Differentiable Machine Learning-Based Modeling for Directly-Modulated Lasers
IEEE PHOTONICS TECHNOLOGY LETTERS(2024)
Abstract
End-to-end learning has become a popular method for joint transmitter and receiver optimization in optical communication systems. Such approach may require a differentiable channel model, thus hindering the optimization of links based on directly modulated lasers (DMLs). This is due to the DML behavior in the large-signal regime, for which no analytical solution is available. In this letter, this problem is addressed by developing and comparing differentiable machine learning-based surrogate models. The models are quantitatively assessed in terms of root mean square error and training/testing time. Once the models are trained, the surrogates are then tested in a numerical equalization setup, resembling a practical end-to-end scenario. Based on the numerical investigation conducted, the convolutional attention transformer is shown to outperform the other models considered.
MoreTranslated text
Key words
Optical transmitters,Optical communication,machine learning,directly modulated laser,transformer,modeling
AI Read Science
Must-Reading Tree
Example
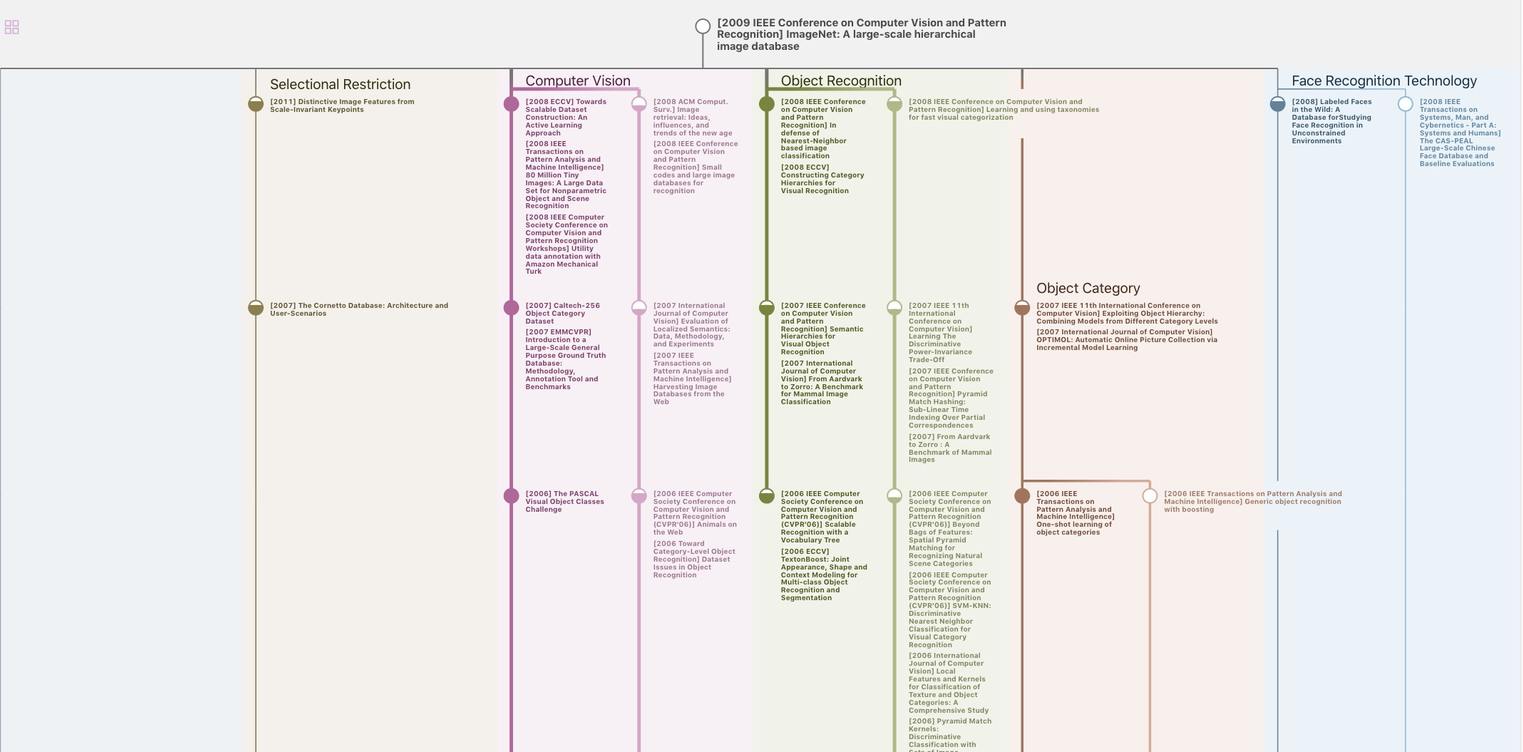
Generate MRT to find the research sequence of this paper
Chat Paper
Summary is being generated by the instructions you defined