Automatic Genre Identification for Robust Enrichment of Massive Text Collections: Investigation of Classification Methods in the Era of Large Language Models
Machine Learning and Knowledge Extraction(2023)
摘要
Massive text collections are the backbone of large language models, the main ingredient of the current significant progress in artificial intelligence. However, as these collections are mostly collected using automatic methods, researchers have few insights into what types of texts they consist of. Automatic genre identification is a text classification task that enriches texts with genre labels, such as promotional and legal, providing meaningful insights into the composition of these large text collections. In this paper, we evaluate machine learning approaches for the genre identification task based on their generalizability across different datasets to assess which model is the most suitable for the downstream task of enriching large web corpora with genre information. We train and test multiple fine-tuned BERT-like Transformer-based models and show that merging different genre-annotated datasets yields superior results. Moreover, we explore the zero-shot capabilities of large GPT Transformer models in this task and discuss the advantages and disadvantages of the zero-shot approach. We also publish the best-performing fine-tuned model that enables automatic genre annotation in multiple languages. In addition, to promote further research in this area, we plan to share, upon request, a new benchmark for automatic genre annotation, ensuring the non-exposure of the latest large language models.
更多查看译文
关键词
machine learning, text classification, large language models, fine-tuning, automatic genre identification, text genre, web genre
AI 理解论文
溯源树
样例
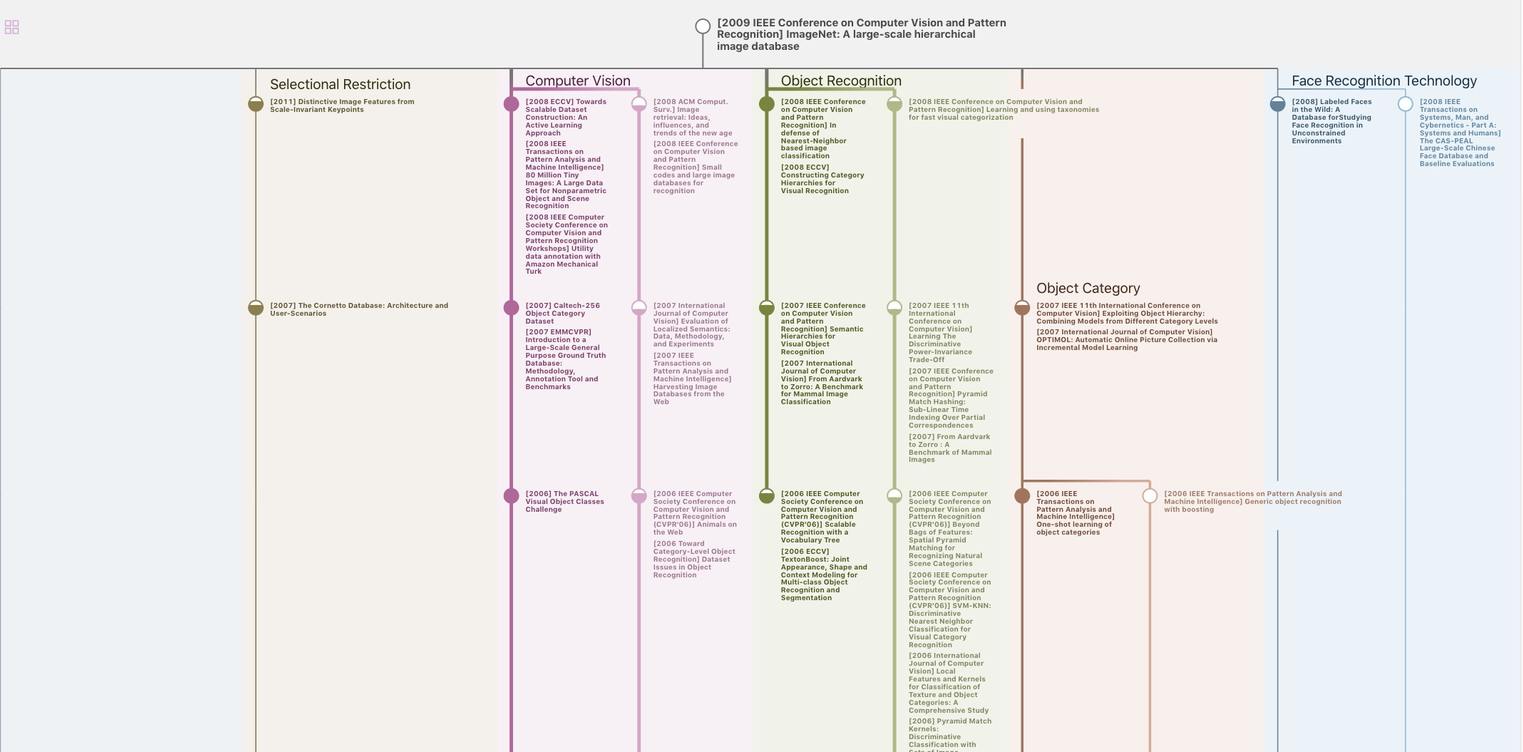
生成溯源树,研究论文发展脉络
Chat Paper
正在生成论文摘要