Generative Speech Recognition Error Correction with Large Language Models and Task-Activating Prompting
CoRR(2023)
摘要
We explore the ability of large language models (LLMs) to act as speech
recognition post-processors that perform rescoring and error correction. Our
first focus is on instruction prompting to let LLMs perform these task without
fine-tuning, for which we evaluate different prompting schemes, both zero- and
few-shot in-context learning, and a novel task activation prompting method that
combines causal instructions and demonstration to increase its context windows.
Next, we show that rescoring only by in-context learning with frozen LLMs
achieves results that are competitive with rescoring by domain-tuned LMs, using
a pretrained first-pass recognition system and rescoring output on two
out-of-domain tasks (ATIS and WSJ). By combining prompting techniques with
fine-tuning we achieve error rates below the N-best oracle level, showcasing
the generalization power of the LLMs.
更多查看译文
关键词
large language model,N-best rescoring,instruction prompting,few-shot learning,in-context learning
AI 理解论文
溯源树
样例
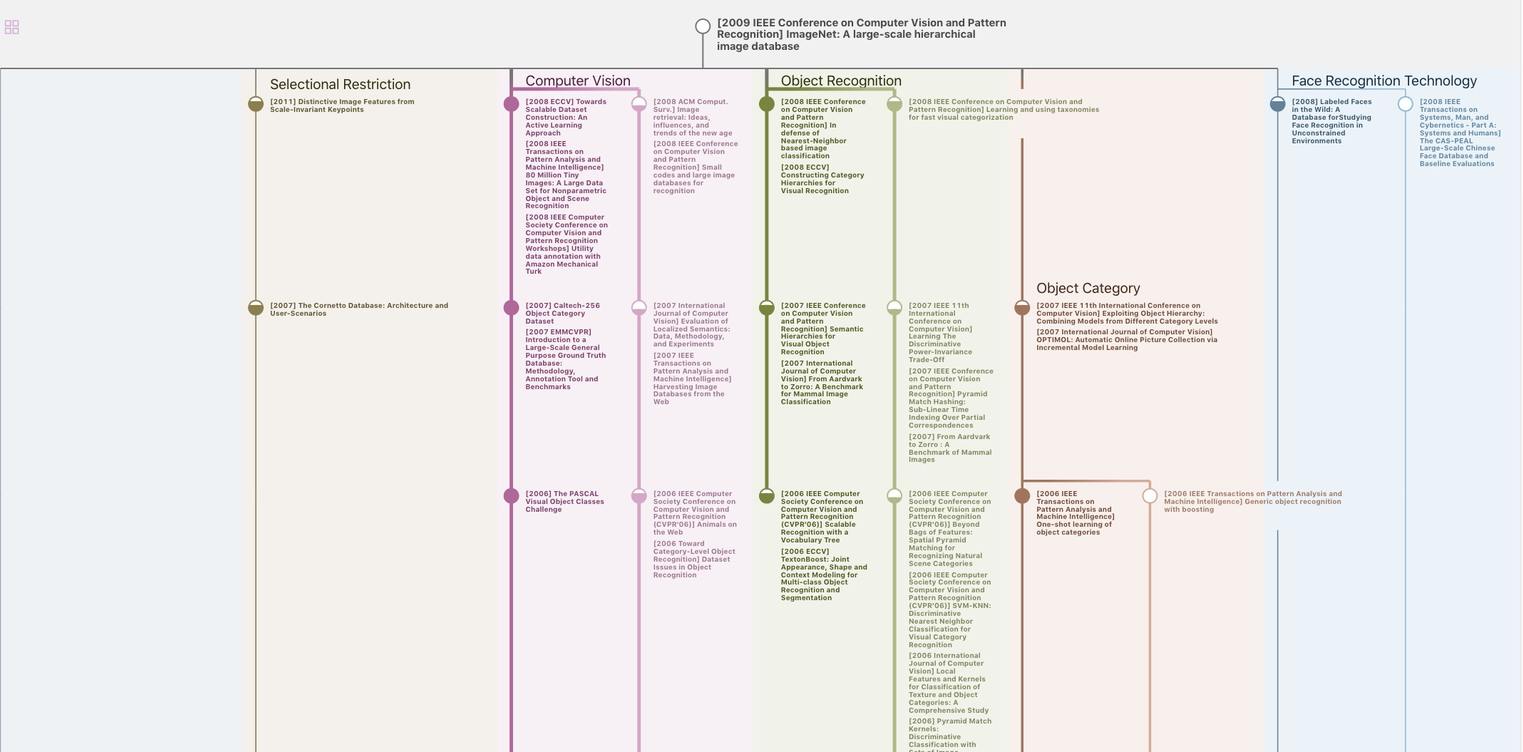
生成溯源树,研究论文发展脉络
Chat Paper
正在生成论文摘要