Analysis of Machine Learning Models for Wastewater Treatment Plant Sludge Output Prediction
SUSTAINABILITY(2023)
Abstract
With China's significant investment in wastewater treatment plants, urban sewage is effectively collected and treated, resulting in a substantial byproduct-sludge. As of 2021, a total of 2827 wastewater treatment plants have been constructed across 31 provinces, municipalities, and autonomous regions in China, with a processing capacity of 60.16 billion cubic meters. The production of dry sludge amounts to 14.229 million tons. The treatment and utilization of sludge pose significant challenges. The scientific calculation of sludge production is the basis for the reduction at the source and the design of sludge treatment and energy utilization. It is directly related to the construction scale, structure size, and equipment selection of the sludge treatment and utilization system and affects the operation and environmental management of the sludge treatment system. The wastewater treatment process using microbial metabolism is influenced by various known and unknown parameters, exhibiting highly nonlinear characteristics. These complex characteristics require the use of mathematical modeling for simulations and control. In this study, nine types of machine learning algorithms were used to establish sludge production prediction models. The extreme gradient boosting tree (XGBoost) and random forest models had the best prediction accuracies, with the former having RMSE, MAE, MAPE, and R-2 values of 4.4815, 2.1169, 1.7032, 0.0415, and 0.8218, respectively. These results suggested a superiority of ensemble learning models in fitting highly nonlinear data. In addition, the contribution and influence of various input features affecting sludge output were also studied for the XGBoost model, and the daily wastewater inflow volume and surrounding temperature features had the greatest impact on sludge production. The innovation of this study lies in the application of machine learning algorithms to achieve the prediction of sludge production in wastewater treatment plants.
MoreTranslated text
Key words
wastewater treatment plant,sludge,machine learning,prediction model
AI Read Science
Must-Reading Tree
Example
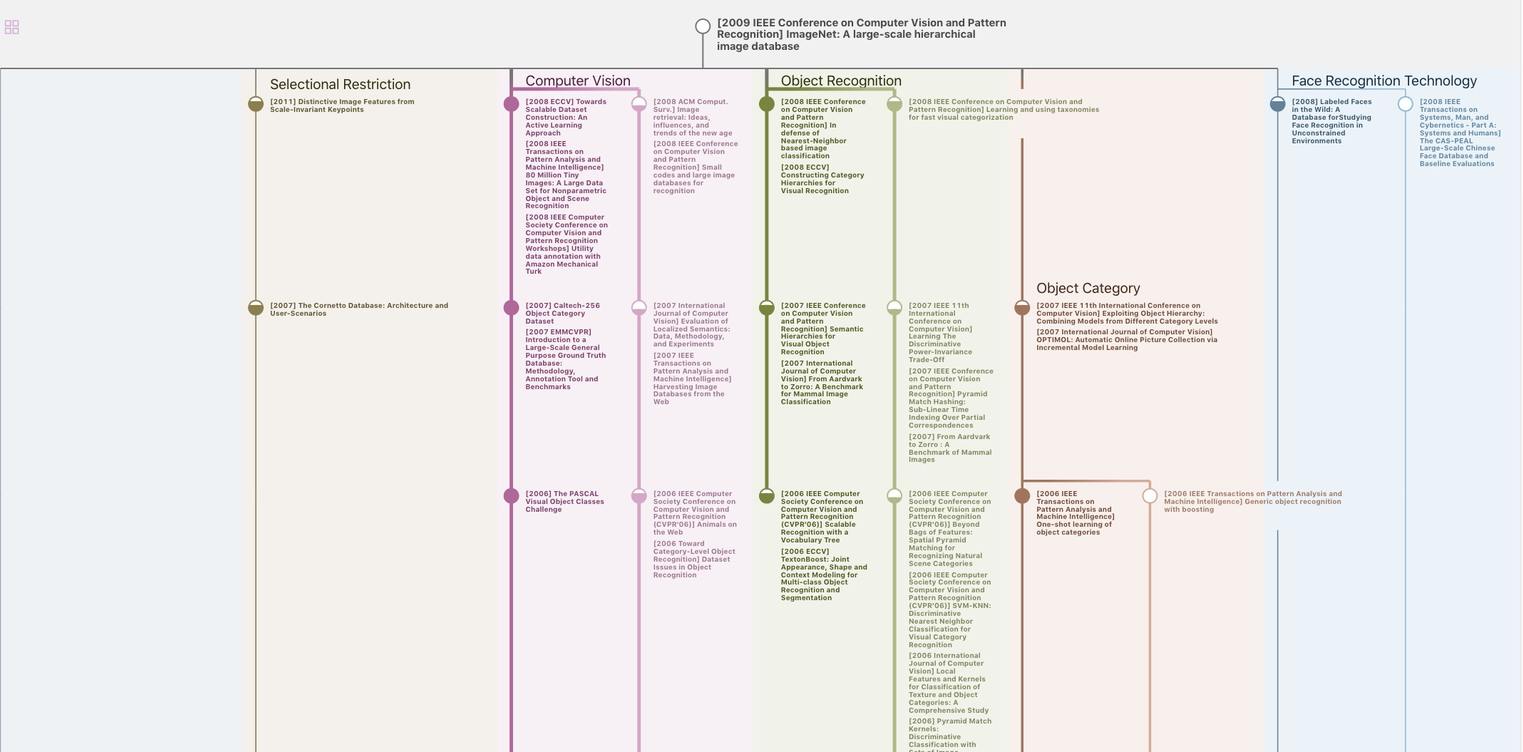
Generate MRT to find the research sequence of this paper
Chat Paper
Summary is being generated by the instructions you defined