On the Mark: Modeling and Forecasting for Public Health Impact.
Health security(2023)
摘要
Health SecurityVol. 21, No. S1 CommentaryOpen AccessCreative Commons licenseOn the Mark: Modeling and Forecasting for Public Health ImpactMark J. Panaggio, Shelby N. Wilson, Jeremy D. Ratcliff, Luke C. Mullany, Jeffrey D. Freeman, and Kaitlin Rainwater-LovettMark J. PanaggioMark J. Panaggio, PhD, is Applied Mathematicians/Data Scientists, Johns Hopkins University Applied Physics.Search for more papers by this author, Shelby N. WilsonAddress correspondence to: Shelby N. Wilson, PhD, Applied Mathematician/Data Scientist, Johns Hopkins University Applied Physics Laboratory, 11100 Johns Hopkins Road, Laurel, MD 20723. E-mail Address: [email protected]Shelby N. Wilson, PhD, is Applied Mathematicians/Data Scientists, Johns Hopkins University Applied Physics.Search for more papers by this author, Jeremy D. RatcliffJeremy D. Ratcliff, PhD, is a Senior Scientist, Asymmetric Operations Sector, Johns Hopkins University Applied Physics.Search for more papers by this author, Luke C. MullanyLuke C. Mullany, PhD, MS, MHS, is a Senior Researcher, Research and Exploratory Development Department, Johns Hopkins University Applied Physics.Search for more papers by this author, Jeffrey D. FreemanJeffrey D. Freeman, PhD, MPH, is Director and Special Assistant to the President, National Center for Disaster Medicine and Public Health, Uniformed Services University of the Health Sciences, Bethesda, MD.Search for more papers by this author, and Kaitlin Rainwater-LovettKaitlin Rainwater-Lovett, PhD, MPH, is Assistant Program Manager, Johns Hopkins University Applied Physics Laboratory, Laurel, MD.Search for more papers by this authorPublished Online:27 Sep 2023https://doi.org/10.1089/hs.2023.0033AboutSectionsPDF/EPUB Permissions & CitationsPermissionsDownload CitationsTrack CitationsAdd to favorites Back To Publication ShareShare onFacebookTwitterLinked InRedditEmail IntroductionThe COVID-19 pandemic wrought significant health, political, and economic disruption globally. From the onset of the pandemic, governments, multinational agencies, and the public relied upon forecasts, or short-term trajectories of disease outcomes generated by mathematical models, to inform personal, political, and operational decisions. The models creating these forecasts are diverse and include assessments of past, current, and future effectiveness of nonpharmaceutical interventions on viral spread and hospitalizations,1-3 estimates of the reproductive number for emerging variants,4 quantification of outcomes (eg, number of COVID-19 cases, hospitalizations, deaths) over various time horizons, and determination of the utility of public health stay-at-home orders.5,6 For the US government, forecasts have informed decisions related to ventilator redistribution, priority populations for vaccination, resource allocation of personal protective equipment and therapeutics (eg, monoclonal antibodies) to healthcare facilities, and future demand of at-home COVID-19 tests.1,7,8 Citizens, perhaps unknowingly, have used forecasting throughout the pandemic to guide their own decisions, such as parents making predictions as to their child's risk of contracting COVID-19 if they did not wear a mask on an airplane. The COVID-19 pandemic has altered the landscape of public and government access to infectious disease data, ushering in new opportunities to expand epidemiological forecasting. Aligning these forecasts with the needs and interests of decisionmakers will increase their uptake and impact.Increased interest from researchers, decisionmakers, and the general public has highlighted the need for real-time forecasts that accurately describe current and future states of the pandemic. In response, the modeling community increased their focus on developing forecasts to provide key insights for public and government decisionmakers. Unfortunately, gaps in understanding between the modeling community and operational staff limited the impact of utility during the COVID-19 pandemic. Forecasts presented by the modeling community were often derived from complex models that were difficult to interpret for operational specialists and, especially at the beginning of the response, lacked the timeliness to inform real-time decisionmaking.8,9 For example, weekly outputs from the COVID-19 Forecast Hub10 did not always meet the needs of decisionmakers who often requested new outputs on the timescale of hours to days. To avoid increasing demands on the (largely volunteer) academic modeling community, new methods for evaluating and communicating outputs from diverse groups to decisionmakers need to be developed. While public health modeling had a substantial positive impact on the response to the COVID-19 pandemic, opportunities to reduce unnecessary morbidity and mortality were missed due to an inability to rapidly resolve and communicate the complex modeling landscape.In this commentary, we present a framework to facilitate the use of forecasting for operational decisionmaking within the context of public health. These insights are derived from our experience developing forecasts and models to inform health response activities during the COVID-19 pandemic as well as knowledge of their limitations and uptake by decisionmakers. These recommendations build on the EPIFORGE 2020 guidelines11 that assist modelers in communicating forecasting outputs.A Framework for Model OperationalizationForecasts are considered “operationalized” when they guide decisionmaking by leaders and/or the public over the course of a response. Ensuring that epidemiological forecasting and modeling can effectively inform public health response activities requires not only continued development of novel models and analytic methods, but also a framework within which these forecasts can be evaluated and communicated to decisionmakers. The process of operationalization involves modeling for accuracy with interpretable precision, understanding differences between ground truth and observed data, and more fully establishing the downstream effects of mitigations beyond the initial model outcomes.Extending the operational impact of forecasts will require technical advancements in 3 primary areas: Uncertainty estimation. All epidemiological forecasts are inherently uncertain, making measuring and comparing uncertainty critical for both high-level decisionmaking and the public's day-to-day choices. Existing methods for describing model uncertainty combine multiple sources of uncertainty into a single range of outcomes that is often too wide to inform decisionmaking. Methods that account for and quantify disparate sources of uncertainty are needed to determine the limitations of existing data collection and modeling pipelines, guide focused efforts to reduce uncertainty, and provide insight into how different scenarios relate to outcomes.Verification and validation (V&V). Thorough V&V of models is needed to build trust with decisionmakers; models that produce preposterous forecasts or drive poor decisionmaking harm the uptake of other, valid models by decreasing trust of the modeling domain at large. A path forward would include independent evaluations of the underlying algorithms and ongoing comparisons of previous model forecasts and observed outcomes. The results of these evaluations must be easily accessible to decisionmakers to enable them to determine the conditions under which models can and should be trusted.Visualization and communication. Forecasts must be available in a useful and interpretable format for the layperson. Dynamic, interactive visualizations are a type of communication tool that supports a deeper understanding of forecasted uncertainty and outputs and may provide appropriate context related to spatial and temporal trends. Forecasts must also be accompanied by documentation of assumptions, limitations, and past performance to ensure that the forecasts are interpreted properly.11Uncertainty EstimationDuring a crisis, access to timely and reliable data, models, and forecasts is critical to prioritize decisions and resources. However, these forecasts and the underlying data and models are inherently uncertain. Uncertainty accumulates during every step of the transformation of model inputs into a forecasted outcome (Figure 1). A proper understanding of this uncertainty is necessary for optimal decisionmaking; when uncertainty is high, forecast consumers may want to develop contingency plans. During the COVID-19 pandemic, the utility of forecasting models was hindered by a high degree of uncertainty and representations of uncertainty that decisionmakers found difficult to interpret and use. Novel methods for reducing, estimating, representing, and communicating uncertainty are needed to ensure that models can be understood, trusted, and leveraged effectively.Figure 1. Potential origins of uncertainty in data collection, modeling, and forecasting of disease.Indicators of disease burden—such as reported cases, hospitalizations, and deaths—are vital for tracking the spread of disease and characterizing burden. However, each of these indicators is subject to sampling biases, latency, and missingness, which can provide an incomplete and inaccurate picture of the current state. These biases stem from a variety of factors, including nonuniformity in testing and reporting rates, data entry errors, missing data, delayed reporting, and aggregation of line-level data that obscures differences across relevant subpopulations.12-14 Attempts to correct for these biases require characterization of the sources and magnitude of biases so that one can estimate the true disease burden from imperfect indicators. While these corrections can reduce bias, they introduce uncertainty into model inputs that is thus appropriately reflected in the resulting forecasts. Although uncertainty may appear undesirable, an accurate accounting for potential sources of bias and variability will provide a better foundation for operational decisions and can inform efforts to address uncertainty at its source.Current modeling efforts represent uncertainty using predictive intervals that indicate ranges, which are expected to include the observed outcome within a sampling frequency (most commonly 50% or 95%). Selecting an appropriate interval width is a balancing act between precision and accuracy. While narrow intervals may be attractive from a visualization perspective, they reduce decisionmaker trust in predictive models if the subsequent realized true value falls outside of the interval bounds. Wide intervals are more likely to contain the true outcome, but they may fail to convey actionable information to decisionmakers.During the COVID-19 pandemic, the intervals presented with forecasts were too wide to facilitate effective decisionmaking, particularly below the state level. For this reason, timely models that generate narrower ranges of uncertainty tied to specific, interpretable, and relevant scenarios are needed to better assess the impact of proposed interventions and factors driving uncertainty.Specific to infectious disease forecasting, there are many ways uncertainty might be reduced in both the data and model methods, such as:Improving estimates of ground-truth infection rates by obtaining more reliable and frequent estimates of the pathogen attack rate. An example are the well-coordinated cross-sectional seroprevalence surveys that evaluate the ratio between reported infections and true infections conducted by the Office for National Statistics in the United Kingdom at an expense of £390 million annually15,16Reducing noise, missingness, and latency in reporting by improving data collection and dissemination tools and technologies available to state and local health departments. This could include the US Centers for Disease Control and Prevention (CDC) ReportStream17 and the proposed Front Door platform18 to reduce jurisdictional reporting burden and expedite data transfer from nongovernment entities (eg, commercial testing laboratories).Developing techniques to improve the selection of model type (eg, mechanistic, statistical, machine learning) based on model outcome to more accurately reflect the uncertainty associated with input data (eg, assessing uncertainty of model selection via bootstrapping methods19).Leveraging additional data sources and types to provide corroborating signals of disease spread (eg, wastewater monitoring, self-reported symptom tracking20).The relevant contributions of each of these components to cumulative forecasted uncertainty are unknown and may be model- and context-specific. Methods for targeting the root causes of uncertainty are needed to quantify the impacts of each source along with the benefits of addressing each limitation. This would likely produce narrower uncertainty intervals relevant to specific scenarios and/or sets of assumptions, such as those demonstrated in the activities of the COVID-19 Scenario Modeling Hub.3,5,6,15,21 Such an analysis would also enable identification of the aspects of existing health surveillance systems that contribute to uncertainty. Targeted development in this area, guided by forecasting and modeling experts, may significantly improve future forecast performance.Lastly, novel methods for representing and communicating uncertainty are needed to address the questions of interest to policymakers. Current modeling efforts focus on counts of cases, hospitalizations, and deaths as their primary targets. However, the timing and magnitudes of peaks, the likelihood of secondary waves, and variations in growth rates and arrival times22 are also important targets. When model outputs are represented using predictive intervals rather than individual trajectories, vital information is obscured, making it more difficult to assess these targets. For this reason, alternative methods for interrogating uncertain forecasts are needed to ensure that all outcomes of interest can be captured.Verification and ValidationForecast-generating models must undergo thorough V&V to ensure the forecasts are trustworthy and that resultant decisions are defensible. COVID-19 brought together teams traditionally involved in infectious disease modeling as well as experts from other domains, such as computational statistics, physics, software development, and finance.7 Each team brought techniques and assumptions from their domain into infectious disease forecasting, leading to a significant increase in the number of novel models used and developed. While the models were innovative and potentially overcame biases in the methods typically used in the field, many were developed rapidly and lacked independent V&V. In other instances, particularly early in the pandemic, several models from influential researchers drove media headlines but rapidly became incongruent with real-world experience, substantially diminishing public and governmental perception of the utility of forecasts.23,24Several factors have contributed to this initial dearth of V&V, including (1) the lack of standardized tools and methods to provide direction as to which existing V&V methods should be used and which novel methods needed to be developed and (2) the lack of feasibility of thoroughly performing V&V. The latter issue is exacerbated for emerging pathogens for which there are insufficient past outcomes to compare with current and past forecasts, as noted elsewhere.25 As a result, decisionmakers were left with little choice but to rely on unvalidated models, some of which proved to be unreliable and thus discouraged decisionmakers from further using resulting forecasts.Although significant progress in the development and use of V&V has occurred, current efforts remain decentralized. Many model performance results are not made public, and those that are publicly available can be difficult to interpret due to a lack of standardization. The most comprehensive example of consistent V&V currently available is provided by the COVID-19 Forecast Hub, which provides a repository of past forecasts and monthly performance evaluation reports.26 While this resource provides a useful starting point for further V&V development, application, and evaluation of models, more can be done to adequately vet models and ensure that validation results are accessible to support critical decisions. Additional development and application of V&V should include:Implementing items 10 (describe and justify the validation approach), 11 (describe and justify the accuracy validation approach), and 12 (describe and justify the approach to benchmarking/comparison) of the EPIFORGE 2020 guidelines11Expanding V&V efforts to address models and data in addition to short-term forecasting performanceAssembling a collection of trusted models that have undergone a more comprehensive evaluation processDeveloping novel methods for describing qualitative aspects of model performanceDeveloping additional tools to make V&V results more accessible and interpretable to end usersV&V should extend beyond short-term performance evaluation. As noted elsewhere, models that perform well during one phase of an epidemic often fail to generalize to other phases, making short-term performance an unreliable indicator of trustworthiness.7 For example, during the early stages of an epidemic, exponential or logistic growth models can yield highly accurate predictions.27 However, these models are mathematically incapable of predicting secondary waves and their accuracy deteriorates after a peak is reached. Independent verification of the structure and assumptions of models can ensure that model interpretation and usage are confined to their domain of validity.Ensemble forecasts, which combine the forecasts from a collection of component models to generate a consensus prediction, are the current state of the art for epidemiological forecasting.28 Within the COVID-19 Forecast Hub, ensemble models based on all submitted forecasts were among the best performers available for predicting incident COVID-19 deaths; similar ensemble performance was noted in the 2015-2016 Zika outbreak in Colombia.7,28 However, the COVID-19 Forecast Hub applied minimal checks related to completeness and consistency with recent data before individual forecasts were incorporated into ensemble models and shared with the public. Although ensembles generally benefit from a diversity of independent estimates produced by a variety of approaches and assumptions,28 the inclusion of poorly performing models that have not been adequately validated can decrease overall performance, particularly when weights are assigned equally. For example, within the COVID-19 Forecast Hub,10 an ensemble model trained on individual model performance did outperform an untrained method for forecasting COVID-19 deaths but not cases.29Future ensemble modeling projects could proceed with 2 cohorts, each with an associated ensemble: (1) open submission with minimal requirements to encourage wide participation and obtain a diverse set of forecasts, and (2) a curated subset of models that undergo a more comprehensive V&V process and can be used for defensible decisionmaking. These trusted models should undergo rigorous V&V, addressing multiple facets of forecast generation, including input data quality, model architecture, fitting methods, and continuous evaluation of performance. This process should involve collecting documentation about each aspect of the model from the inputs used to the assumptions and limitations, as recommended in item 8 of the EPIFORGE 2020 guidelines.11 Testing with synthetic or historical data could ensure that models provide reasonable and accurate forecasts under conditions where the ground truth is known. This helps ensure a collection of high-quality, thoroughly vetted models are available to inform critical decisions. As individual model performance is variable over time, maintaining a of models considered “trusted” would be an ongoing process.7,28Performance results for both cohorts of models should be generated on a rolling basis and made readily available alongside the forecast results. These results should include existing metrics—such as mean absolute error, coverage rate, and weighted intervals scores—as well as novel metrics for describing qualitative behavior, such as the ability to predict rates of change and peak timing. This will require standardizing ground-truth data sources, designing new evaluation metrics, and reassessing the way forecasts are collected to ensure that these metrics can be computed using the available data. Although existing tools, such as the Forecast Evaluation Dashboard developed by the Carnegie Mellon University Delphi Group,30 provide some performance metrics and visualization options for model evaluation, these options are limited and the results are not linked to visualizations of the forecasts themselves. As a result, considerable effort is required to access validation results relevant to interpreting particular forecasts. Without easy access to reliable V&V results, distinguishing high- versus low-performing models can be difficult. This can cause forecasting failures from low-performing models that contribute to diminished trust in all models. The creation of a centralized and standardized hub for V&V linked to visualization tools would improve both the reliability and accessibility of V&V results and ensure that decisionmakers are able to better determine which models to use, when they can be trusted, and how the forecasts should be interpreted.Visualization and CommunicationThe end goals of forecasting activities are multifaceted and complex. Therefore, the tools designed both to visualize and communicate model results must meet the requirements of end users. Defining a specific user base allows for the development of tools targeted to support specific user needs. Potential user groups include public health officials, government representatives, and the general public. The intended audience should influence the scientific complexity of the forecast visualization.31A number of visualization modes can communicate relevant metrics of current and future states of an ongoing public health event, as displayed in the healthdata.gov COVID-19 Community Profile Report.32 These include the following:Time series to provide detailed views of trends (Figure 2);Geographic maps to highlight spatial patterns (Figure 3);Point plots with prediction intervals to compare values and uncertainties (Figure 4);Geofacets to compare trends across regions (Figure 5); andRankings to highlight concerning areas or trends.Figure 2. US Centers for Disease Control and Prevention ensemble forecast time series plot of reported hospital admissions from April 12 through June 13, 2022 (black) and forecasted hospital admissions from June 21 to July 15, 2022 (red).33Figure 3. Community Profile Report 7-day average of reported hospital admissions per 100,000 population by county from June 13 to June 19, 2022.32Figure 4. Ranked point plot of cumulative confirmed COVID-19 hospital admissions per inpatient bed from June 23 to July 13, 2022 (top 20 states shown), estimated by Johns Hopkins Applied Physics Laboratory Gecko-SARIMA model. Abbreviations: CI, confidence interval; SARIMA, Seasonal Auto-Regressive Integrated Moving Average.Figure 5. Ensemble forecast geofaceted map of reported hospital admissions from April 26 to June 20, 2022 and forecasted hospital admissions from June 22 to July 10, 2022.32These visualization modes were drawn from end users' insights to enable situational awareness for decisionmakers in network security.34 A key element in leveraging such visualizations is development of a suite of flexible tools that allow users to access metrics and/or forecasts at the desired geographic resolution of interest with appropriate context to facilitate situational awareness, communication of forecast limitations, and data-driven decisionmaking.With both an established user base as well as a suite of dynamic visualizations, a targeted user interface can be developed. User-defined visualizations can be in the form of both static reports and dynamic interactive tools.Static reports, such as those delivered in the Community Profile Report,32 could be designed for use at both the national and subnational geographic scales. Additionally, dynamic, interactive tools can be developed to allow users to explore both the current predictive capabilities of verified and validated models as well as the contribution of each individual model to an ensembled prediction. Such dynamic tools were initiated during the COVID-19 pandemic, including through the COVID-19 Scenario Modeling Hub,35 but they require additional scaling in computational power and interactive options to create user-friendly dynamic interactions. These tools would allow users to visualize a comparison of particular scenarios, such as the effects of providing a predetermined set of resources over selected locations or demographics within prespecified time periods.36,37Key to providing an operational tool to users is thorough documentation and training on how to use and interpret the output of predictive forecasts (similar to item 14 of the EPIFORGE 2020 guidelines).11 While such understanding is common among the modeling community, particular effort must be placed into translating this understanding to nonexperts. Increased independent review of trusted models, standardization of predictive forecasts, and thorough and well-vetted documentation will lead to greater confidence among decisionmakers when leveraging forecasts.The modeling community remains focused on estimating future values of key metrics; however, their forecasts were underutilized to support policy decisions during the COVID-19 pandemic.8 Genuine partnerships must be developed between modelers and key decisionmakers to determine forecasting scenarios of interest, define model requirements, and identify the appropriate context for interpreting model results. Relevant questions to help determine requirements and context include the following:Are there clear guidelines for what options are available for decisionmakers?What levels of certainty are necessary to make a particular decision?What elements of forecasts are necessary to make a particular decision?Under what circumstances should forecast results be interpreted with caution?What are the consequences of inaccurate forecasts?What elements of forecasting efforts should be communicated to the public?Established requirements combined with clear visualizations will permit forecast use by local decisionmakers without overwhelming complex graphics, technical jargon, or models that are inappropriate for their jurisdiction. Clear and accessible forecasts also allow decisionmakers to more easily identify models that are best suited for their needs.A Platform for Improving OperationalizationSuccessfully operationalizing the analytic work of modelers requires more than collecting infectious disease forecasting models. Decisionmakers need to have confidence in the models and accuracy of forecasts; if trust cannot be established, even the best forecasts are unlikely to be informative and impactful. Opaque, highly complex models are unlikely to be used or trusted if they cannot be understood and validated. While improving disease surveillance and data collection methods will enhance model inputs, integrating forecast hubs with evaluation platforms and expanding user-centered exploration capabilities will make forecasts more accessible. The ability to visualize, interact, and customize forecasts facilitates increased understanding and communication. In turn, understanding what decisionmakers need to know and the precision required of forecasts would provide focused targets for model and scenario development in the context of the decisions required during an epidemic response.Developing an integrated and interactive V&V and visualization platform can directly address these needs. Such a platform should allow modelers to submit their models, documentation, and forecasts for independent V&V, while making forecasts more accessible and interpretable. Through the presentation of both current and historical forecasts along with the associated uncertainty in a flexible visualization interface, users can easily retrieve forecasts relevant to the locations of interest along with the context needed to interpret those forecasts properly. This platform should include the ability to:Simultaneously view forecasts for multiple indicators of disease burden for a single locationCompare forecasts across multiple locations side by sideView historical forecasts associated with a given model alongside the observed outcomesAccess model documentation and performance results directly from a forecastGenerate customizable reports that allow users to quickly access the forecasts they need in a reproducible wayAlthough previous efforts, such as a Forecast Evaluation Dashboard developed by the Carnegie Mellon University Delphi Group30 and the COVID-19 Forecast Hub,10 have addressed some of these needs, further unification is needed to provide the level of accessibility, dependability, and trustworthiness required to fully leverage the potential impact of models to improve response capabilities.The focus of the novel platform should be to establish robust methods of model V&V for existing and emerging threats and enable decisionmaking through improved articulation and visualization of uncertainty, especially in the context of practical considerations and tradeoffs.Closing ThoughtsOperational models with actionable forecasts have the potential to provide significant benefit to response efforts for current and ongoing public health emergencies. The COVID-19 pandemic highlighted several obstacles limiting the use of forecasts to support public health activities. To address these challenges, modeling and foreca
更多查看译文
关键词
COVID-19, Infectious disease forecasting, Uncertainty, Modeling, Public health decisionmaking
AI 理解论文
溯源树
样例
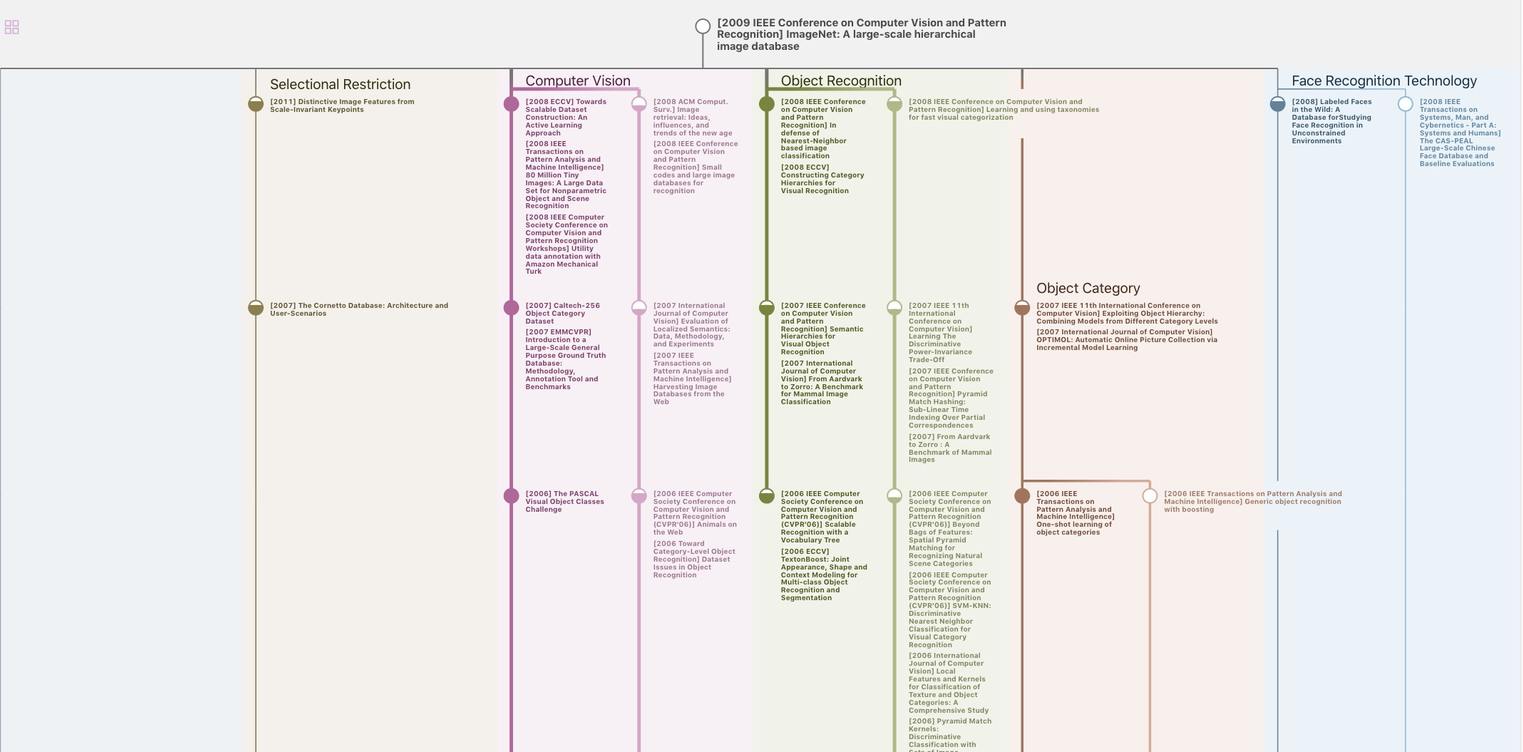
生成溯源树,研究论文发展脉络
Chat Paper
正在生成论文摘要