FORB: A Flat Object Retrieval Benchmark for Universal Image Embedding
arXiv (Cornell University)(2023)
摘要
Image retrieval is a fundamental task in computer vision. Despite recent advances in this field, many techniques have been evaluated on a limited number of domains, with a small number of instance categories. Notably, most existing works only consider domains like 3D landmarks, making it difficult to generalize the conclusions made by these works to other domains, e.g., logo and other 2D flat objects. To bridge this gap, we introduce a new dataset for benchmarking visual search methods on flat images with diverse patterns. Our flat object retrieval benchmark (FORB) supplements the commonly adopted 3D object domain, and more importantly, it serves as a testbed for assessing the image embedding quality on out-of-distribution domains. In this benchmark we investigate the retrieval accuracy of representative methods in terms of candidate ranks, as well as matching score margin, a viewpoint which is largely ignored by many works. Our experiments not only highlight the challenges and rich heterogeneity of FORB, but also reveal the hidden properties of different retrieval strategies. The proposed benchmark is a growing project and we expect to expand in both quantity and variety of objects. The dataset and supporting codes are available at https://github.com/pxiangwu/FORB/.
更多查看译文
关键词
flat object retrieval benchmark,universal image
AI 理解论文
溯源树
样例
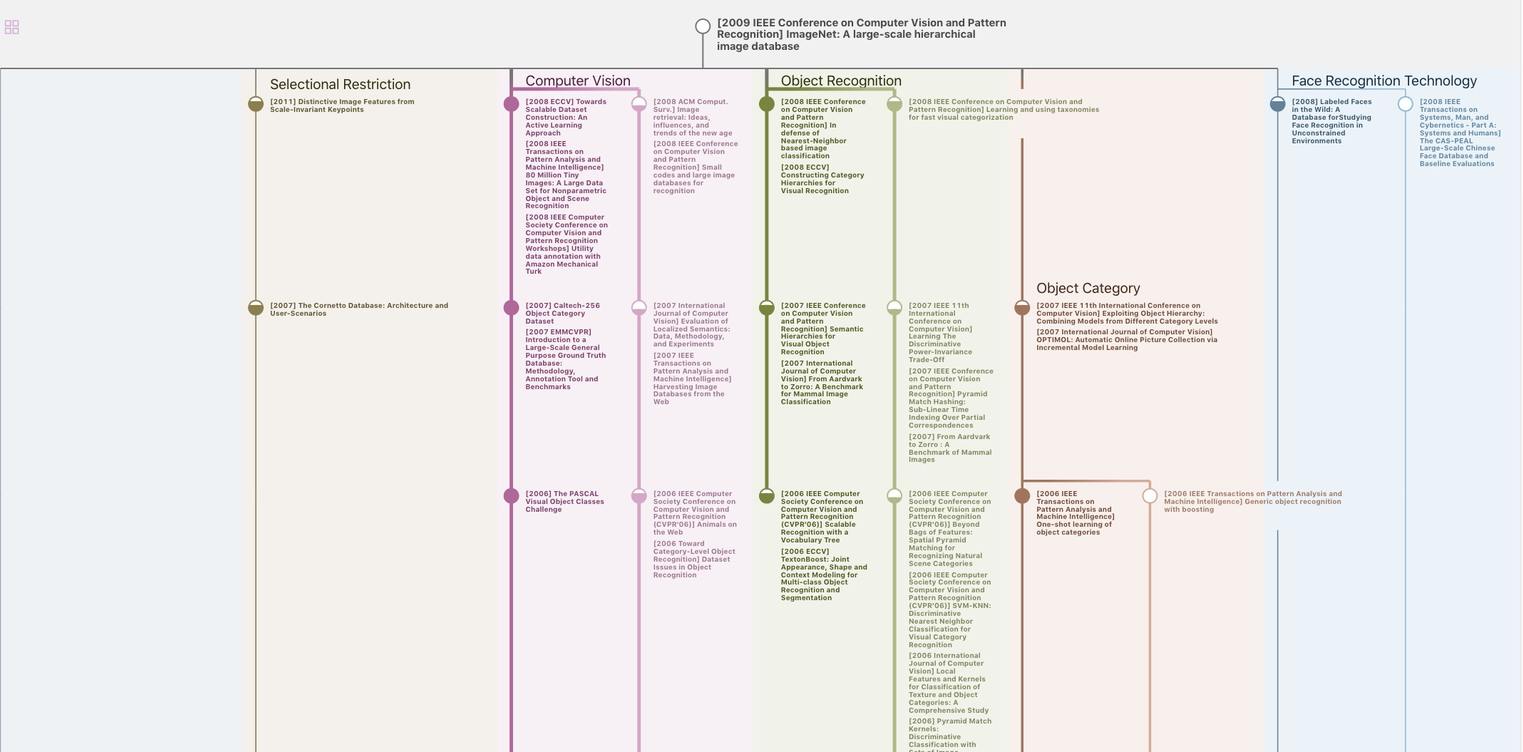
生成溯源树,研究论文发展脉络
Chat Paper
正在生成论文摘要