A Layered Semg-Fmg Hybrid Sensor for Hand Motion Recognition from Forearm Muscle Activities.
IEEE transactions on human-machine systems(2023)
摘要
The activities of muscles in the forearm have been widely investigated to develop human interfaces involving hand motions, especially in the fields of prosthetic hands and teleoperation. Although surface electromyography (sEMG) is considered as an effective biological signal from which hand motions can be recognized, the availability and quality of sEMG data can limit the usability and intuitiveness of human interfaces. This article introduces force myography (FMG) as a supplementary signal and proposes a layered sEMG–FMG hybrid sensor that can measure both sEMG and FMG at the same skin surface location. Meanwhile, a layer fusion convolution neural network (LFC) is designed to extract multiscale features from sEMG and FMG. To evaluate the effectiveness of the hybrid sEMG–FMG sensor and LFC, a 22-hand motion classification experiment was conducted on nine able-bodied subjects. The recognition results indicated a significantly improved classification accuracy (p < 0.001) of the hybrid sEMG–FMG modality with respect to single sEMG or FMG modality. The classification accuracies (CAs) of LFC were compared with conventional machine learning methods, including support vector machine, random forest classifier, xgboost, and k-nearest neighbor. Compared with the single-modality sEMG, the CAs of the dual-modality sEMG–FMG using conventional methods, and LFC were improved by 21.31% and 16.71%, respectively. These results suggest that the layered sEMG–FMG sensing approach can effectively enhance the performance of human interfaces, which offers great potential in the clinical applications of sophisticated prosthetic hands and teleoperation.
更多查看译文
关键词
Force myography (FMG),hybrid sensor,layer fusion convolutional neural network (LFC),surface electromyography (sEMG)
AI 理解论文
溯源树
样例
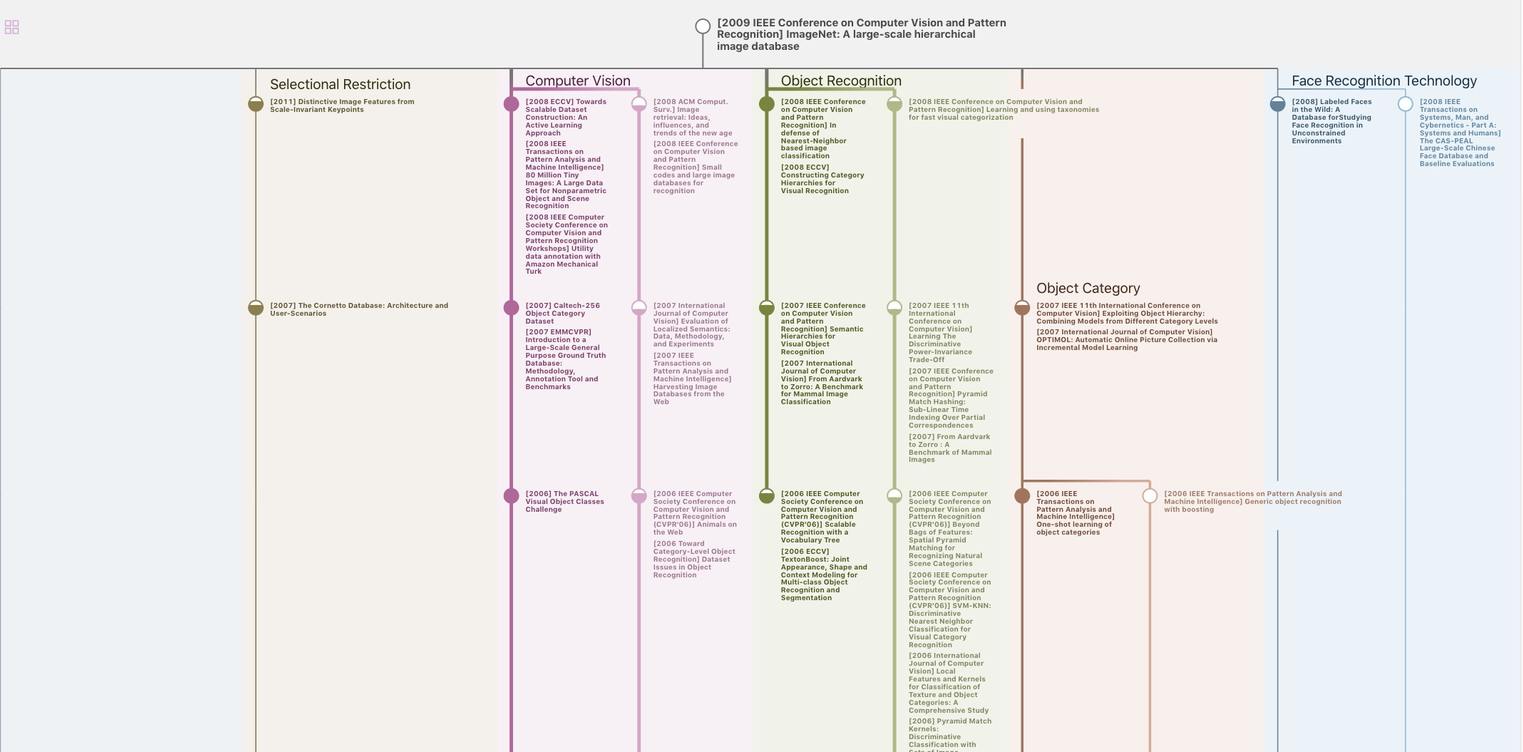
生成溯源树,研究论文发展脉络
Chat Paper
正在生成论文摘要