PPFLHE: A privacy-preserving federated learning scheme with homomorphic encryption for healthcare data
APPLIED SOFT COMPUTING(2023)
Abstract
Healthcare data are characterized by explosive growth and value, which is the private data of patients, and its characteristics and storage environment have brought significant issues of data privacy and security. People are reluctant to share their data for privacy concerns during machine learning. To balance this contradiction, Federated Learning was proposed as a solution to train on private data without sharing it. However, many studies show that there is still the possibility of privacy leakage during the training process of federated learning. In light of this, we propose a privacy-preserving federated learning scheme with homomorphic encryption(PPFLHE). Specifically, on the client side, homomorphic encryption technology is used to encrypt the training model shared by users to ensure its security and privacy. In addition, to prevent internal attacks, Access Control (AC) technology is used to confirm the user's identity and judge whether it is trusted; on the server side, the Acknowledgment (ACK) mechanism is designed to remove the dropped or unresponsive users temporarily, which reduces the waiting delay and communication overhead, and solves the problem of user's exiting during training. Theoretical analysis and experimental results show that the proposed scheme achieves high data utility and classification accuracy (81.53%), and low communication delay while achieving privacy preserving, compared to state-of-the-art methods.(c) 2023 Elsevier B.V. All rights reserved.
MoreTranslated text
Key words
Federated learning,Homomorphic encryption,Privacy -preserving,Healthcare data
AI Read Science
Must-Reading Tree
Example
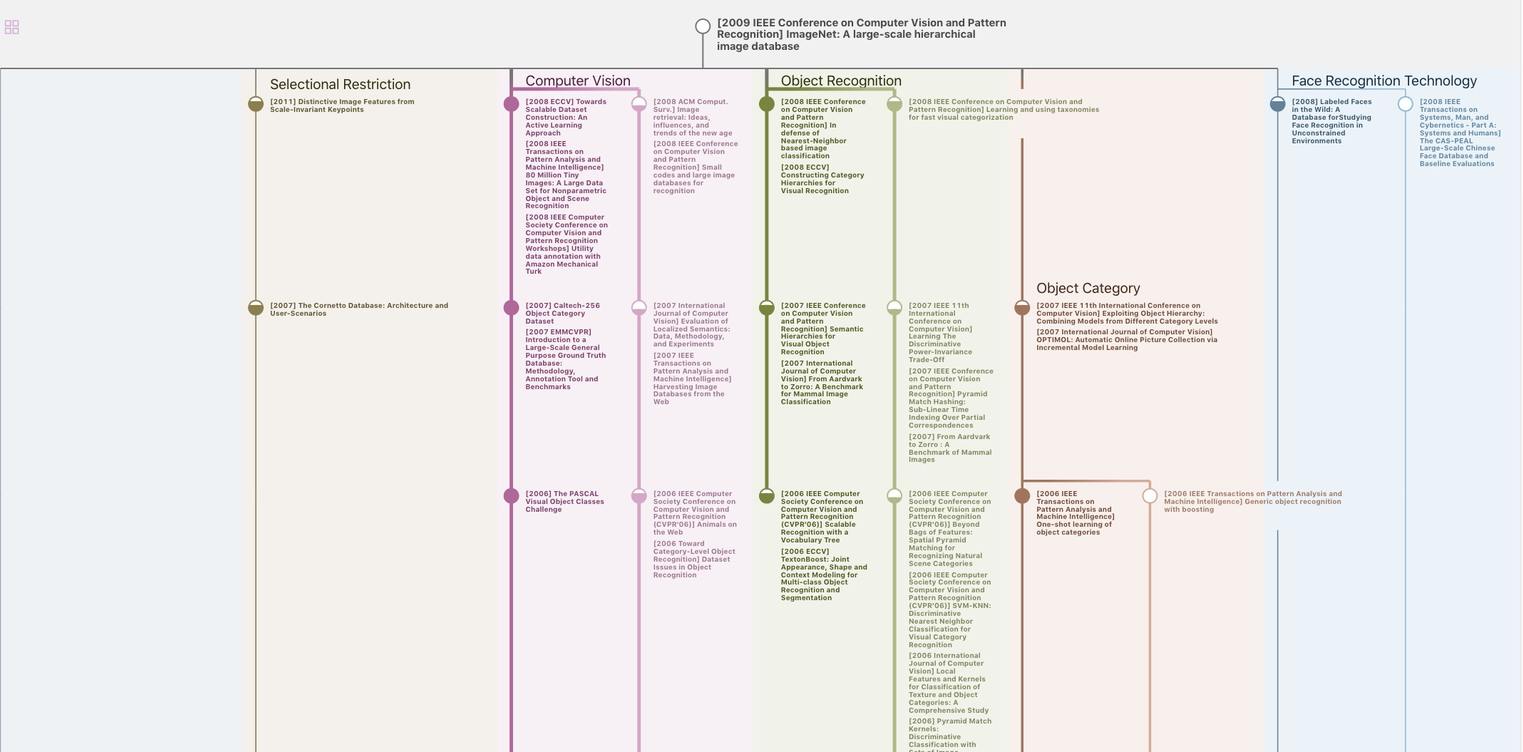
Generate MRT to find the research sequence of this paper
Chat Paper
Summary is being generated by the instructions you defined