Self-paced decentralized federated transfer framework for rotating machinery fault diagnosis with multiple domains
MECHANICAL SYSTEMS AND SIGNAL PROCESSING(2024)
Abstract
Leveraging distributed data from various clients to tackle target issues has become a prominent trend in fault diagnosis. However, the growing concerns about data privacy have gained significant attention in the research community. Addressing this, a self-paced decentralized federated transfer framework is developed for diagnosing faults in rotating machinery across diverse domains. To improve efficiency and enhance security in data privacy protection, a decentralized federated optimization strategy is formulated to address communication challenges across varied data domains. Initially, pre-trained source models extract self-supervised information from the target client data and utilize the self-paced mechanism to integrate this information into auxiliary models. At the same time, this paper employs a nonlinear hashing mapping scheme to encode features from the target client. Subsequently, contributions of different source models are assessed to determine their respective weights. The federated source models, along with auxiliary models, are then weighted appropriately to integrate the final target model. Finally, the obtained target model and encoded target features are transmitted back to the source clients for updates and feature alignment, with iterations continuing until convergence is reached. Thus, the proposed framework effectively addresses the gap in data distribution while ensuring data privacy protection. Comprehensive experiments validate the effectiveness and security of the proposed framework for fault diagnosis.
MoreTranslated text
Key words
Rotating machinery fault diagnosis,Data privacy,Decentralized federated learning,Multiple domains
AI Read Science
Must-Reading Tree
Example
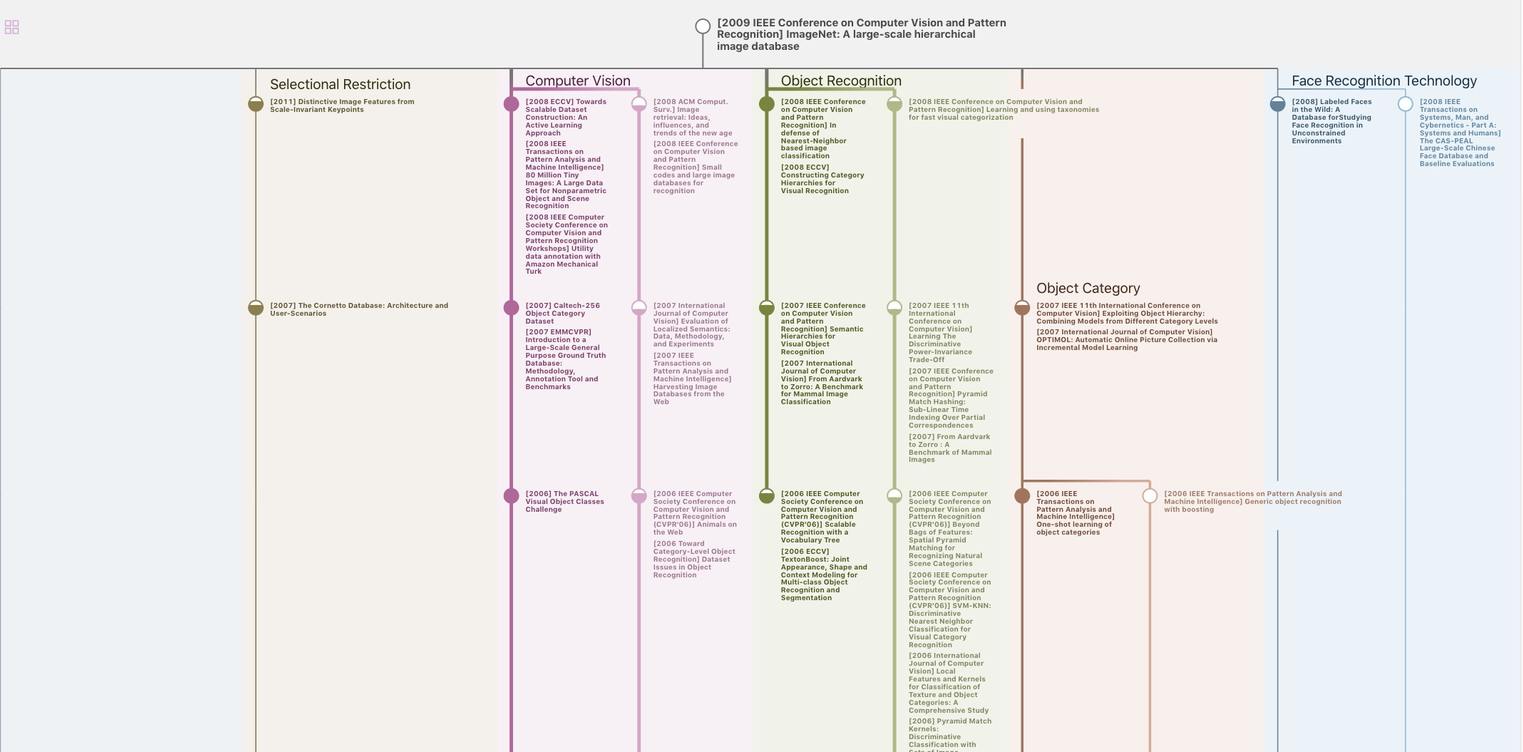
Generate MRT to find the research sequence of this paper
Chat Paper
Summary is being generated by the instructions you defined