Towards Faithful Neural Network Intrinsic Interpretation with Shapley Additive Self-Attribution
CoRR(2023)
摘要
Self-interpreting neural networks have garnered significant interest in research. Existing works in this domain often (1) lack a solid theoretical foundation ensuring genuine interpretability or (2) compromise model expressiveness. In response, we formulate a generic Additive Self-Attribution (ASA) framework. Observing the absence of Shapley value in Additive Self-Attribution, we propose Shapley Additive Self-Attributing Neural Network (SASANet), with theoretical guarantees for the self-attribution value equal to the output's Shapley values. Specifically, SASANet uses a marginal contribution-based sequential schema and internal distillation-based training strategies to model meaningful outputs for any number of features, resulting in un-approximated meaningful value function. Our experimental results indicate SASANet surpasses existing self-attributing models in performance and rivals black-box models. Moreover, SASANet is shown more precise and efficient than post-hoc methods in interpreting its own predictions.
更多查看译文
关键词
neural network,shapley,self-attribution
AI 理解论文
溯源树
样例
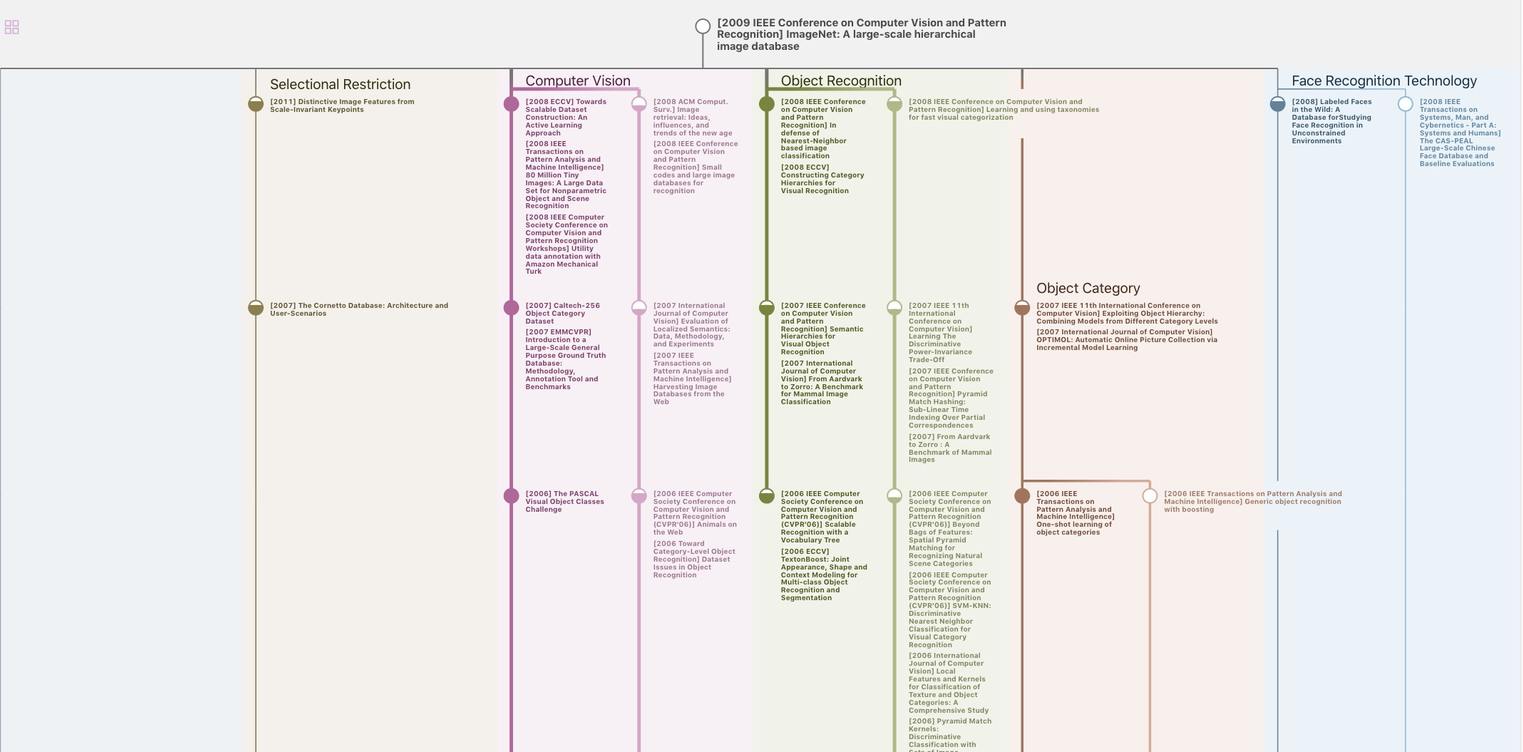
生成溯源树,研究论文发展脉络
Chat Paper
正在生成论文摘要