Prediction Model of Fouling Thickness of Heat Exchanger Based on TA-LSTM Structure
Processes(2023)
摘要
Heat exchangers in operation often experience scaling, which can lead to a decrease in heat exchange efficiency and even safety accidents when fouling accumulates to a certain thickness. To address this issue, manual intervention is currently employed to monitor fouling thickness in advance. In this study, we propose a two-layer LSTM neural network model with an attention mechanism to effectively learn fouling thickness data under different working conditions. The model accurately predicts the scaling thickness of the heat exchanger during operation, enabling timely human intervention and ensuring that the scaling remains within a safe range. The experimental results demonstrate that our proposed neural network model (TA-LSTM) outperforms both the traditional BP neural network model and the LSTM neural network model in terms of accuracy and stability. Our findings provide valuable technical support for future research on heat exchanger descaling and fouling growth detection.
更多查看译文
关键词
heat exchanger fouling,neural network,deep learning,attention mechanism,two-layer LSTM
AI 理解论文
溯源树
样例
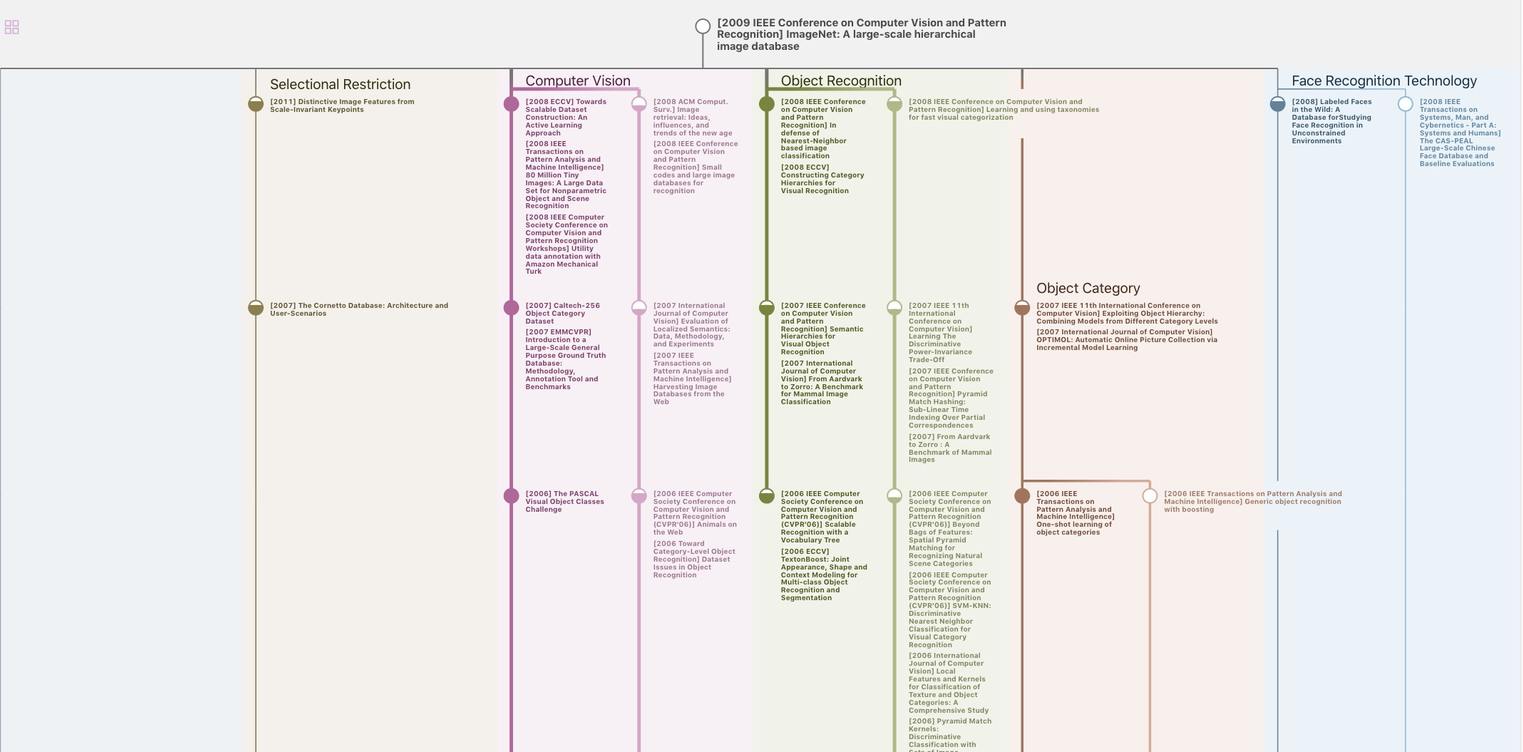
生成溯源树,研究论文发展脉络
Chat Paper
正在生成论文摘要