A Scalable Multi-Chiplet Deep Learning Accelerator with Hub-Side 2.5D Heterogeneous Integration
2023 IEEE Hot Chips 35 Symposium (HCS)(2023)
摘要
With the slowdown of Moore's law, the scenario diversity of specialized computing, and the rapid development of application algorithms, an efficient chip design requires modularization, flexibility, and scalability. In this study, we propose_ a Chiplet,-based deep, learning accelerator protoype that -contains oneHUB . Chipletand, six. extended SIDE- Chiplets integrated on an RDL layer for the 2.5D package. The SIDE and the HUB contain one and four AI cores, respectively. Given that our Chiplet-system targets diverse scenarios via scalable connected SIDE Chiplets, we need to handle three challenges: a) devise a flexible architecture design supporting diverse shapes, b) search for a workload mapping with low die-to-die communication, and c) adopt a high-bandwidth die-to-die interface to maintain efficient data transfer. This study proposes a flexible neural core (FNC) featuring dynamic bit-width computing and flexible parallelism. Next, we use a hierarchy-based mapping. scheme to decouple different parallelism levels and help analyze the communication. A 12Gbps,_D2D interface is introduced to achieve 192Gb/s bandwidth per D2D port with 1.04pJ/bit efficiency and 55um bump pitch. The proposed seven-Chiplet accelerator achieves a peak performance of 1 0/20/40 TOPS for INT16/8/4. When enabling 0~6 SIDE Chiplets, the system power ranges from 4.5W to 12W. The power efficiency of the FNC is 2.02TOPS/W while that of the overall system is 1.67TOPS/W.
更多查看译文
AI 理解论文
溯源树
样例
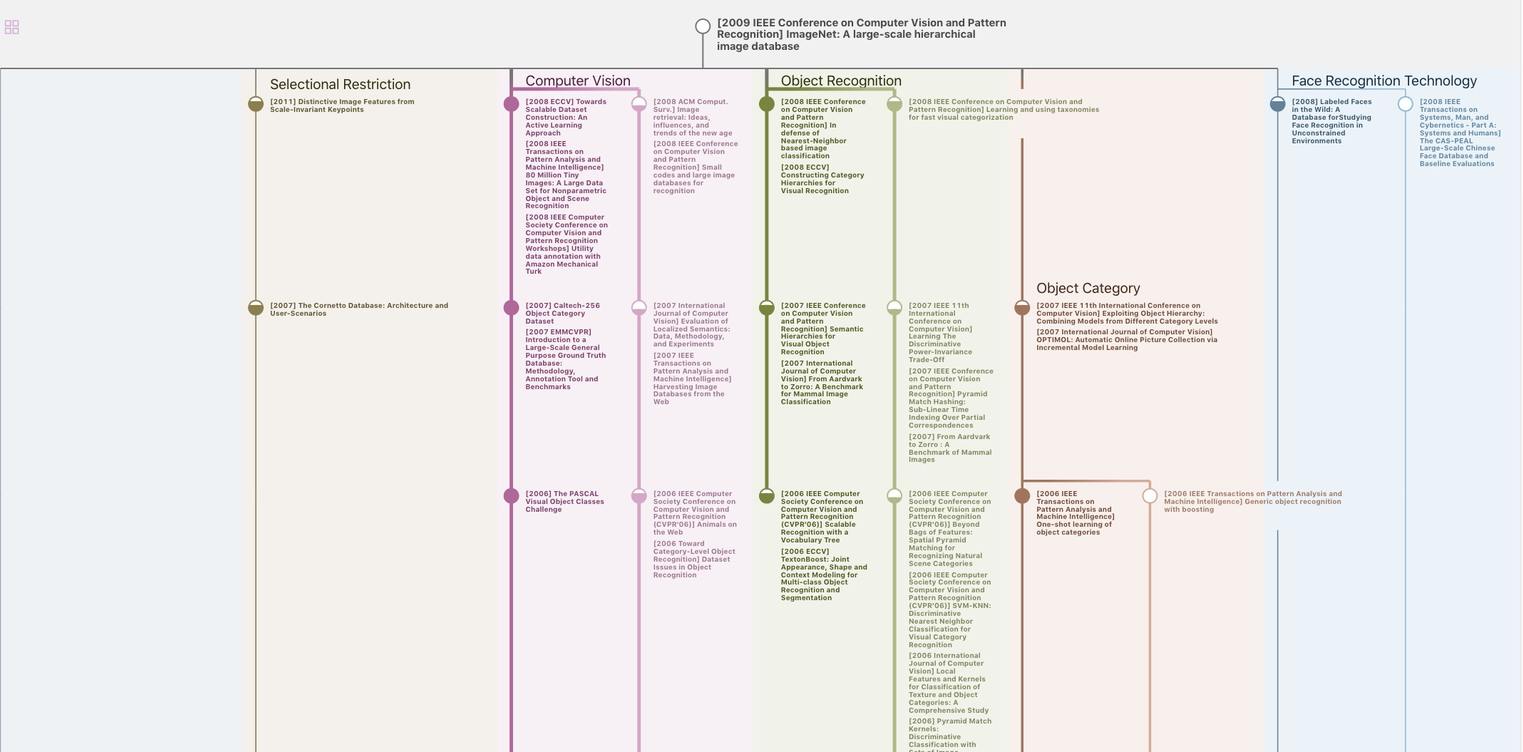
生成溯源树,研究论文发展脉络
Chat Paper
正在生成论文摘要