Deep Learning based retinal layer segmentation in optical coherence tomography scans of patients with inherited retinal diseases
medrxiv(2023)
Abstract
Background On optical coherence tomography (OCT) scans of patients with inherited retinal diseases (IRDs), the outer nuclear layer (ONL) thickness measurement has been well established as a surrogate marker for photoreceptor preservation. Current automatic segmentation tools fail in OCT segmentation in IRDs, and manual segmentation is time consuming.
Methods and Material Patients with IRD and the availability of an OCT scan were screened for the present study. Additionally, OCT scans of patients without retinal disease were included, to provide training data for the artificial intelligence (AI). We trained a U-net based model on healthy patients and applied a domain adaption technique to IRD patients’ scans.
Results We established an AI-based image segmentation algorithm that reliably segments the ONL in OCT scans of IRD patients. In a test dataset, the dice-score of the algorithm was 98.7% . Furthermore, we generated thickness maps of the full retinal thickness and the ONL layer for each patient.
Conclusion Accurate segmentation of anatomical layers on OCT scans plays a crucial role for predictive models linking retinal structure to visual function. The here-presented OCT image segmentation algorithm could provide the basis for further studies on IRDs.
### Competing Interest Statement
The authors have declared no competing interest.
### Funding Statement
This study did not receive any funding
### Author Declarations
I confirm all relevant ethical guidelines have been followed, and any necessary IRB and/or ethics committee approvals have been obtained.
Yes
The details of the IRB/oversight body that provided approval or exemption for the research described are given below:
The Ethics Committee of the Medical Faculty at the Ludwig-Maximilians-Universitaet of Munich gave ethical approval for this work.
I confirm that all necessary patient/participant consent has been obtained and the appropriate institutional forms have been archived, and that any patient/participant/sample identifiers included were not known to anyone (e.g., hospital staff, patients or participants themselves) outside the research group so cannot be used to identify individuals.
Yes
I understand that all clinical trials and any other prospective interventional studies must be registered with an ICMJE-approved registry, such as ClinicalTrials.gov. I confirm that any such study reported in the manuscript has been registered and the trial registration ID is provided (note: if posting a prospective study registered retrospectively, please provide a statement in the trial ID field explaining why the study was not registered in advance).
Yes
I have followed all appropriate research reporting guidelines, such as any relevant EQUATOR Network research reporting checklist(s) and other pertinent material, if applicable.
Yes
All data produced in the present study are available upon reasonable request to the authors.
All data produced in the present study are available upon reasonable request to the authors.
MoreTranslated text
AI Read Science
Must-Reading Tree
Example
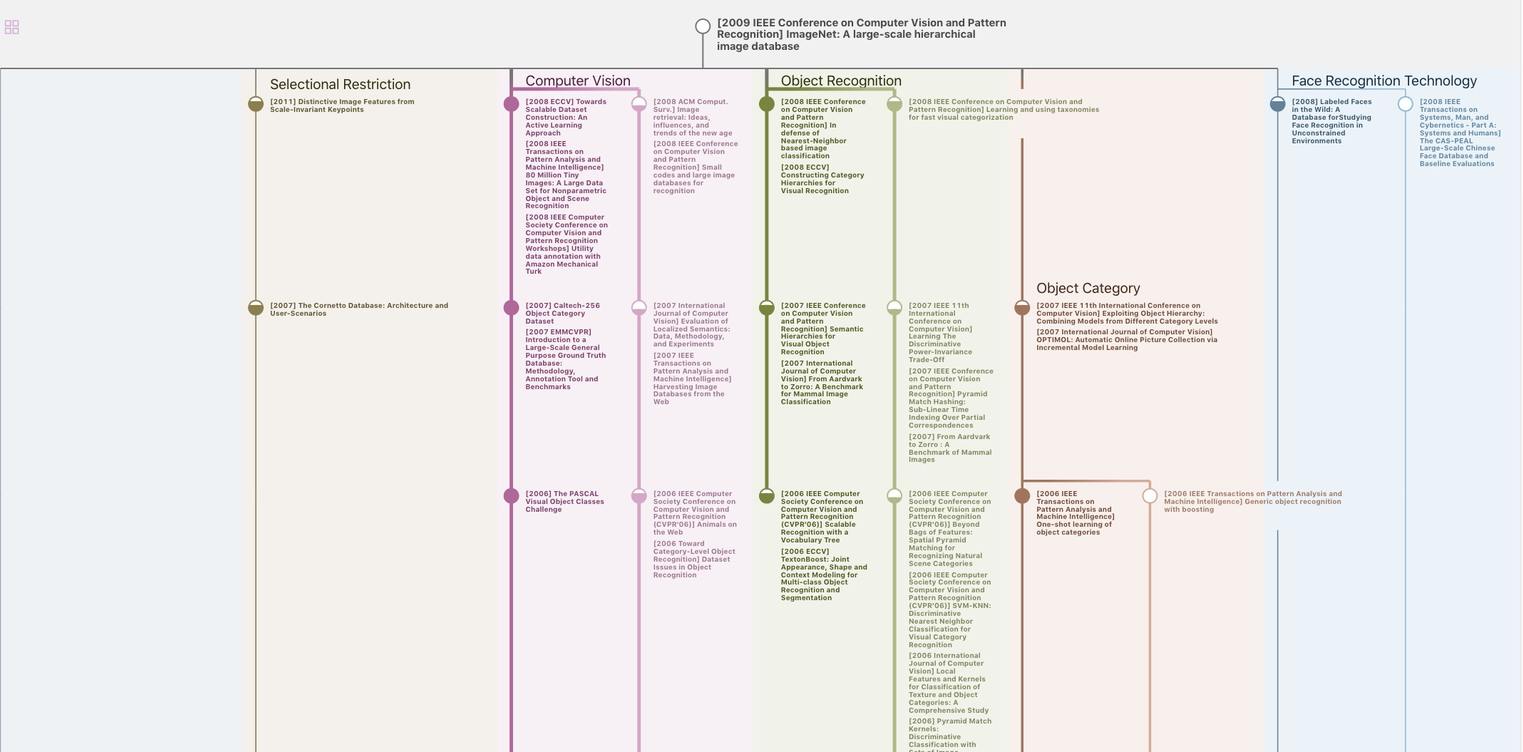
Generate MRT to find the research sequence of this paper
Chat Paper
Summary is being generated by the instructions you defined