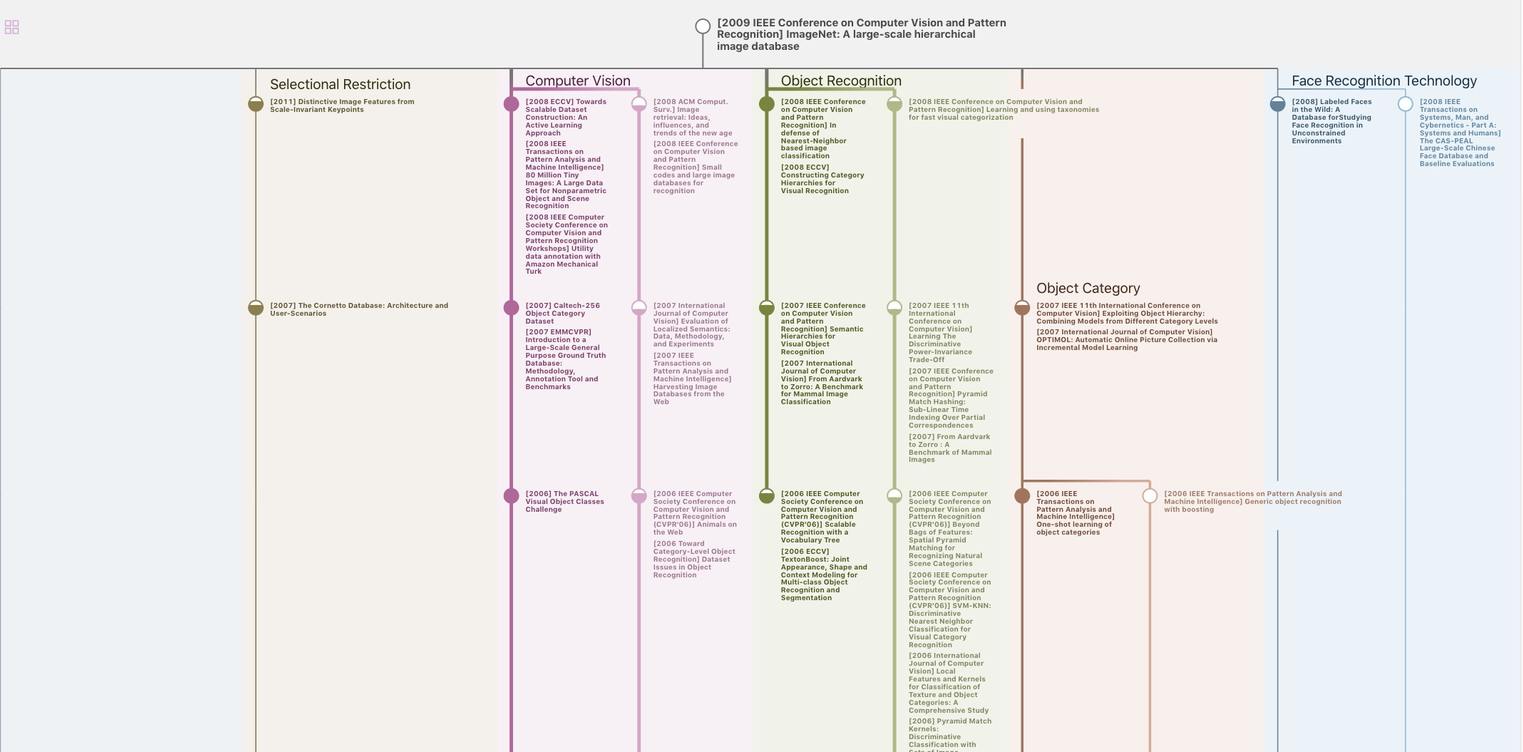
Generate MRT to find the research sequence of this paper
Chat Paper
Summary is being generated by the instructions you defined