Learning from SAM: Harnessing a Foundation Model for Sim2Real Adaptation by Regularization
CoRR(2023)
摘要
Domain adaptation is especially important for robotics applications, where
target domain training data is usually scarce and annotations are costly to
obtain. We present a method for self-supervised domain adaptation for the
scenario where annotated source domain data (e.g. from synthetic generation) is
available, but the target domain data is completely unannotated. Our method
targets the semantic segmentation task and leverages a segmentation foundation
model (Segment Anything Model) to obtain segment information on unannotated
data. We take inspiration from recent advances in unsupervised local feature
learning and propose an invariance-variance loss over the detected segments for
regularizing feature representations in the target domain. Crucially, this loss
structure and network architecture can handle overlapping segments and
oversegmentation as produced by Segment Anything. We demonstrate the advantage
of our method on the challenging YCB-Video and HomebrewedDB datasets and show
that it outperforms prior work and, on YCB-Video, even a network trained with
real annotations. Additionally, we provide insight through model ablations and
show applicability to a custom robotic application.
更多查看译文
关键词
sim2real domain adaptation,regularization,segmentation foundation model,learning
AI 理解论文
溯源树
样例
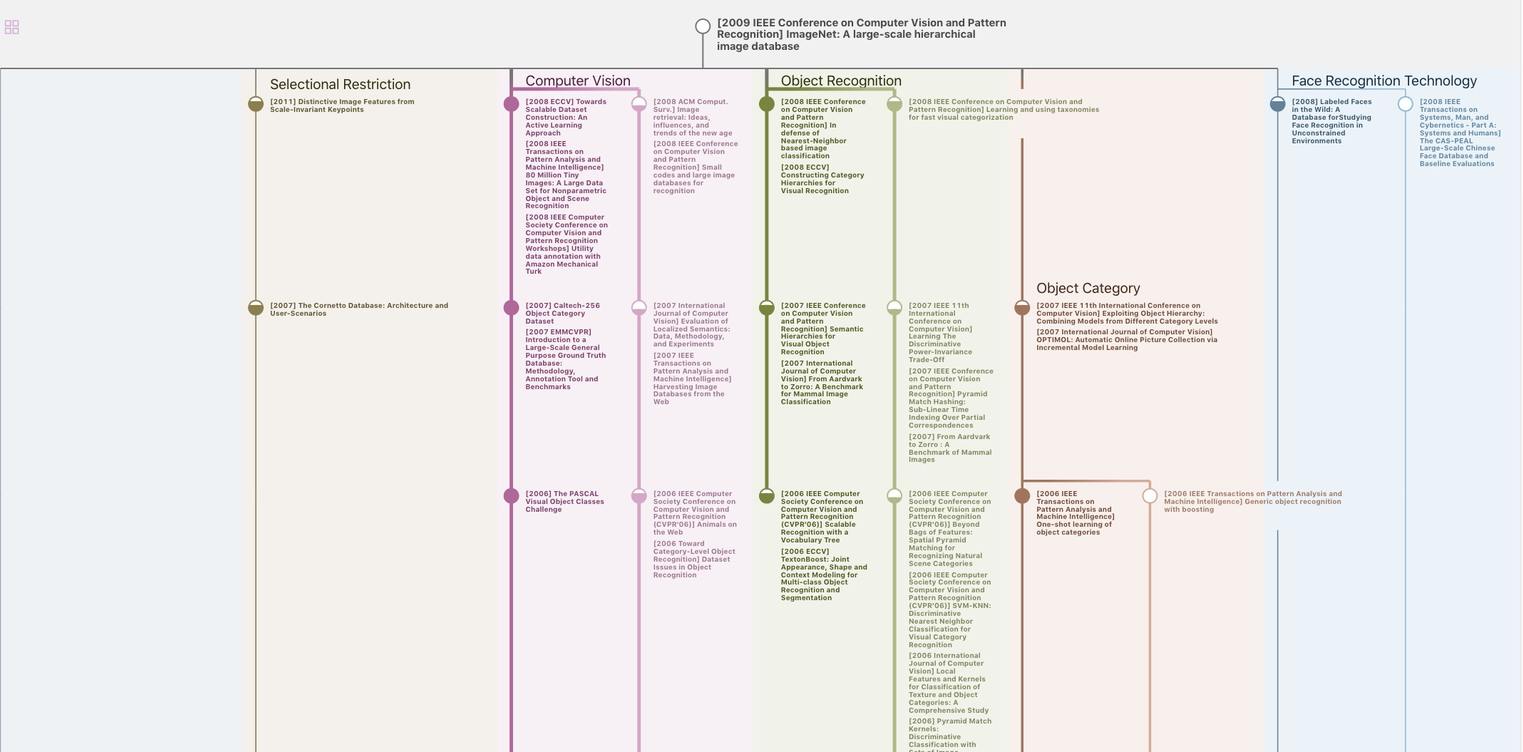
生成溯源树,研究论文发展脉络
Chat Paper
正在生成论文摘要