D$^3$Fields: Dynamic 3D Descriptor Fields for Zero-Shot Generalizable Robotic Manipulation
CoRR(2023)
摘要
Scene representation has been a crucial design choice in robotic manipulation systems. An ideal representation should be 3D, dynamic, and semantic to meet the demands of diverse manipulation tasks. However, previous works often lack all three properties simultaneously. In this work, we introduce D$^3$Fields - dynamic 3D descriptor fields. These fields capture the dynamics of the underlying 3D environment and encode both semantic features and instance masks. Specifically, we project arbitrary 3D points in the workspace onto multi-view 2D visual observations and interpolate features derived from foundational models. The resulting fused descriptor fields allow for flexible goal specifications using 2D images with varied contexts, styles, and instances. To evaluate the effectiveness of these descriptor fields, we apply our representation to a wide range of robotic manipulation tasks in a zero-shot manner. Through extensive evaluation in both real-world scenarios and simulations, we demonstrate that D$^3$Fields are both generalizable and effective for zero-shot robotic manipulation tasks. In quantitative comparisons with state-of-the-art dense descriptors, such as Dense Object Nets and DINO, D$^3$Fields exhibit significantly better generalization abilities and manipulation accuracy.
更多查看译文
关键词
dynamic 3d descriptor d$^3$fields,manipulation,zero-shot
AI 理解论文
溯源树
样例
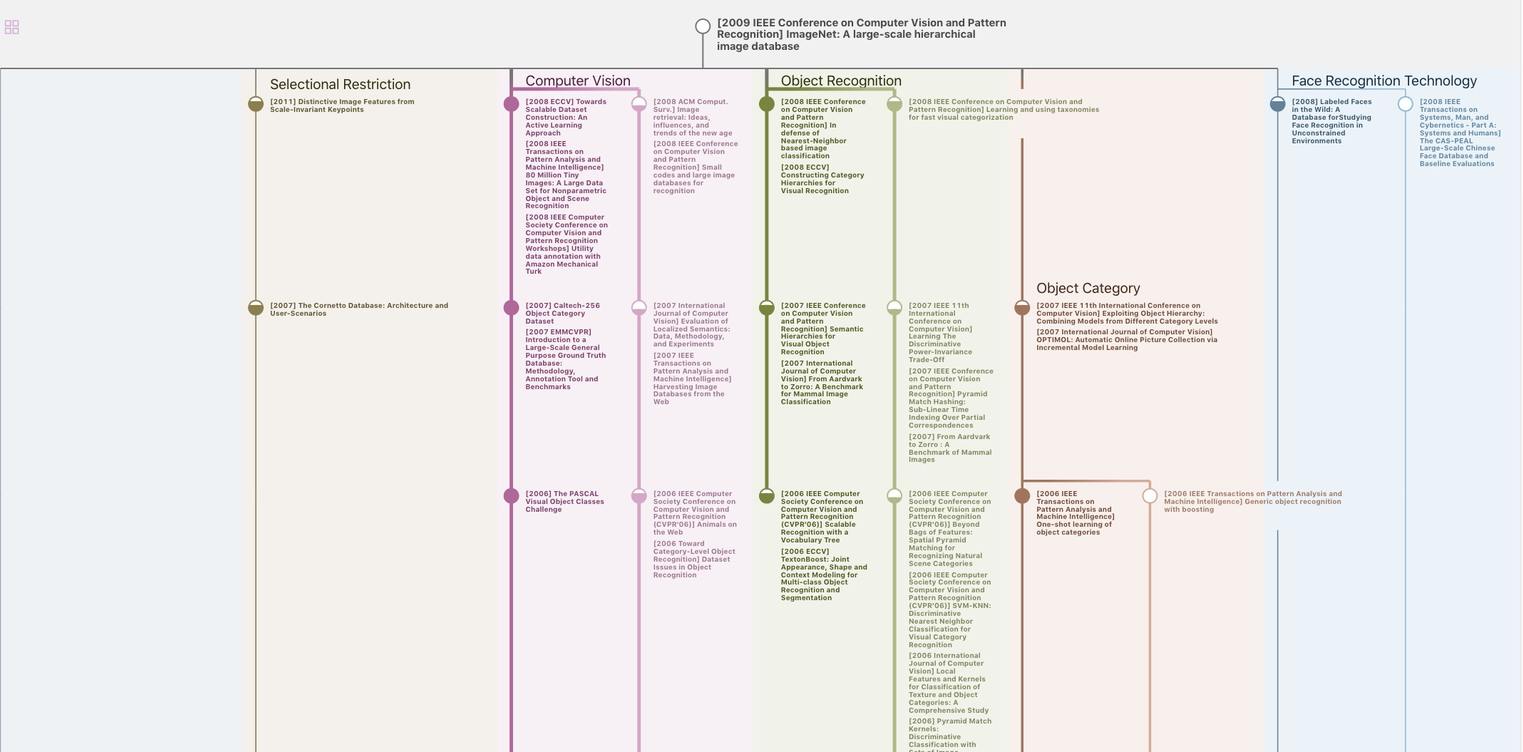
生成溯源树,研究论文发展脉络
Chat Paper
正在生成论文摘要