Masked autoencoders are scalable learners of cellular morphology
CoRR(2023)
Abstract
Inferring biological relationships from cellular phenotypes in high-content microscopy screens provides significant opportunity and challenge in biological research. Prior results have shown that deep vision models can capture biological signal better than hand-crafted features. This work explores how weakly supervised and self-supervised deep learning approaches scale when training larger models on larger datasets. Our results show that both CNN- and ViT-based masked autoencoders significantly outperform weakly supervised models. At the high-end of our scale, a ViT-L/8 trained on over 3.5-billion unique crops sampled from 95-million microscopy images achieves relative improvements as high as 28% over our best weakly supervised models at inferring known biological relationships curated from public databases.
MoreTranslated text
Key words
masked autoencoders,cellular morphology,scalable learners
AI Read Science
Must-Reading Tree
Example
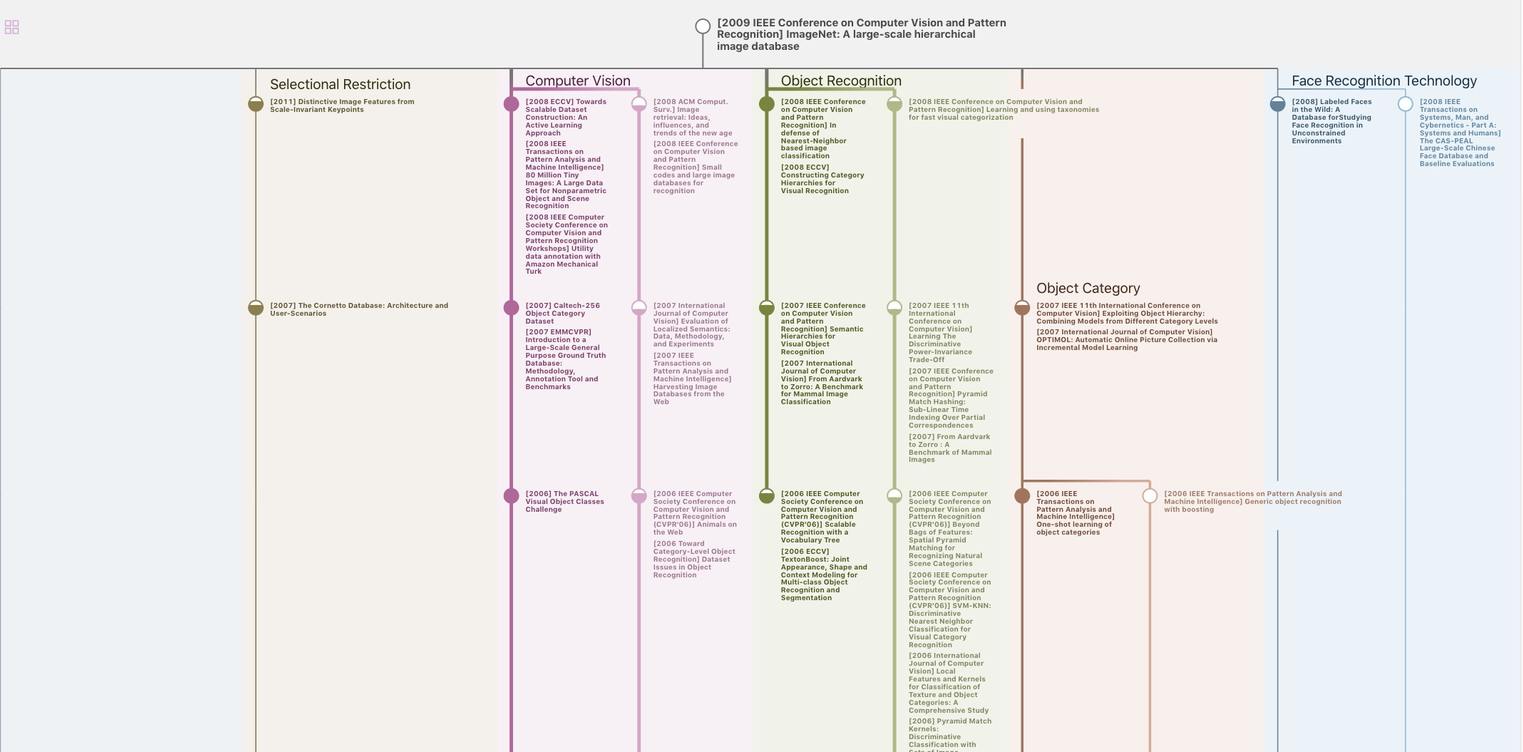
Generate MRT to find the research sequence of this paper
Chat Paper
Summary is being generated by the instructions you defined