Specificity-aware Federated Learning with Dynamic Feature Fusion Network for Imbalanced Medical Image Classification.
IEEE journal of biomedical and health informatics(2023)
摘要
Recently, federated learning has become a powerful technique for medical image classification due to its ability to utilize datasets from multiple clinical clients while satisfying privacy constraints. However, there are still some obstacles in federated learning. Firstly, most existing methods directly average the model parameters collected by medical clients on the server, ignoring the specificities of the local models. Secondly, class imbalance is a common issue in medical datasets. In this paper, to handle these two challenges, we propose a novel specificity-aware federated learning framework that benefits from an Adaptive Aggregation Mechanism (AdapAM) and a Dynamic Feature Fusion Strategy (DFFS). Considering the specificity of each local model, we set the AdapAM on the server. The AdapAM utilizes reinforcement learning to adaptively weight and aggregate the parameters of local models based on their data distribution and performance feedback for obtaining the global model parameters. For the class imbalance in local datasets, we propose the DFFS to dynamically fuse the features of majority classes based on the imbalance ratio in the min-batch and collaborate the rest of features. We conduct extensive experiments on a dermoscopic dataset and a fundus image dataset. Experimental results show that our method can achieve state-of-the-art results in these two real-world medical applications.
更多查看译文
关键词
Federated learning,medical image classification,class imbalance,adaptive aggregation
AI 理解论文
溯源树
样例
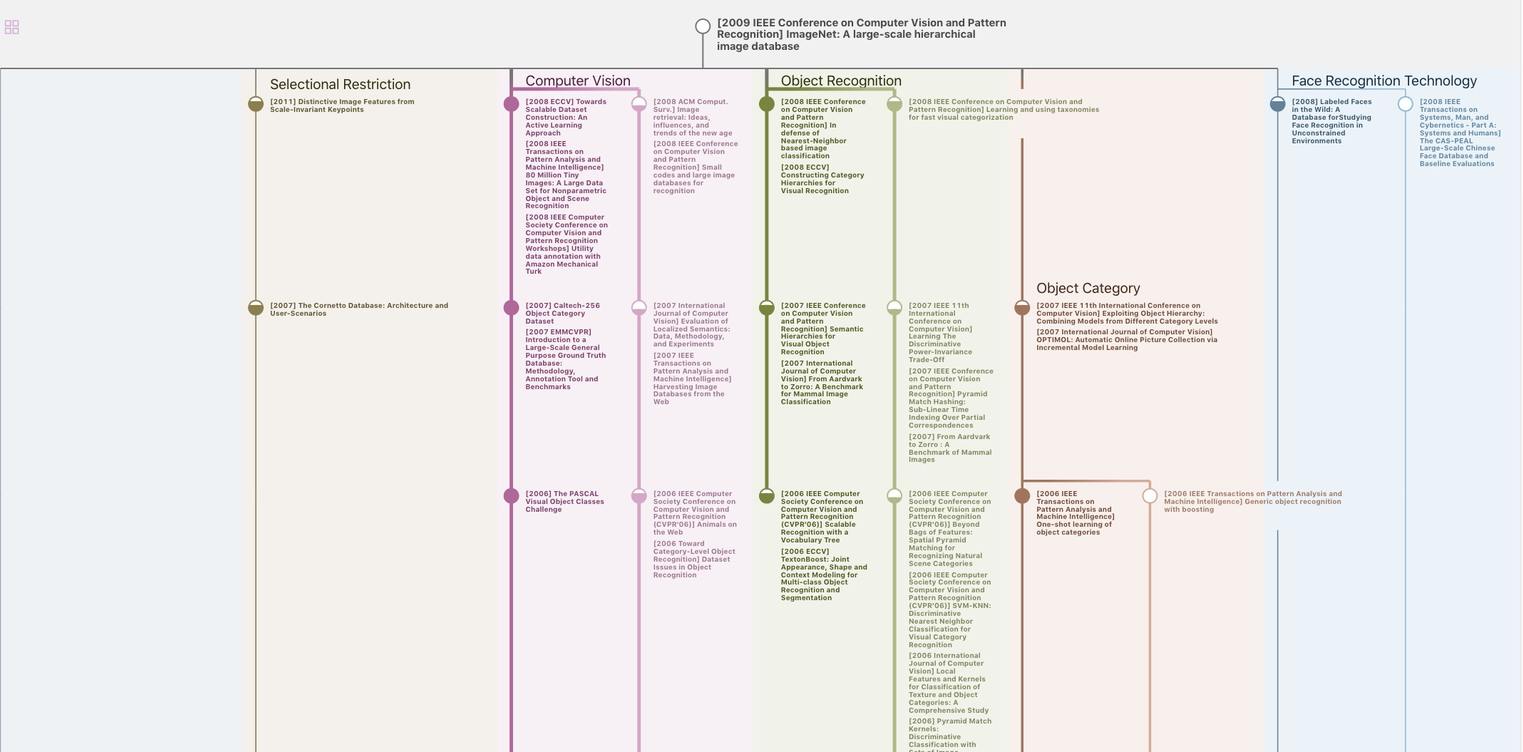
生成溯源树,研究论文发展脉络
Chat Paper
正在生成论文摘要