A Bayesian approach to identifying the role of hospital structure and staff interactions in nosocomial transmission of SARS-CoV-2
medrxiv(2023)
Abstract
Nosocomial infections threaten patient safety, and were widely reported during the COVID-19 pandemic. Effective hospital infection control requires a detailed understanding of the role of different transmission pathways, yet these are poorly quantified. Using patient and staff data from a large UK hospital we demonstrate a method to infer unobserved epidemiological event times efficiently and disentangle the infectious pressure dynamics by ward. A stochastic individual-level, continuous-time state-transition model was constructed to model transmission of SARS-CoV-2, incorporating a dynamic staff-patient contact network as time-varying parameters. A Metropolis-Hastings MCMC algorithm was used to estimate transmission rate parameters associated with each possible source of infection, and the unobserved infection and recovery times. We found that the total infectious pressure exerted on an individual in a ward varied over time, as did the primary source of transmission. There was marked heterogeneity between wards; each ward experienced unique infectious pressure over time. Hospital infection control should consider the role of between-ward movement of staff as a key infectious source of nosocomial infection for SARS-CoV-2. With further development, this method could be implemented routinely for real-time monitoring of nosocomial transmission and to evaluate interventions.
### Competing Interest Statement
The authors have declared no competing interest.
### Funding Statement
JREB is supported by a Lancaster University Faculty of Health and Medicine doctoral scholarship. JMR and CPJ acknowledge support from UK Research and Innovation, through the JUNIPER modelling consortium grant MR/V038613/1 (JMR & CPJ), and through grant COV0357/MR/V028456/1 (JMR). CPJ acknowledges support from EPSRC grant EP/V042866/1.
### Author Declarations
I confirm all relevant ethical guidelines have been followed, and any necessary IRB and/or ethics committee approvals have been obtained.
Yes
The details of the IRB/oversight body that provided approval or exemption for the research described are given below:
Anonymised patient location, staff work patterns and SARS-CoV-2 testing results were extracted from routine trust electronic records. The study received HRA approval via IRAS and trust approval as a research project, accessing only routinely collected anonymised data (IRAS ID 288257).
I confirm that all necessary patient/participant consent has been obtained and the appropriate institutional forms have been archived, and that any patient/participant/sample identifiers included were not known to anyone (e.g., hospital staff, patients or participants themselves) outside the research group so cannot be used to identify individuals.
Yes
I understand that all clinical trials and any other prospective interventional studies must be registered with an ICMJE-approved registry, such as ClinicalTrials.gov. I confirm that any such study reported in the manuscript has been registered and the trial registration ID is provided (note: if posting a prospective study registered retrospectively, please provide a statement in the trial ID field explaining why the study was not registered in advance).
Yes
I have followed all appropriate research reporting guidelines, such as any relevant EQUATOR Network research reporting checklist(s) and other pertinent material, if applicable.
Yes
Full model code is available at [https://github.com/jbridgen/nosocomial\_covid\_model][1]. Anonymised patient and staff data are not publicly available due to restrictions placed on use of NHS routinely collected data and current HRA & IRAS approval. Any application to use original anonymised dataset will require HRA Confidentiality Advisory Group approval. Data access point of contact stacy.todd{at}liverpoolft.nhs.uk.
[https://github.com/jbridgen/nosocomial\_covid\_model][1]
[1]: https://github.com/jbridgen/nosocomial_covid_model
MoreTranslated text
AI Read Science
Must-Reading Tree
Example
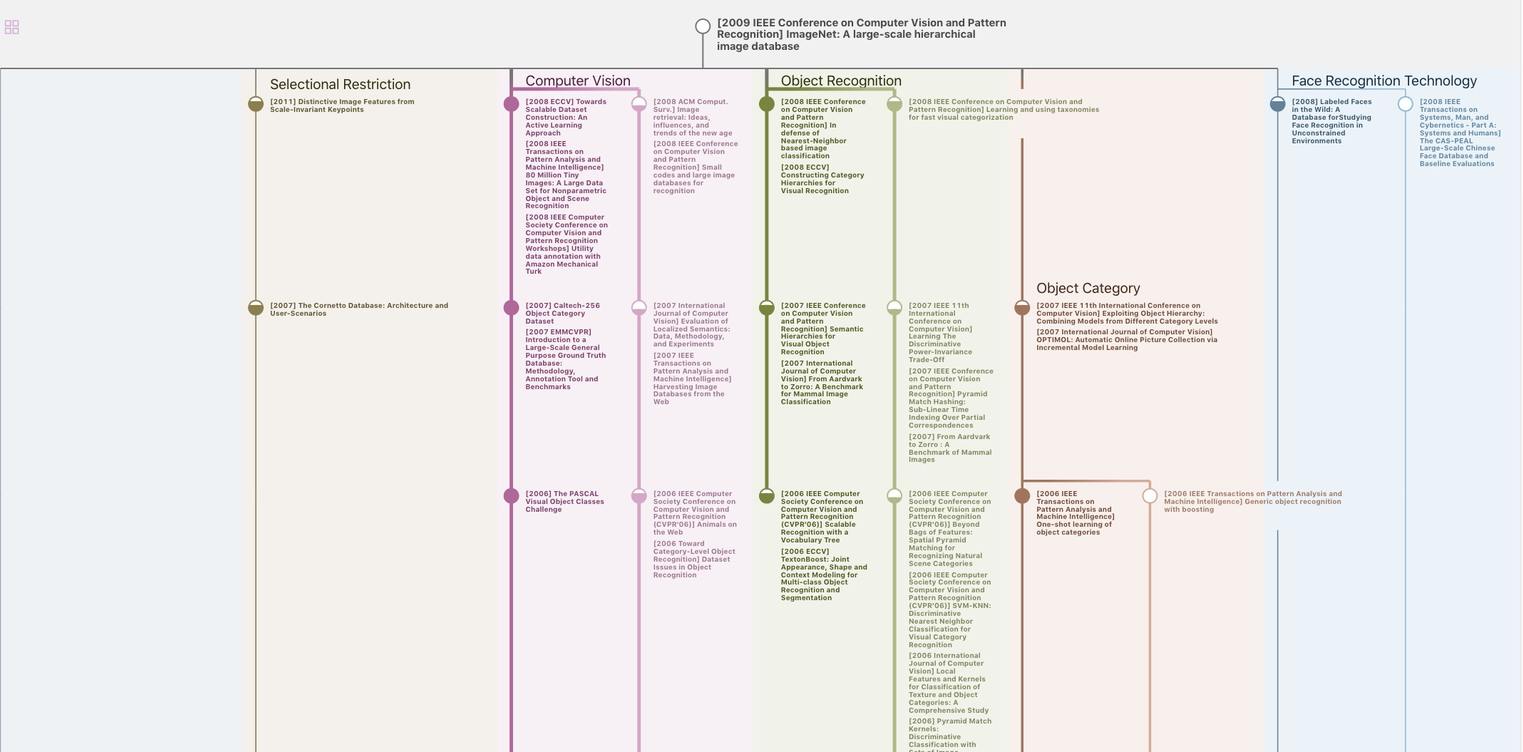
Generate MRT to find the research sequence of this paper
Chat Paper
Summary is being generated by the instructions you defined