Machine learning-derived identification of tumor-infiltrating immune cell-related signature for improving prognosis and immunotherapy responses in patients with skin cutaneous melanoma
Cancer cell international(2023)
摘要
Background Immunoblockade therapy based on the PD-1 checkpoint has greatly improved the survival rate of patients with skin cutaneous melanoma (SKCM). However, existing anti-PD-1 therapeutic efficacy prediction markers often exhibit a poor situation of poor reliability in identifying potential beneficiary patients in clinical applications, and an ideal biomarker for precision medicine is urgently needed. Methods 10 multicenter cohorts including 4 SKCM cohorts and 6 immunotherapy cohorts were selected. Through the analysis of WGCNA, survival analysis, consensus clustering, we screened 36 prognostic genes. Then, ten machine learning algorithms were used to construct a machine learning-derived immune signature (MLDIS). Finally, the independent data sets (GSE22153, GSE54467, GSE59455, and in-house cohort) were used as the verification set, and the ROC index standard was used to evaluate the model. Results Based on computing framework, we found that patients with high MLDIS had poor overall survival and has good prediction performance in all cohorts and in-house cohort. It is worth noting that MLDIS performs better in each data set than almost all models which from 51 prognostic signatures for SKCM. Meanwhile, high MLDIS have a positive prognostic impact on patients treated with anti-PD-1 immunotherapy by driving changes in the level of infiltration of immune cells in the tumor microenvironment. Additionally, patients suffering from SKCM with high MLDIS were more sensitive to immunotherapy. Conclusions Our study identified that MLDIS could provide new insights into the prognosis of SKCM and predict the immunotherapy response in patients with SKCM.
更多查看译文
关键词
Skin cutaneous melanoma,Machine learning,Tumor microenvironment,Immunotherapy
AI 理解论文
溯源树
样例
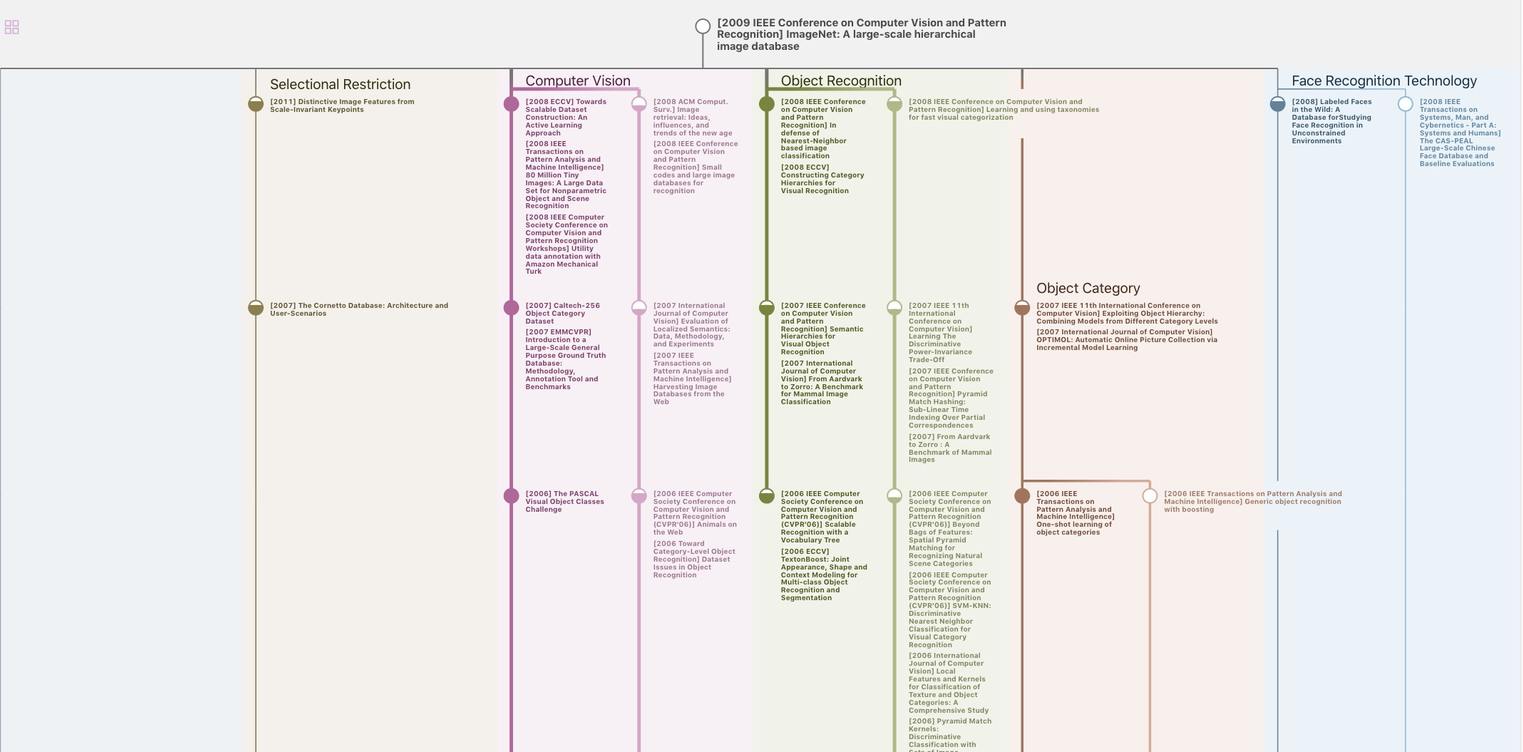
生成溯源树,研究论文发展脉络
Chat Paper
正在生成论文摘要