Extension of Transformational Machine Learning: Classification Problems
CoRR(2023)
摘要
This study explores the application and performance of Transformational Machine Learning (TML) in drug discovery. TML, a meta learning algorithm, excels in exploiting common attributes across various domains, thus developing composite models that outperform conventional models. The drug discovery process, which is complex and time-consuming, can benefit greatly from the enhanced prediction accuracy, improved interpretability and greater generalizability provided by TML. We explore the efficacy of different machine learning classifiers, where no individual classifier exhibits distinct superiority, leading to the consideration of ensemble classifiers such as the Random Forest. Our findings show that TML outperforms base Machine Learning (ML) as the number of training datasets increases, due to its capacity to better approximate the correct hypothesis, overcome local optima, and expand the space of representable functions by combining separate classifiers capabilities. However, this superiority is relative to the resampling methods applied, with Near Miss demonstrating poorer performance due to noisy data, overlapping classes, and nonlinear class boundaries. Conversely, Random Over Sampling (ROS) provides a more robust performance given its resistance to noise and outliers, improved class overlap management, and suitability for nonlinear class boundaries.
更多查看译文
关键词
transformational machine learning,machine learning,classification,extension
AI 理解论文
溯源树
样例
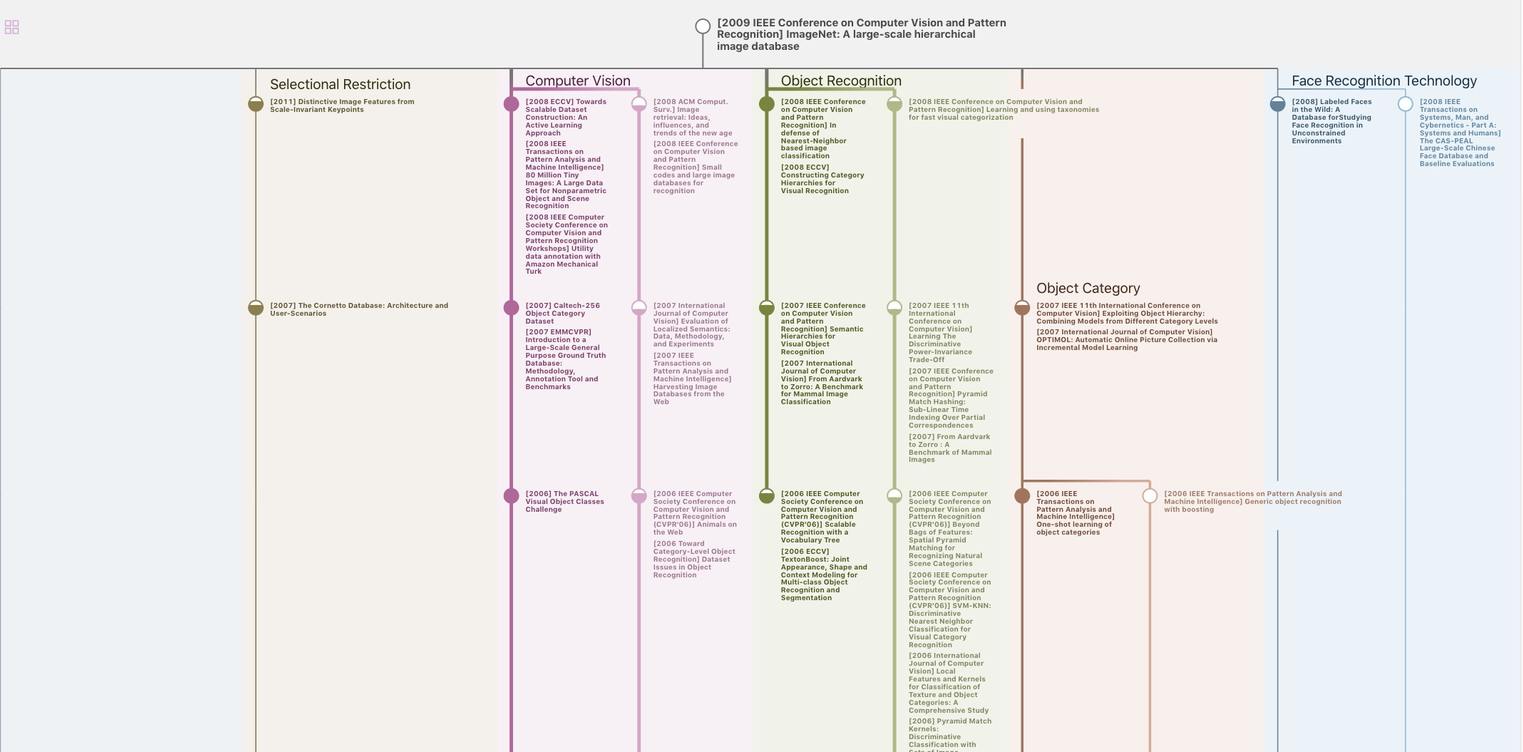
生成溯源树,研究论文发展脉络
Chat Paper
正在生成论文摘要