Mechanical Properties of Single and Polycrystalline Solids from Machine Learning
ADVANCED THEORY AND SIMULATIONS(2024)
摘要
Calculating the elastic and mechanical characteristics of non-crystalline solids can be challenging due to the high computational cost of ab initio methods and the low accuracy of empirical potentials. This paper proposes a computational technique for efficient calculations of mechanical properties of polycrystals, composites, and multi-phase systems from atomistic simulations with high accuracy and reasonable computational cost. The calculated elastic moduli of polycrystalline diamond and their dependence on grain size are determined using a developed approach based on actively learned machine learning interatomic potentials (MLIPs). These potentials are trained on local fragments of the polycrystalline system, and ab initio calculations are used to compute forces, stresses, and energies. This technique allows researchers to perform extensive calculations of the mechanical properties of complex solids with different compositions and structures, achieving high accuracy and facilitating the transition from ideal (single crystal) systems to more realistic ones. Machine learning interatomic potentials based on moment tensor potentials are used to calculate the mechanical properties of single and polycrystalline diamond. The potentials are fitted on the fly to quantum mechanical calculations of local fragments (environments) of the large polycrystallines. This methodology is tested by calculating elastic moduli as a function of grain size of polycrystallines. image
更多查看译文
关键词
machine learning,moment tensor potentials,polycrystalline materials
AI 理解论文
溯源树
样例
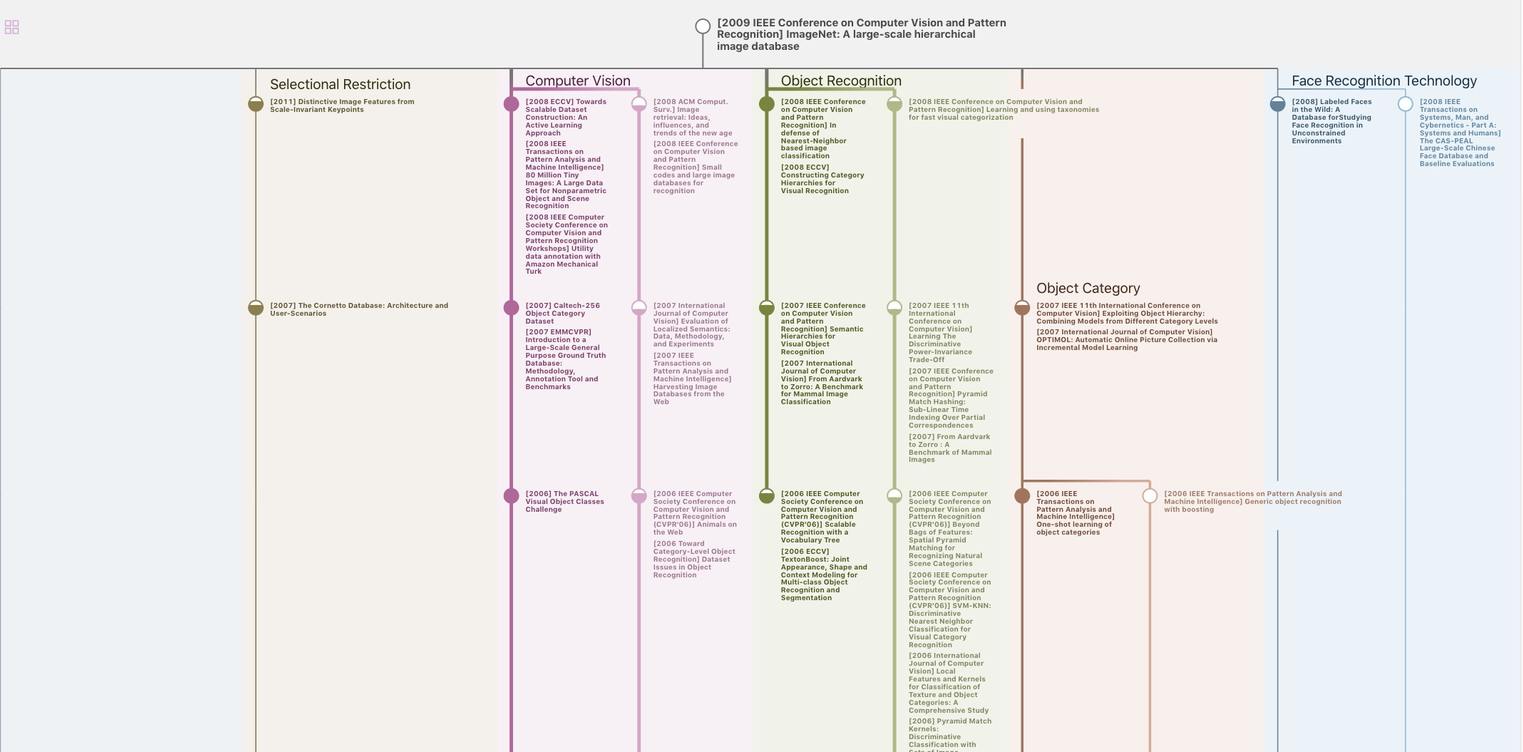
生成溯源树,研究论文发展脉络
Chat Paper
正在生成论文摘要