Self-Supervised Learning for Fusion of IR and RGB Images in Visual Teach and Repeat Navigation
2023 European Conference on Mobile Robots (ECMR)(2023)
摘要
With increasing computation power, longer battery life and lower prices, mobile robots are becoming a viable option for many applications. When the application requires long-term autonomy in an uncontrolled environment, it is necessary to equip the robot with a navigation system robust to environmental changes. Visual Teach and Repeat (VT&R) is one such navigation system that is lightweight and easy to use. Similarly, as other methods rely on camera input, the performance of VT&R can be highly influenced by changes in the scene's appearance. One way to address this problem is to use machine learning or/and add redundancy to the sensory input. However, it is usually complicated to collect long-term datasets for given sensory input, which can be exploited by machine learning methods to extract knowledge about possible changes in the environment from the data. In this paper, we show that we can use a dataset not containing the environmental changes to train a model processing infrared images and improve the robustness of the VT&R framework by fusion with the classic method based on RGB images. In particular, our experiments show that the proposed training scheme and fusion method can alleviate the problems arising from adverse illumination changes. Our approach can broaden the scope of possible VT&R applications that require deployment in environments with significant illumination changes.
更多查看译文
AI 理解论文
溯源树
样例
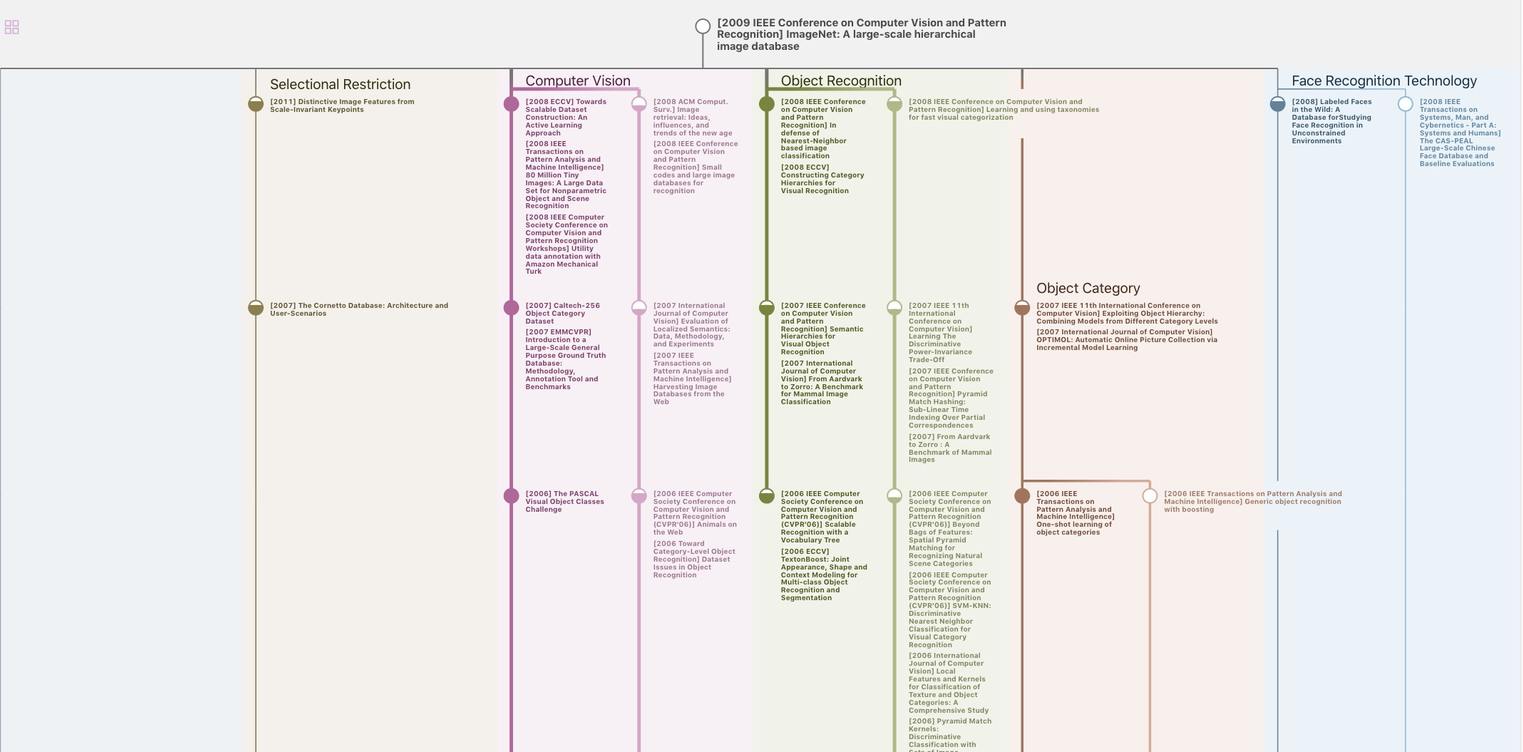
生成溯源树,研究论文发展脉络
Chat Paper
正在生成论文摘要